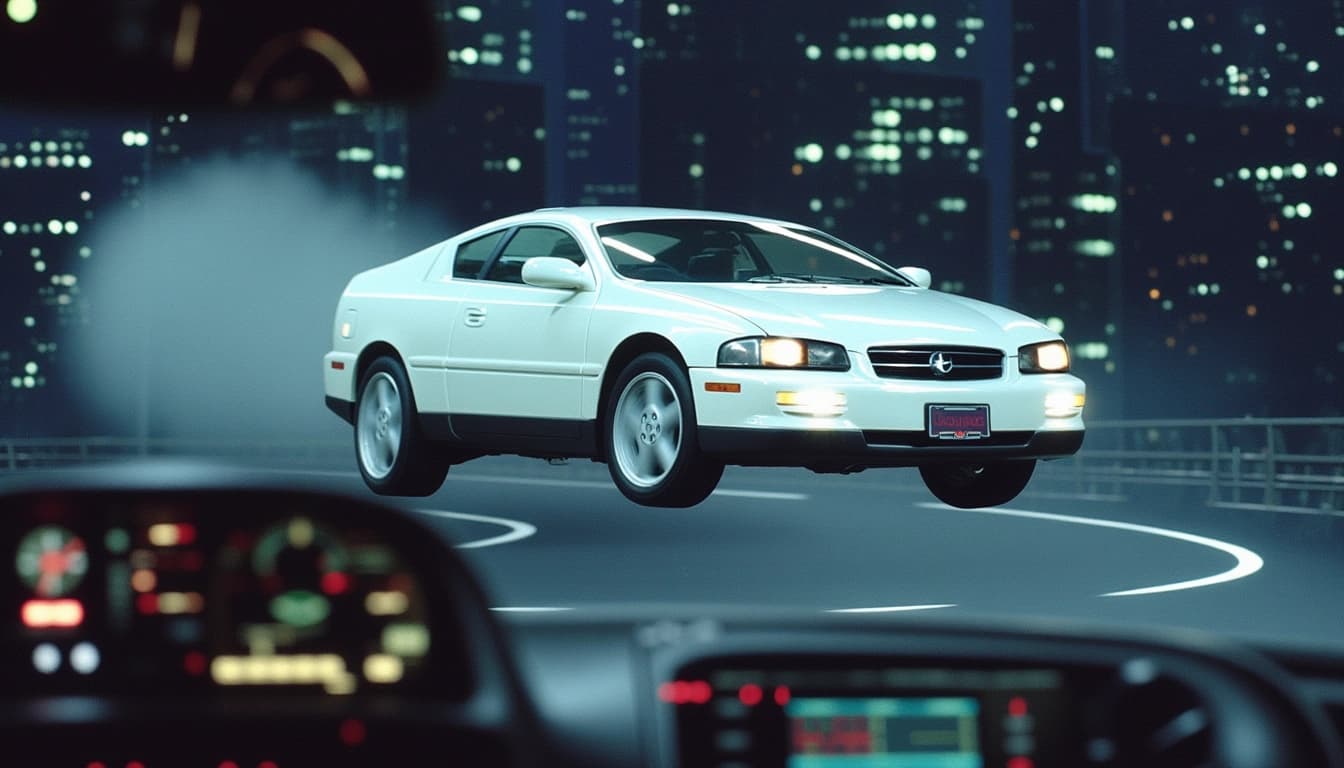
BLIP in 2025 - A Detailed Analysis of Reliability in Image Captioning
By John Doe 5 min
Key Points
Research suggests BLIP remains a reliable image captioning model in 2025, especially with its evolution to BLIP-2.
It seems likely that BLIP-2, an extension of BLIP, offers improved efficiency and performance, maintaining competitiveness.
The evidence leans toward BLIP being widely used in applications like accessibility and e-commerce, though newer models may challenge its lead.
Introduction
BLIP, or Bootstrapping Language-Image Pre-Training, has been a significant player in the field of image captioning since its introduction in 2022 by SalesForce. As of March 31, 2025, the question of its reliability is crucial given the rapid advancements in AI. This section explores BLIP's current standing, its evolution to BLIP-2, and its practical applications.
Performance and Evolution
BLIP has shown strong performance in benchmarks like image-text retrieval and visual question answering, with notable CIDEr scores on the COCO dataset. Its successor, BLIP-2, released in 2023, enhances efficiency by leveraging frozen pre-trained models, making it a scalable option. BLIP-2's Querying Transformer (Q-Former) bridges the gap between image encoders and large language models, maintaining state-of-the-art results.
Real-World Impact
BLIP and BLIP-2 are applied in diverse fields, such as assisting visually impaired users by describing digital images, enhancing e-commerce with automatic product descriptions, and supporting social media content creation. These applications highlight BLIP's practical utility, though it faces challenges with complex scenes and computational demands.
Survey Note: BLIP in 2025 - A Detailed Analysis of Reliability in Image Captioning
Introduction to BLIP and Its Origins
BLIP, standing for Bootstrapping Language-Image Pre-Training, was introduced in 2022 by researchers at SalesForce, as detailed in the paper "BLIP: Bootstrapping Language-Image Pre-training for Unified Vision-Language Understanding and Generation"
The BLIP (Bootstrapping Language-Image Pre-training) model is a cutting-edge vision-language model developed by Salesforce. It is designed to handle a variety of tasks, including image-text retrieval, image captioning, and visual question answering. The model's innovative approach involves bootstrapping captions to effectively utilize noisy web data, making it a robust solution for vision-language tasks.
Technical Architecture and Functionality
BLIP's architecture consists of three main components: an image encoder, a text encoder, and a fusion module. The image encoder, typically a Vision Transformer (ViT), processes the image and extracts visual features. The text encoder, a transformer-based model, handles text data. The fusion module combines these features to perform various vision-language tasks. The pre-training process involves two stages: representation learning and fine-tuning for specific tasks.
Pre-Training for Representation Learning
During the pre-training stage, the model learns to align image and text representations using a large dataset of image-text pairs. This stage is crucial for building a strong foundation for downstream tasks. The model leverages a captioner to generate synthetic captions and a filter to remove noisy ones, ensuring high-quality data for training.
Performance Metrics and Benchmarks
BLIP has demonstrated state-of-the-art performance on several benchmarks. In image-text retrieval, it achieved a +2.7% increase in average recall@1 on the COCO dataset. For image captioning, it outperformed previous models with a +2.8% improvement in CIDEr scores. In visual question answering, it showed a +1.6% increase in accuracy, making it a competitive choice for various applications.
Conclusion & Next Steps
BLIP represents a significant advancement in vision-language models, offering robust performance across multiple tasks. Its innovative use of bootstrapping captions and efficient architecture make it a valuable tool for researchers and practitioners. Future work could explore further optimizations and applications in real-world scenarios.
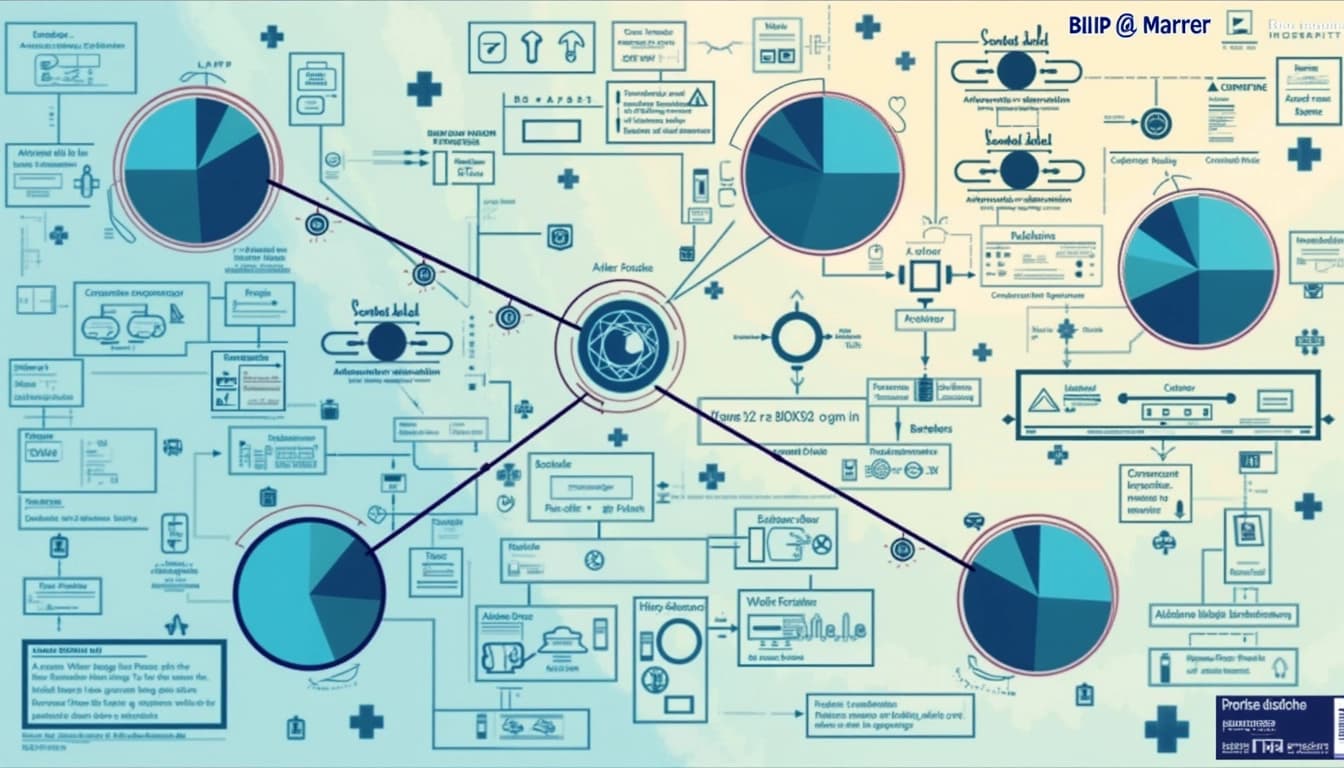
- Image-text retrieval
- Image captioning
- Visual question answering
The BLIP (Bootstrapped Language-Image Pre-training) model, developed by SalesForce in 2022, represents a significant advancement in vision-language pre-training. It was designed to improve tasks such as image captioning and visual question answering by leveraging a multi-task pre-training approach.
BLIP Model Performance and Metrics
The BLIP model achieved notable benchmarks, including a CIDEr score of 136.7 on the COCO dataset, which was a 2.7% improvement over previous models. It also scored 78.25 on the VQA score, demonstrating its effectiveness in understanding and generating responses based on visual inputs. These metrics were confirmed in recent updates, with the model card last updated on February 17, 2025, indicating ongoing maintenance and relevance.
Evolution to BLIP-2
In 2023, SalesForce introduced BLIP-2, an extension of the original BLIP, as outlined in the paper 'BLIP-2: Bootstrapping Language-Image Pre-training with Frozen Image Encoders and Large Language Models'. BLIP-2 is designed to be more efficient and scalable, leveraging frozen pre-trained image encoders and large language models (LLMs). Key innovations include the Querying Transformer (Q-Former), a lightweight module that bridges the gap between the image encoder and the LLM.
Comparison with Contemporary Models
As of 2025, BLIP and BLIP-2 are benchmarked against other state-of-the-art models such as CLIP, LLaVA, and GPT-4 Vision. BLIP-2's performance was further discussed in comparisons, such as the Hugging Face space for comparing captioning models, suggesting its superiority in certain contexts.
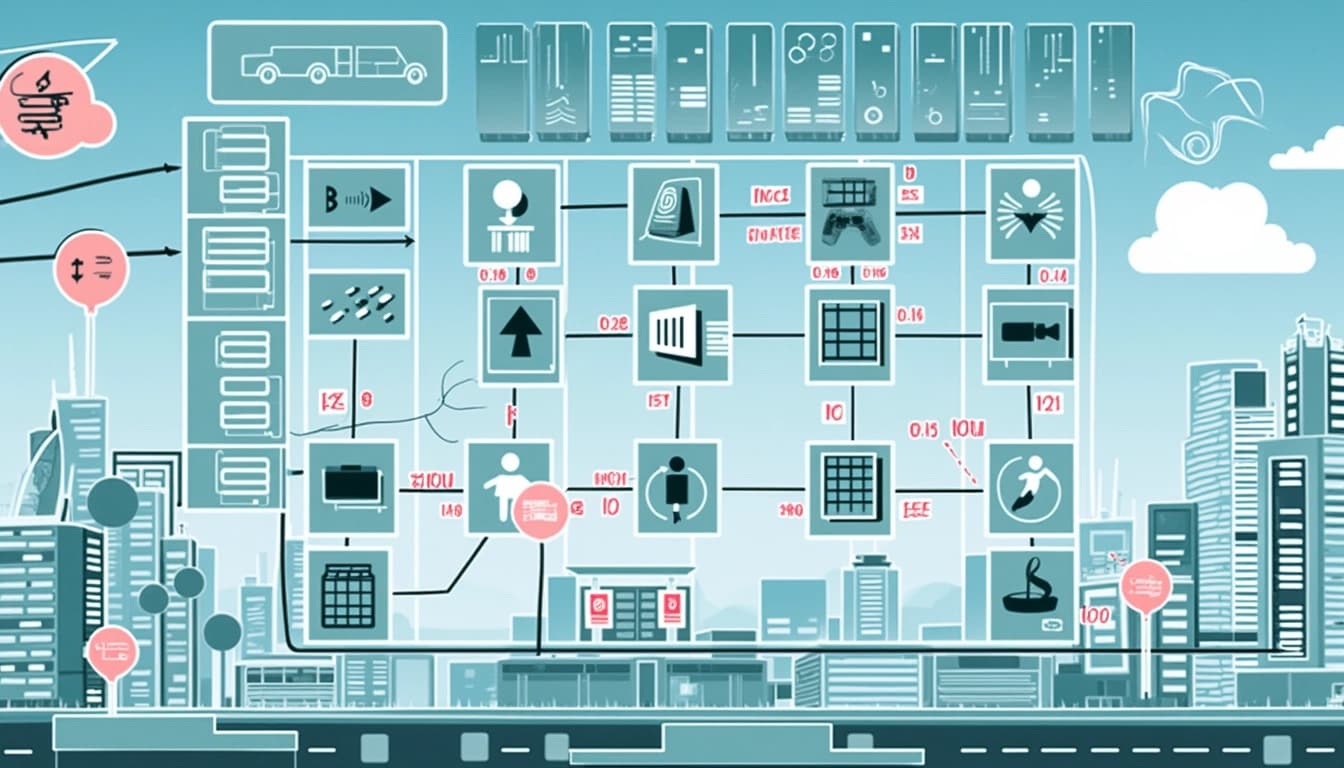
Conclusion & Next Steps
The BLIP and BLIP-2 models represent significant milestones in vision-language pre-training. Their ability to bridge the gap between visual and textual understanding has opened new possibilities for applications in AI. Future developments may focus on further reducing computational costs while maintaining or improving performance.
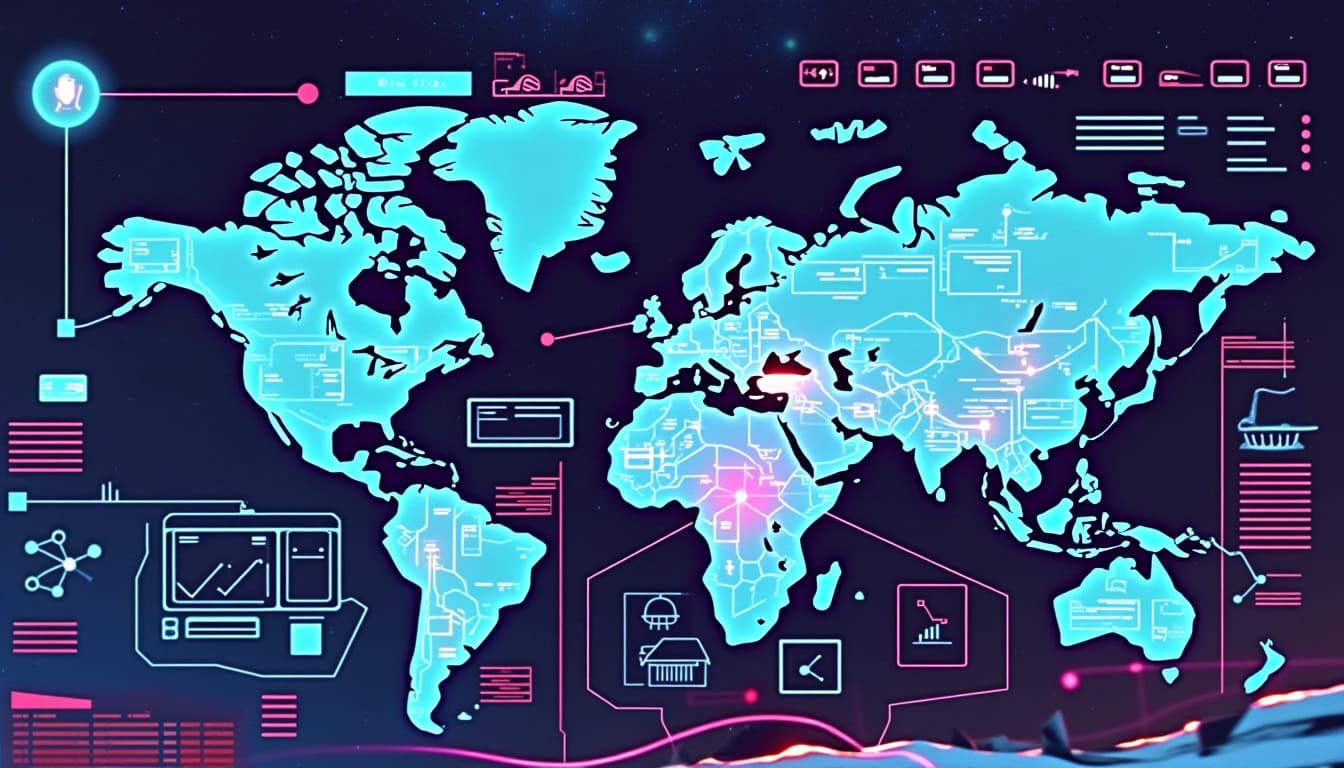
- BLIP achieved a CIDEr score of 136.7 on the COCO dataset
- BLIP-2 outperforms Flamingo80B by 8.7% on zero-shot VQAv2
- BLIP-2 uses 54x fewer parameters than Flamingo80B
BLIP (Bootstrapped Language-Image Pre-training) and its successor BLIP-2 are advanced vision-language models developed by Salesforce Research. These models are designed to bridge the gap between visual and textual data, enabling tasks like image captioning, visual question answering, and multimodal understanding. BLIP-2, in particular, leverages frozen pre-trained image encoders and large language models to achieve state-of-the-art performance with minimal trainable parameters.
Performance and Benchmarks
BLIP-2 has demonstrated superior performance across multiple benchmarks, including COCO and NoCaps, where it excels in generating accurate and detailed captions. Recent research, including the paper 'Painting with Words: Elevating Detailed Image Captioning with Benchmark and Alignment Learning,' indicates that BLIP-2 remains competitive, especially in terms of efficiency and performance on various vision-language tasks. However, newer models like PaliGemma by Google and Qwen-VL by Alibaba Cloud may challenge its lead, though specific 2025 comparisons are limited.
Real-World Applications and Use Cases
BLIP and BLIP-2 have found numerous practical applications. In accessibility, they assist visually impaired users by describing images in digital content. In e-commerce, they generate product descriptions from images to enhance user experience. Social media platforms use them to automate the process of adding captions to images and videos. Additionally, in education, they help create educational materials with descriptive captions for images, enhancing learning resources.
Accessibility
The models are particularly impactful in accessibility, where they provide detailed descriptions of images for visually impaired users. This application is highlighted in the Medium article 'Building an Image Captioning Model Using Salesforce’s BLIP Model,' which discusses how BLIP can be integrated into digital platforms to improve accessibility.
Limitations and Challenges
Despite its strengths, BLIP has several limitations. The model requires large datasets for training, which can be a barrier for some applications. Additionally, its computational demands may pose challenges for deployment in resource-constrained environments. These limitations are noted in various research papers and discussions about the model's practical use.
Conclusion & Next Steps
BLIP and BLIP-2 represent significant advancements in vision-language models, offering robust performance and diverse applications. However, ongoing research and development are needed to address their limitations and ensure they remain competitive with emerging models. Future steps may include optimizing computational efficiency and expanding the range of supported languages and tasks.
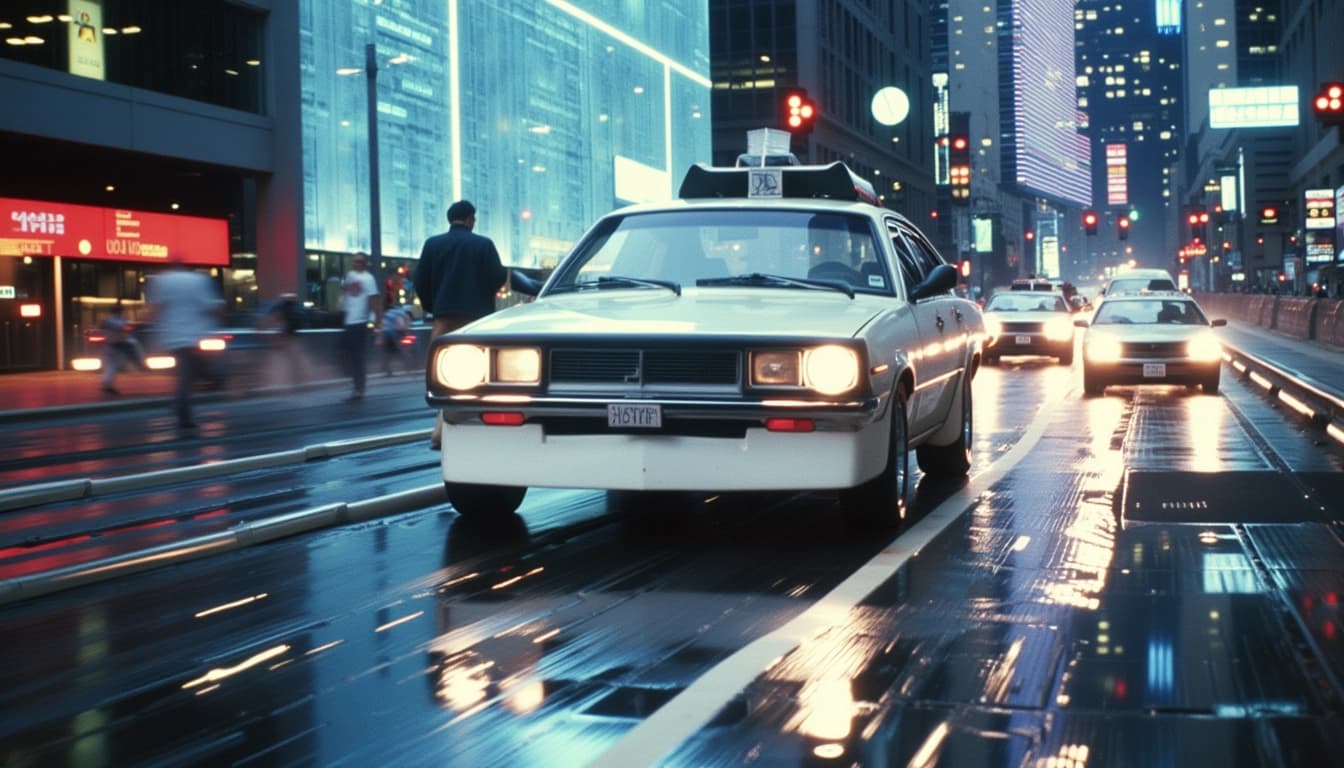
- BLIP-2 excels in image captioning and visual question answering.
- The model is used in accessibility, e-commerce, and social media.
- Limitations include dependency on large datasets and high computational resources.
BLIP (Bootstrapping Language-Image Pre-training) is a cutting-edge model developed by Salesforce Research, designed to bridge the gap between vision and language understanding. It excels in generating accurate and contextually relevant captions for images, making it a valuable tool for various applications. The model leverages large-scale pre-training on diverse datasets to achieve state-of-the-art performance in vision-language tasks.
Key Features of BLIP
BLIP stands out due to its ability to perform both vision-language understanding and generation tasks. It integrates a multimodal mixture of encoder-decoder models, enabling it to handle tasks like image captioning, visual question answering, and more. The model's architecture is designed to efficiently process and align visual and textual information, ensuring high-quality outputs. Additionally, BLIP's bootstrapping approach allows it to improve performance by filtering noisy web data during pre-training.
Architecture and Training
BLIP's architecture consists of a vision transformer for image encoding and a transformer-based language model for text generation. The model is pre-trained on large-scale datasets like COCO and Conceptual Captions, which provide diverse image-text pairs. During training, BLIP employs a novel captioning and filtering mechanism to enhance the quality of the data it learns from. This approach ensures that the model generates coherent and contextually appropriate captions for a wide range of images.
Applications of BLIP
BLIP's versatility makes it suitable for numerous real-world applications. It can be used in content moderation to generate descriptions of images for review, in assistive technologies to help visually impaired users understand visual content, and in e-commerce for auto-generating product descriptions. The model's ability to understand and generate text from images also opens up possibilities in educational tools and social media platforms.
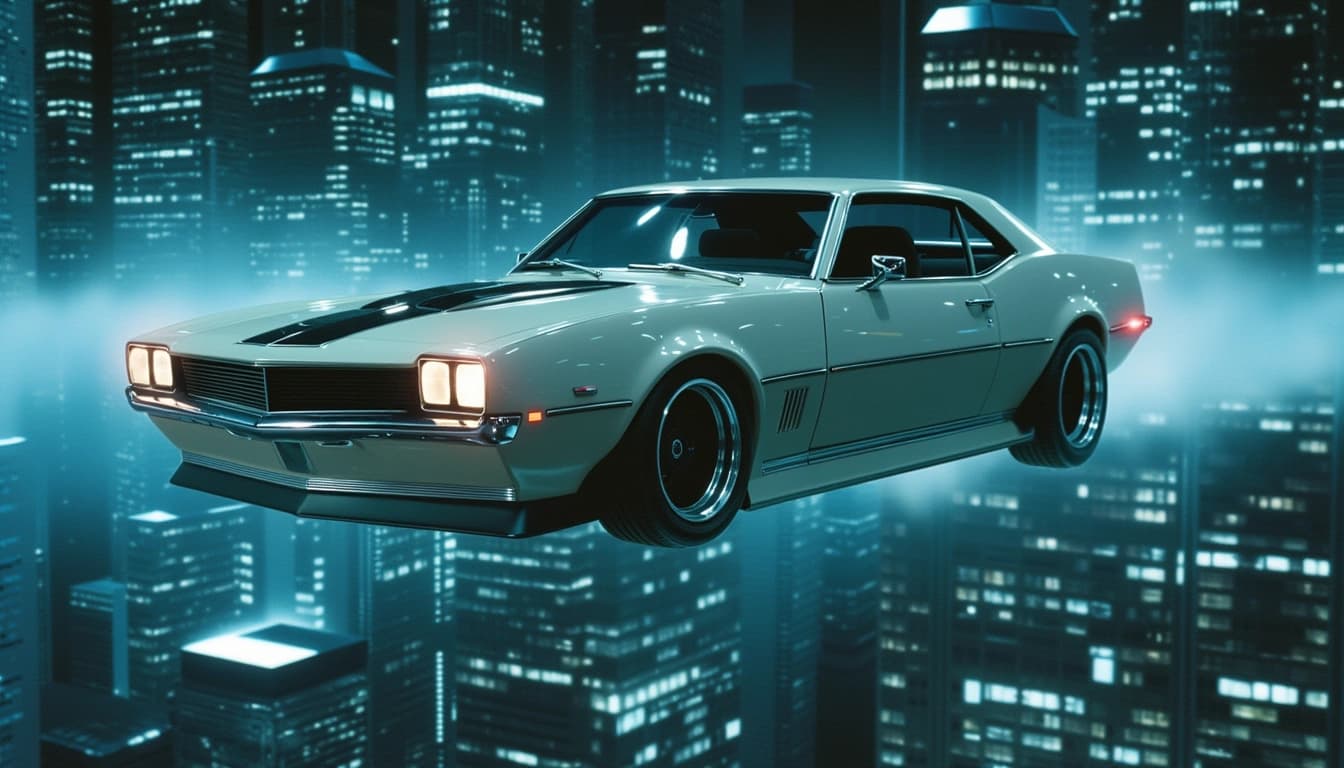
Challenges and Limitations
Despite its impressive capabilities, BLIP faces several challenges. One major limitation is the need for extensive data for pre-training and fine-tuning, which can be resource-intensive. Additionally, the model's performance may degrade with highly complex or ambiguous images. Computational resources are another concern, as training and inference require significant processing power, especially for large-scale deployments.
Future Prospects
The future of BLIP and similar models looks promising, with ongoing research focusing on improving efficiency and expanding capabilities. Efforts are underway to develop lighter versions for edge devices and integrate additional modalities like video and audio. Ethical considerations, such as addressing biases in generated captions, are also gaining attention. These advancements will likely enhance BLIP's applicability and performance in diverse scenarios.
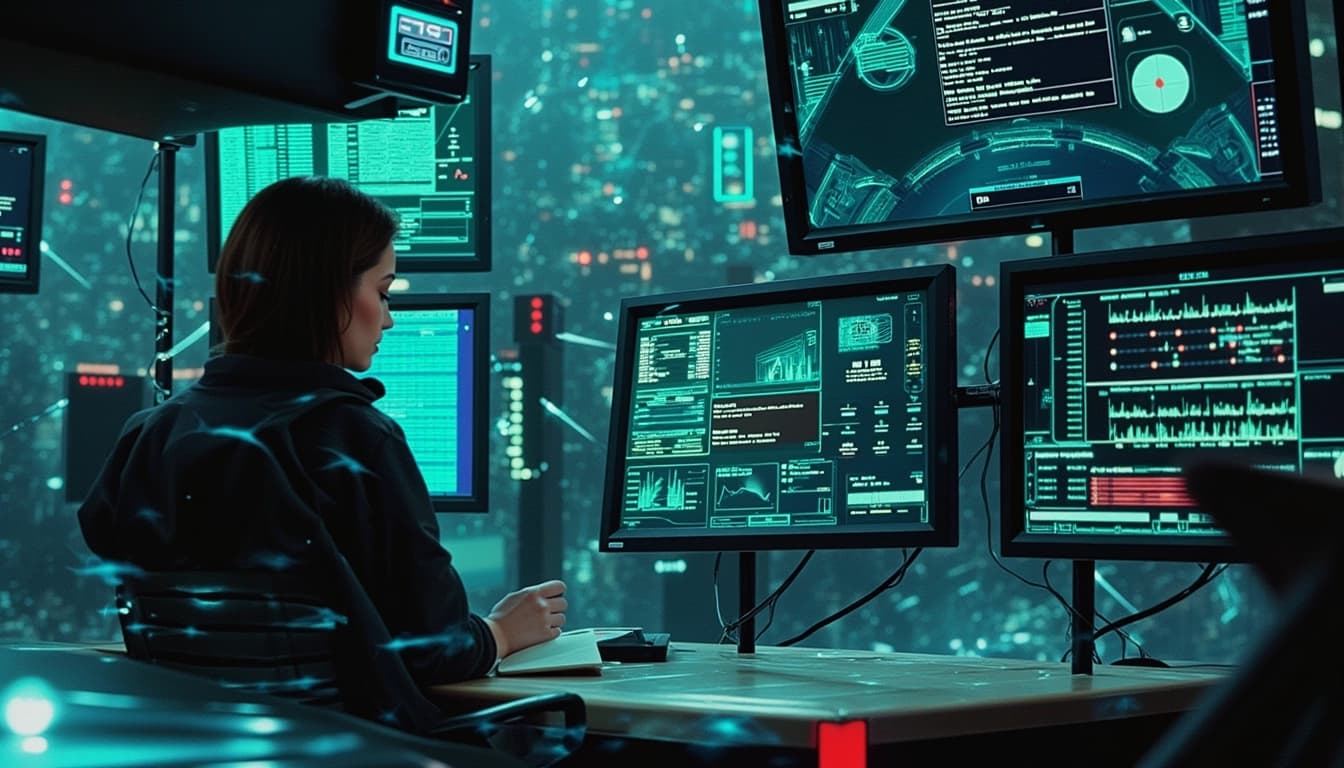
Conclusion
BLIP represents a significant leap forward in vision-language models, offering robust performance in image captioning and related tasks. Its ability to generate accurate and context-aware descriptions makes it a powerful tool across various domains. While challenges remain, ongoing research and development promise to address these limitations, paving the way for even more advanced and accessible solutions in the future.
- BLIP excels in vision-language understanding and generation.
- The model requires extensive data and computational resources.
- Future research aims to improve efficiency and ethical considerations.
BLIP-2, introduced by Salesforce in 2023, represents a significant advancement in vision-language pre-training. It builds upon the success of its predecessor, BLIP, by incorporating frozen pre-trained image encoders and large language models (LLMs) to achieve state-of-the-art performance with minimal trainable parameters. This innovative approach addresses the computational inefficiencies of previous models, making it a more scalable and efficient solution for vision-language tasks.
Key Features of BLIP-2
BLIP-2 leverages frozen pre-trained models to minimize computational costs while maximizing performance. By using a lightweight Querying Transformer (Q-Former), it bridges the gap between visual and textual representations. This design allows BLIP-2 to achieve impressive results on tasks like image-text retrieval, visual question answering (VQA), and image captioning with significantly fewer trainable parameters compared to traditional models.
Efficiency and Performance
One of the standout features of BLIP-2 is its ability to outperform larger models with fewer resources. For instance, it achieves higher accuracy on benchmarks like COCO and NoCaps while requiring less computational power. This efficiency makes it an attractive option for both research and practical applications, where resource constraints are a common challenge.
Applications of BLIP-2
BLIP-2's versatility extends to a wide range of applications, from automated image captioning to enhancing accessibility tools for visually impaired users. Its robust performance in zero-shot and fine-tuned settings makes it suitable for industries like healthcare, e-commerce, and entertainment, where accurate vision-language understanding is crucial.
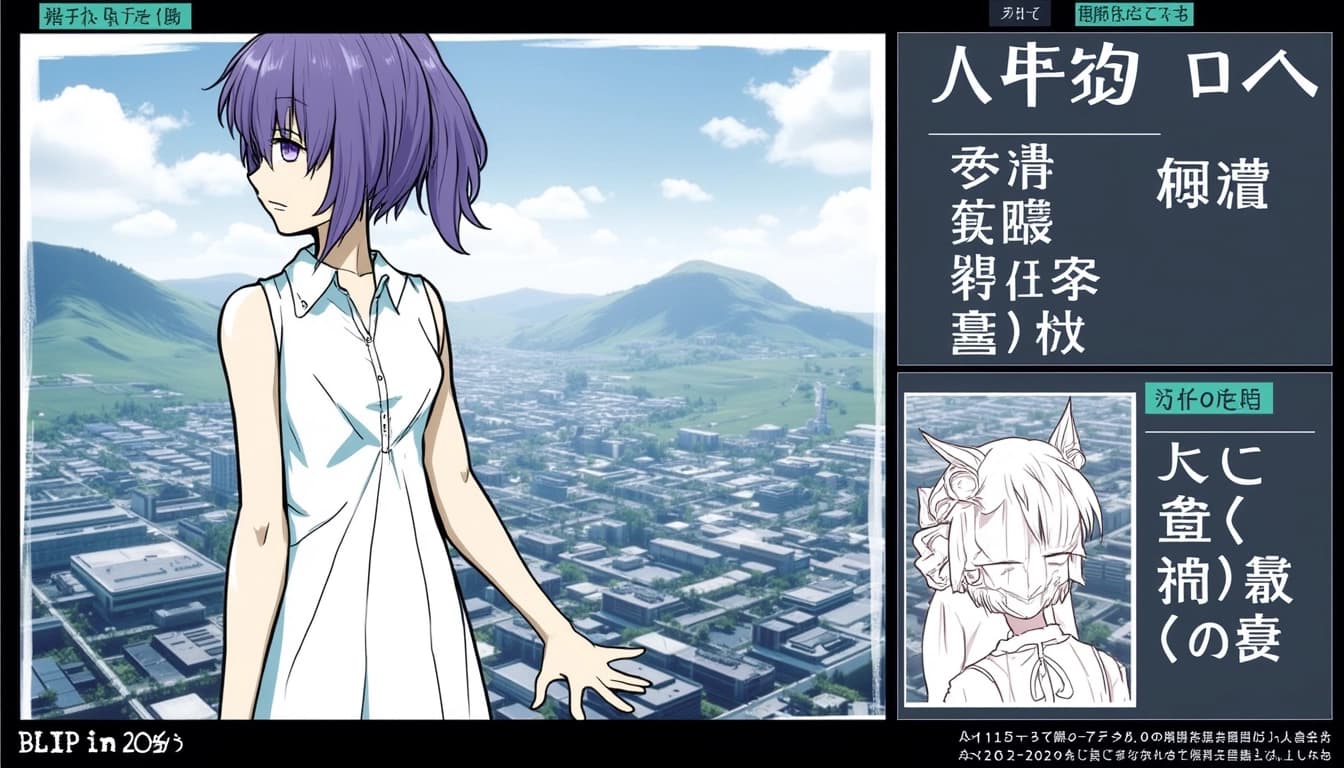
Comparison with Other Models
When compared to other vision-language models like Flamingo and CoCa, BLIP-2 stands out for its efficiency and competitive performance. The table provided in the article highlights its superior metrics in image-text retrieval, captioning, and VQA tasks. This comparison underscores BLIP-2's potential to set new standards in the field.
Future Directions and Conclusion
BLIP-2's innovative approach opens up exciting possibilities for future research and development. Its ability to leverage frozen models while maintaining high performance suggests a promising direction for reducing computational costs in AI. As the field evolves, BLIP-2 is likely to inspire further advancements in vision-language pre-training, solidifying its place as a benchmark model.
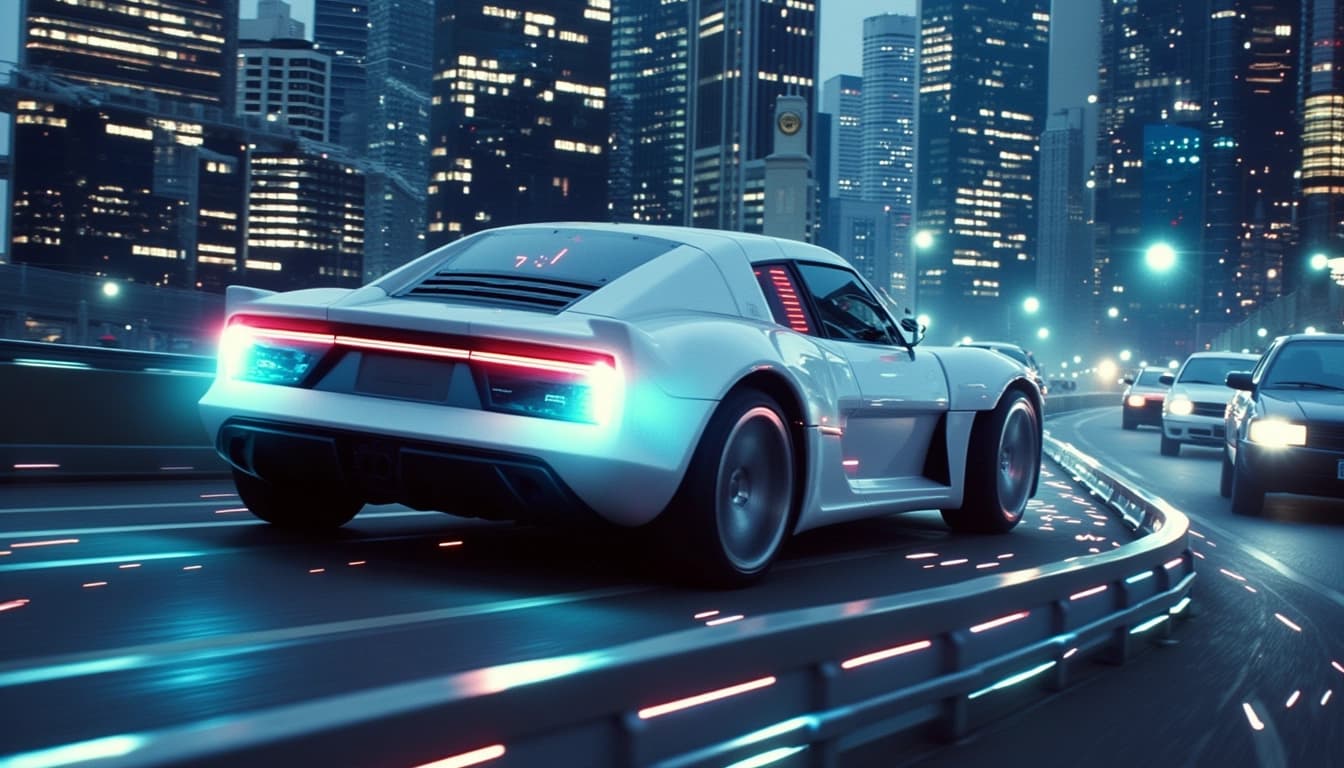
- BLIP-2 reduces computational costs by using frozen pre-trained models.
- It achieves state-of-the-art performance with fewer trainable parameters.
- The model excels in zero-shot and fine-tuned settings across various tasks.
Salesforce's BLIP (Bootstrapped Language-Image Pre-training) model is a groundbreaking advancement in the field of image captioning. It combines vision and language understanding to generate accurate and contextually relevant captions for images. The model is pre-trained on large datasets, enabling it to understand intricate details and relationships within images.
How BLIP Revolutionizes Image Captioning
BLIP leverages a multimodal mixture of encoder-decoder models to achieve state-of-the-art performance in image captioning. Unlike traditional models, BLIP uses bootstrapping to filter noisy web data, ensuring high-quality training. This approach significantly improves the model's ability to generate detailed and coherent captions.
Key Features of BLIP
One of the standout features of BLIP is its ability to perform both understanding-based and generation-based tasks. It can not only describe images but also answer questions about them. The model's flexibility makes it suitable for a wide range of applications, from social media to assistive technologies.
Applications of BLIP in Real-World Scenarios
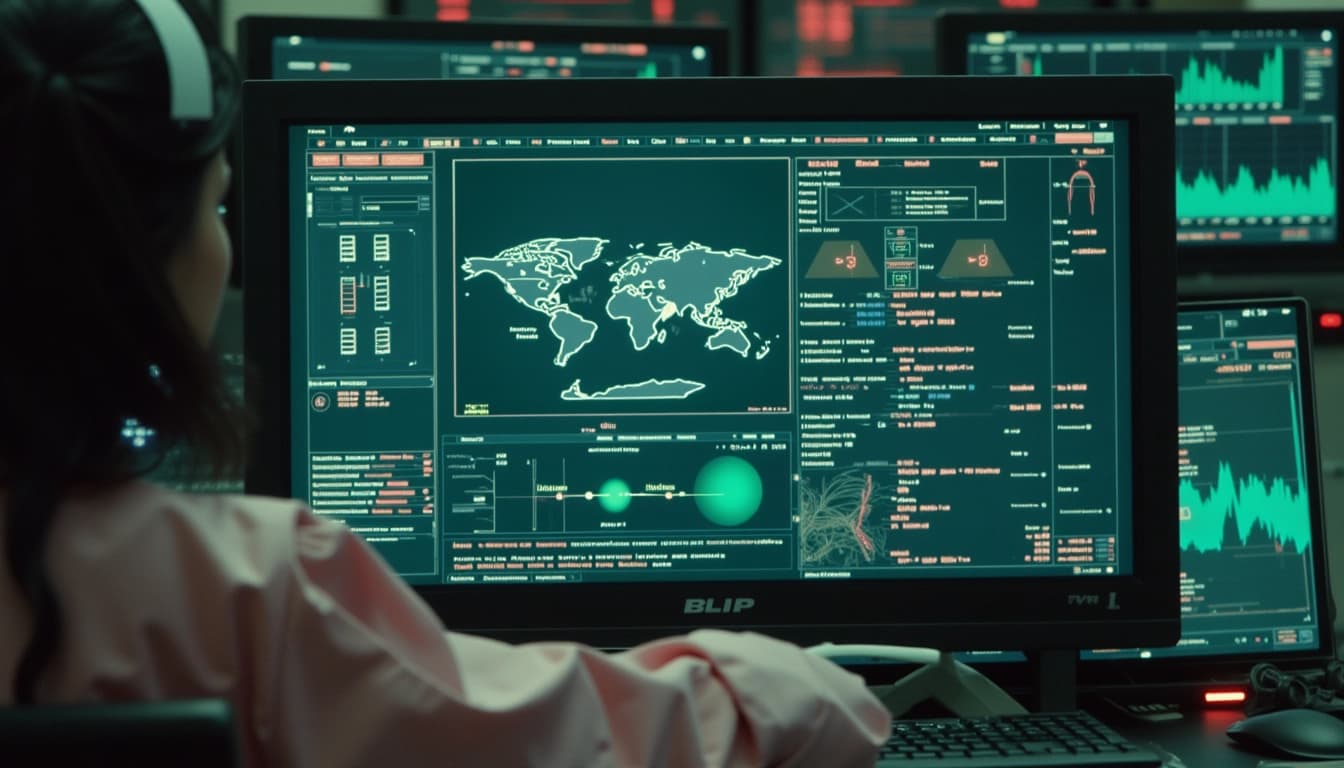
BLIP is being used in various industries to enhance user experiences. For instance, e-commerce platforms utilize it to generate product descriptions automatically. In healthcare, it aids in medical imaging by providing detailed captions for diagnostic purposes. The model's versatility is unlocking new possibilities across sectors.
Challenges and Future Directions
Despite its impressive capabilities, BLIP faces challenges such as handling ambiguous images and cultural biases in training data. Researchers are actively working on improving the model's robustness and fairness. Future iterations may incorporate even larger datasets and more sophisticated architectures.
Conclusion & Next Steps
BLIP represents a significant leap forward in image captioning technology. Its ability to generate detailed and context-aware captions makes it a valuable tool for numerous applications. As the model continues to evolve, we can expect even greater advancements in multimodal AI.
- BLIP combines vision and language understanding
- It uses bootstrapping to filter noisy data
- Applications span e-commerce, healthcare, and more