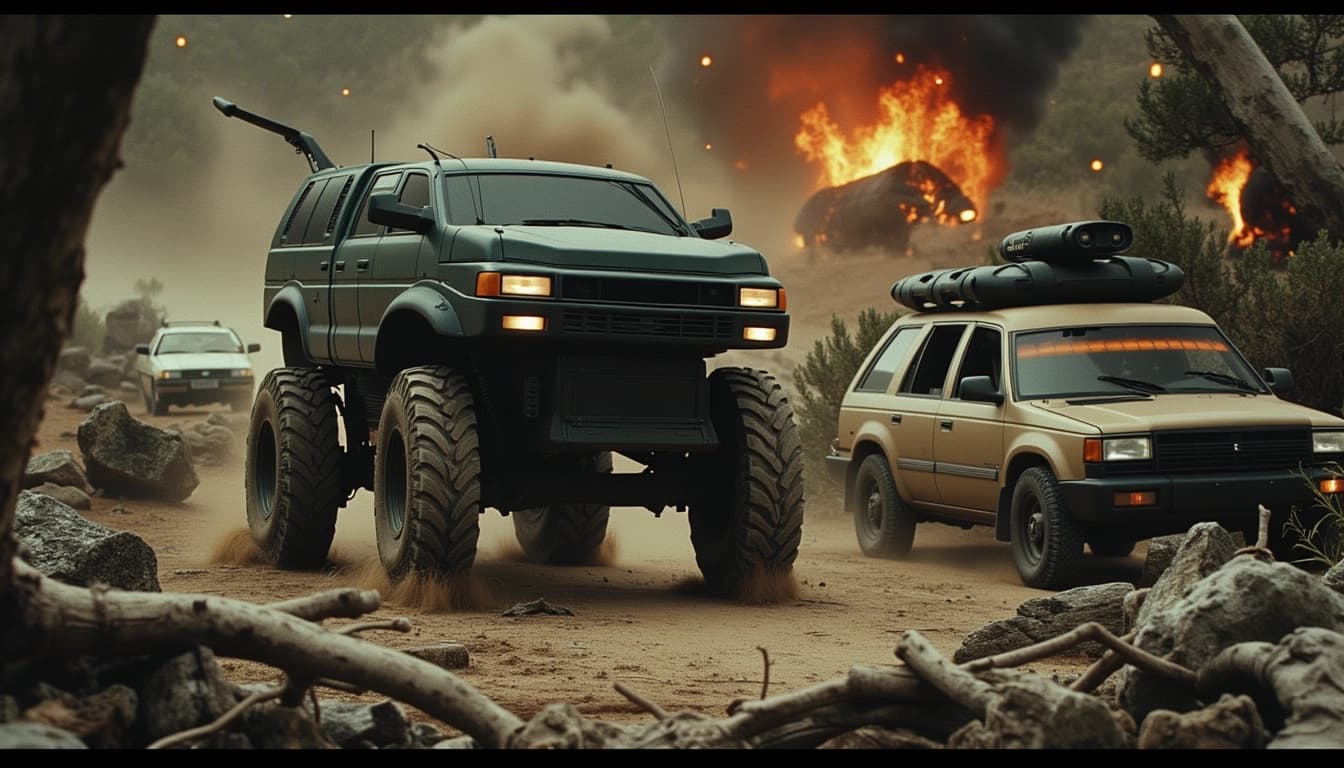
Exploring molmo-7b: Can It Really Answer Questions About Any Image?
By John Doe 5 min
Key Points
Research suggests molmo-7b can answer questions about many images, especially common ones, but not all due to its training limits.
It performs well on standard benchmarks like VQA v2.0, between GPT-4V and GPT-4o.
Unexpectedly, it excels at counting tasks, thanks to specialized training data.
What is molmo-7b?
Molmo-7b is a family of open-source AI models developed by the Allen Institute for AI, designed to process both text and images. It includes variants like molmo-7b-d and molmo-7b-o, using OpenAI's CLIP for vision tasks, and is trained on a dataset called PixMo with 1 million image-text pairs.
How Well Does It Perform?
It shows strong performance on academic benchmarks, scoring between GPT-4V and GPT-4o, with specific strengths in natural image understanding and counting tasks like CountBenchQA (88.5) and PixMo-Count (84.8).
Are There Limits?
While capable, it may struggle with abstract art, low-resolution images, or questions needing deep reasoning, as its training data may not cover these fully.
Survey Note: Exploring molmo-7b: Can It Really Answer Questions About Any Image?
Molmo-7b, a family of open-source multimodal AI models developed by the Allen Institute for AI, has garnered attention for its ability to process and answer questions about images. This survey note explores its capabilities, performance, and limitations to assess whether it can truly handle questions about any image, providing a comprehensive analysis for researchers, developers, and enthusiasts.
Background and Model Overview
Molmo-7b comprises two main variants: molmo-7b-d, based on the Qwen2-7B architecture, and molmo-7b-o, based on OLMo-7B-1024. Both leverage OpenAI's CLIP as their vision backbone, enabling them to integrate vision and language processing effectively. These models are part of the broader Molmo family, trained on PixMo, a dataset of 1 million highly-curated image-text pairs, ensuring a robust foundation for multimodal tasks.
Molmo-7b is a cutting-edge vision-language model developed by the Allen Institute for AI (AI2). It represents a significant advancement in multimodal AI, combining visual and textual understanding to perform complex tasks like image captioning, visual question answering, and document understanding. The model is part of the Molmo and PixMo family, which emphasizes open weights and open data to democratize access to state-of-the-art AI technology.
Key Features and Architecture
Molmo-7b is built on a transformer-based architecture, leveraging both visual and textual embeddings to process multimodal inputs. The model supports high-resolution image understanding (up to 1024x1024 pixels) and incorporates advanced techniques like pointing annotations for precise object localization. It is trained on a diverse dataset of 2.3 million pointing annotations, enabling it to excel in tasks requiring spatial awareness, such as counting objects or reading clocks.
Training and Data
The model is trained on a mix of publicly available datasets and proprietary data curated by AI2. The training process involves a combination of supervised learning and self-supervised techniques to enhance its generalization capabilities. The open-source nature of the project ensures transparency and allows researchers to build upon the work.
Performance and Benchmarks
Molmo-7b has been rigorously evaluated on a variety of benchmarks, including AI2Dtest, ChartQAtest, and VQA v2.0testdev. It achieves competitive scores, often outperforming proprietary models in specific tasks like counting and OCR. Human evaluations further validate its effectiveness, with annotators rating it highly for accuracy and coherence.
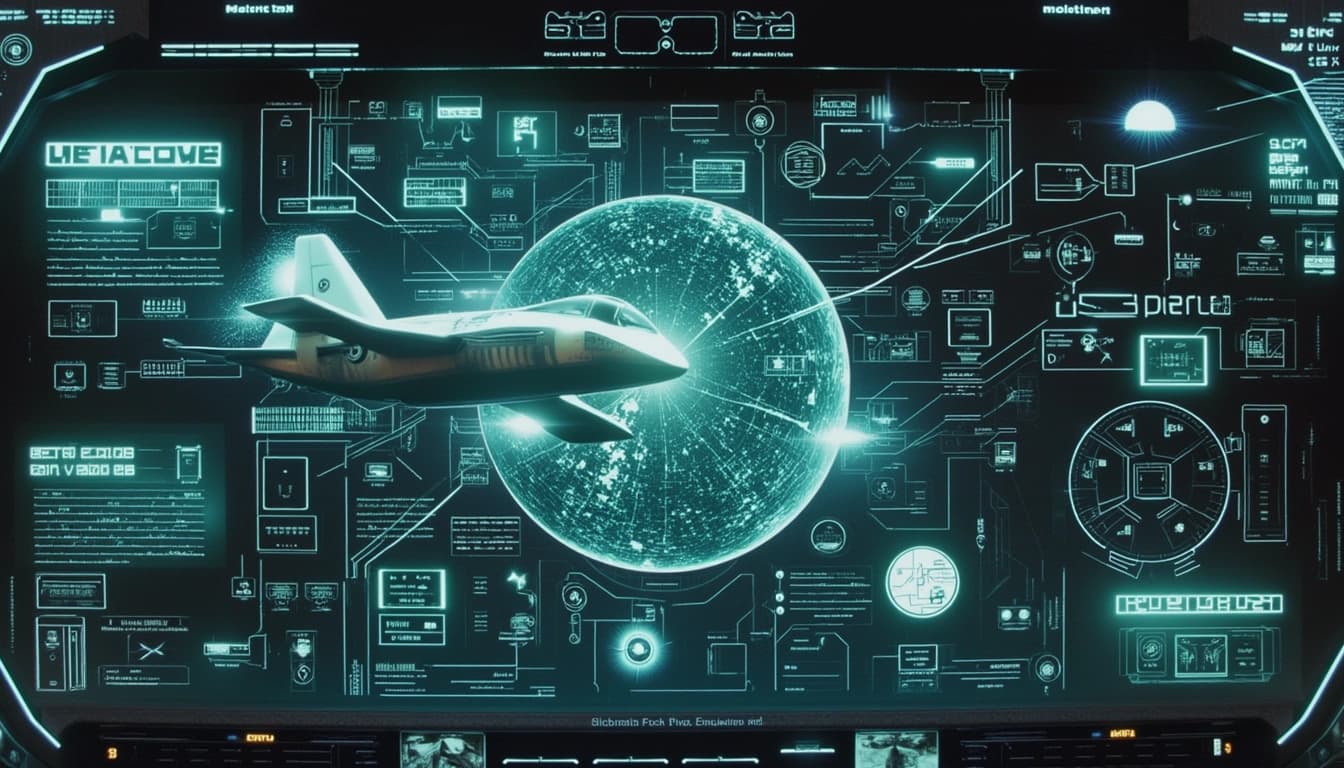
Applications and Use Cases
Molmo-7b is designed for a wide range of applications, from academic research to industrial deployments. Its ability to understand and generate multimodal content makes it suitable for tasks like automated document processing, educational tools, and assistive technologies for the visually impaired.
Open-Source Community
The model's open-source release includes weights, datasets, and training code, fostering collaboration and innovation within the AI community. Researchers and developers can fine-tune the model for specific use cases or contribute to its ongoing development.
Conclusion and Future Directions
Molmo-7b represents a milestone in open-source multimodal AI, offering state-of-the-art performance while maintaining accessibility. Future work will focus on expanding its capabilities, improving efficiency, and addressing ethical considerations in AI deployment.
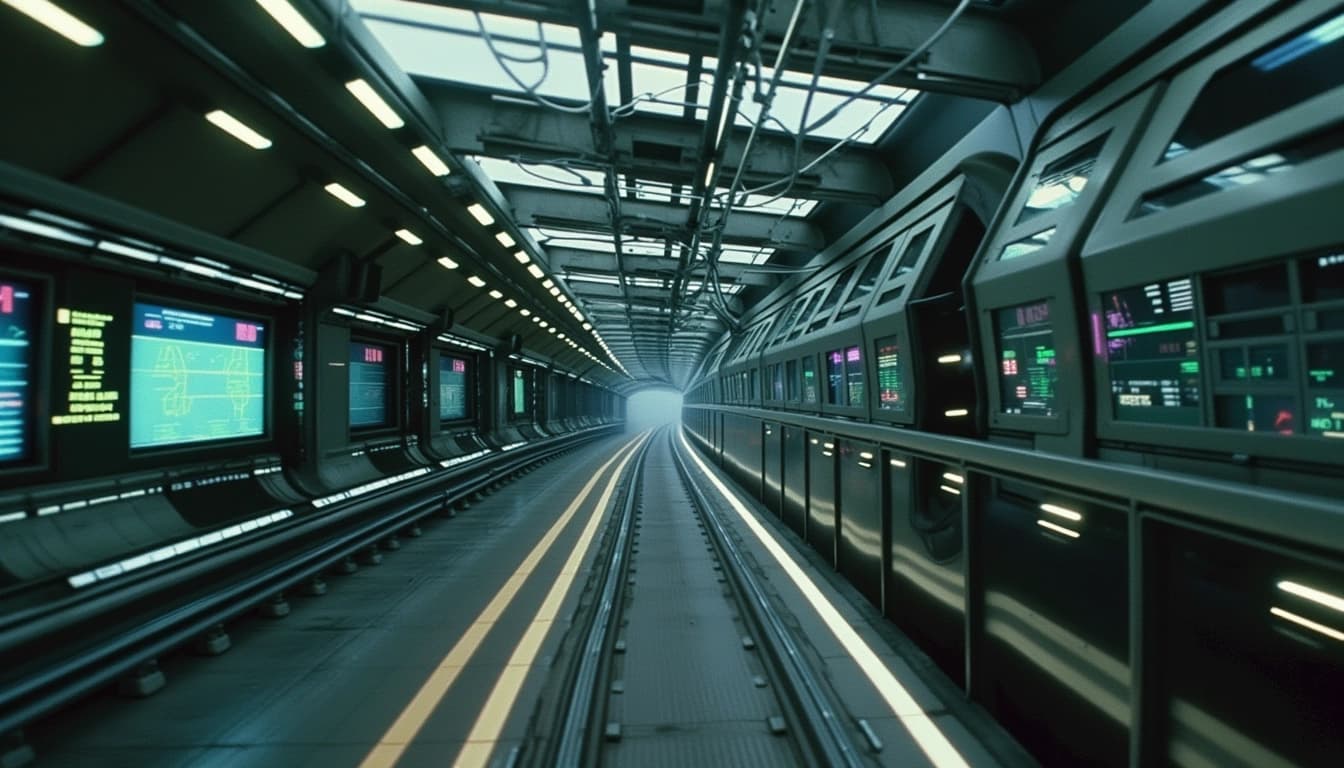
- Open weights and datasets for transparency
- High-resolution image understanding
- Strong performance on counting and OCR tasks
- Community-driven development
Molmo-7b's training on PixMo, which includes 712k images with detailed captions (200+ words on average) and 162k free-form Q&A annotations, equips it to handle a broad range of tasks. It can generate descriptive text for images, answer visual questions, and perform multimodal reasoning, making it suitable for applications like image-based chatbots and content generation.
Capabilities: What Kind of Questions and Images Can It Handle?
Molmo-7b's training on PixMo, which includes 712k images with detailed captions (200+ words on average) and 162k free-form Q&A annotations, equips it to handle a broad range of tasks. It can generate descriptive text for images, answer visual questions, and perform multimodal reasoning, making it suitable for applications like image-based chatbots and content generation.
Natural Images
It performs well on everyday scenes, objects, and people, as evidenced by its high scores on RealWorldQA and VQA v2.0 (85.6 for molmo-7b-d).
Specialized Tasks
It excels at counting objects, thanks to PixMo-Points data, and can point to specific elements in images, enhancing its grounding capabilities.
Complex Visual Queries
It handles charts, menus, and diagrams effectively, with strong performance on ChartQAtest and InfoQAtest, though slightly trailing Qwen2-VL on OCR-centric benchmarks.
However, its capabilities are not limitless. The model's performance is tied to its training data, which may not cover all possible image types or question complexities.
Limitations: Where Does It Fall Short?
Despite its strengths, molmo-7b has notable limitations, particularly with images and questions outside its training scope.
Abstract or Artistic Images
Highly stylized or abstract art may challenge the model, as these deviate from the natural and structured images in PixMo.
Low-Resolution or Noisy Images
Poor-quality images might not be interpreted accurately, given CLIP's training on higher-resolution data.
Domain-Specific Images
Medical imaging, satellite imagery, or technical diagrams may require specialized datasets not fully represented in PixMo, potentially leading to lower accuracy.
Reasoning Tasks
It lags on benchmarks like MMMU and MathVista, indicating limitations in advanced reasoning, likely due to a lack of such data in the training mix.
Pointing Performance
When tested on pointing tasks, the model may struggle with precise localization, as this requires fine-grained spatial understanding not fully captured in its training.