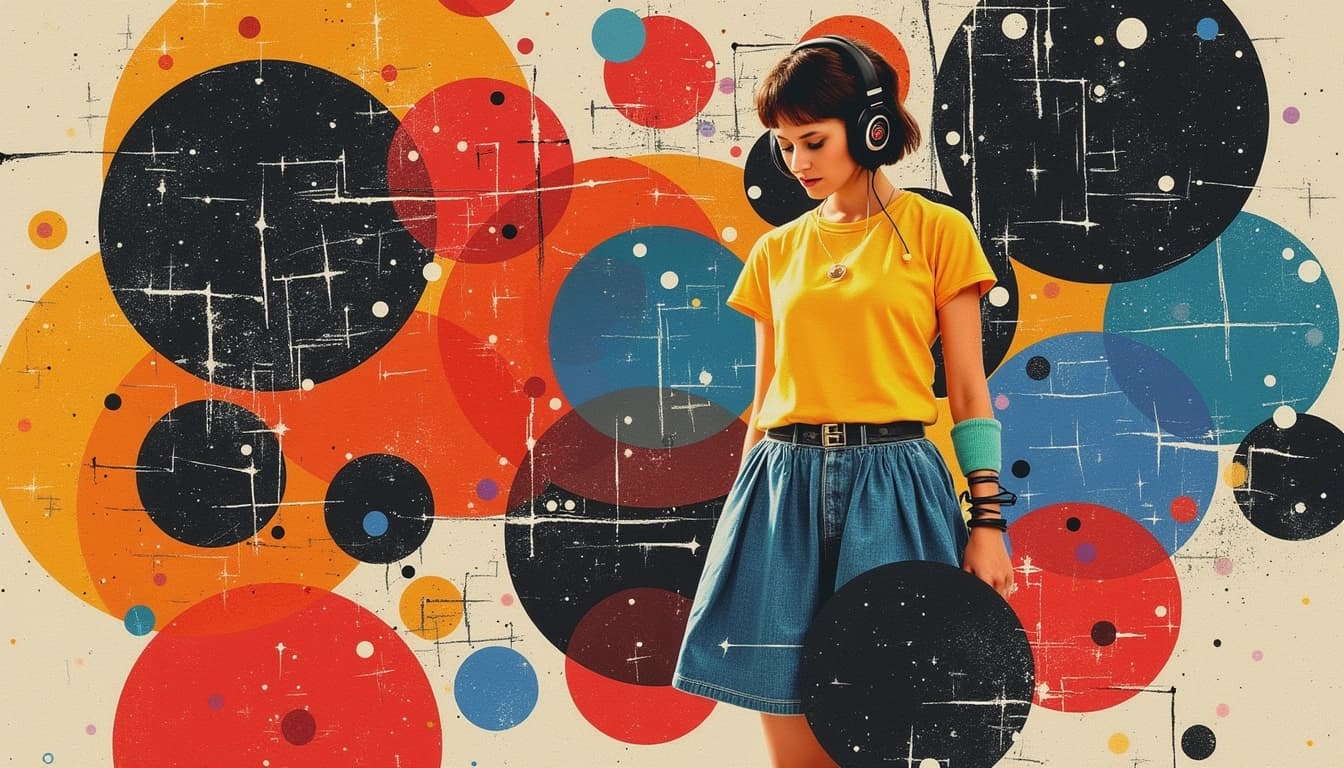
Introduction to Kandinsky-2.2
By John Doe 5 min
Key Points
Research suggests Kandinsky-2.2, a multilingual text-to-image AI model, is best for global projects needing images from text in multiple languages.
It seems likely that its ControlNet support makes it ideal for artistic projects requiring specific image controls, like using sketches or depth maps.
The evidence leans toward its use in high-quality image generation for marketing, education, real estate, and fashion, enhancing visual content creation.
Introduction to Kandinsky-2.2
Kandinsky-2.2 is an advanced, open-source, multilingual text-to-image latent diffusion model developed by ai-forever. It excels at generating high-quality images from textual descriptions, supporting multiple languages and offering enhanced control over the image generation process. This makes it a versatile tool for various real-world applications, particularly where visual content needs to be both diverse and precise.
Key Features and Benefits
Kandinsky-2.2 stands out with several key features:
- **Multilinguality:** It supports multiple languages, making it suitable for global projects where content needs to be accessible in different linguistic contexts.
- **ControlNet Support:** This feature allows for controlled image generation using additional inputs like depth maps or sketches, enabling precise manipulation for specific artistic or design needs.
- **Improved Image Encoder:** Utilizing CLIP-ViT-G, it enhances the model's ability to understand text better and generate aesthetically pleasing, high-quality images.
Best Use Cases in Real Projects
Given its capabilities, Kandinsky-2.2 is particularly effective in the following areas:
- **Global Marketing and Advertising:** Generate images for campaigns in multiple languages, ensuring culturally relevant visuals for diverse audiences. For example, creating ads in English, Spanish, and Mandarin without needing separate models.
- **Artistic Projects with Specific Controls:** Ideal for artists who need
Kandinsky-2.2, developed by ai-forever, is a cutting-edge, open-source, multilingual text-to-image latent diffusion model. It represents a significant advancement over its predecessors, such as Kandinsky-2.1, by incorporating a more powerful image encoder (CLIP-ViT-G) and introducing ControlNet support. These enhancements enable the model to generate visually appealing images with improved text comprehension, making it a versatile tool for various creative and practical applications.
Key Features of Kandinsky-2.2
One of the standout features of Kandinsky-2.2 is its multilingual capability, which allows it to generate images from text prompts in multiple languages. This makes it particularly useful for global applications where language diversity is essential. Additionally, the model supports high-resolution image generation (up to 1024x1024 pixels) and includes advanced features like inpainting and ControlNet for guided image creation. The integration of ControlNet enables users to steer the generation process with sketches or depth maps, adding a layer of precision to the output.
Multilingual Support
The multilingual support in Kandinsky-2.2 is a game-changer for content creators working across different linguistic contexts. Whether you're generating educational materials, marketing content, or artistic visuals, the model can interpret and respond to prompts in various languages. This feature eliminates the need for manual translation or separate models for different languages, streamlining the creative process and expanding accessibility.
Practical Applications
Kandinsky-2.2 can be applied in numerous real-world scenarios, from marketing and advertising to education and design. For instance, marketers can use it to create localized ad visuals without the need for extensive photoshoots. Educators can generate multilingual visual aids to enhance learning experiences. Designers can leverage its ControlNet capabilities to produce detailed architectural or fashion designs based on specific inputs. The possibilities are vast and varied.
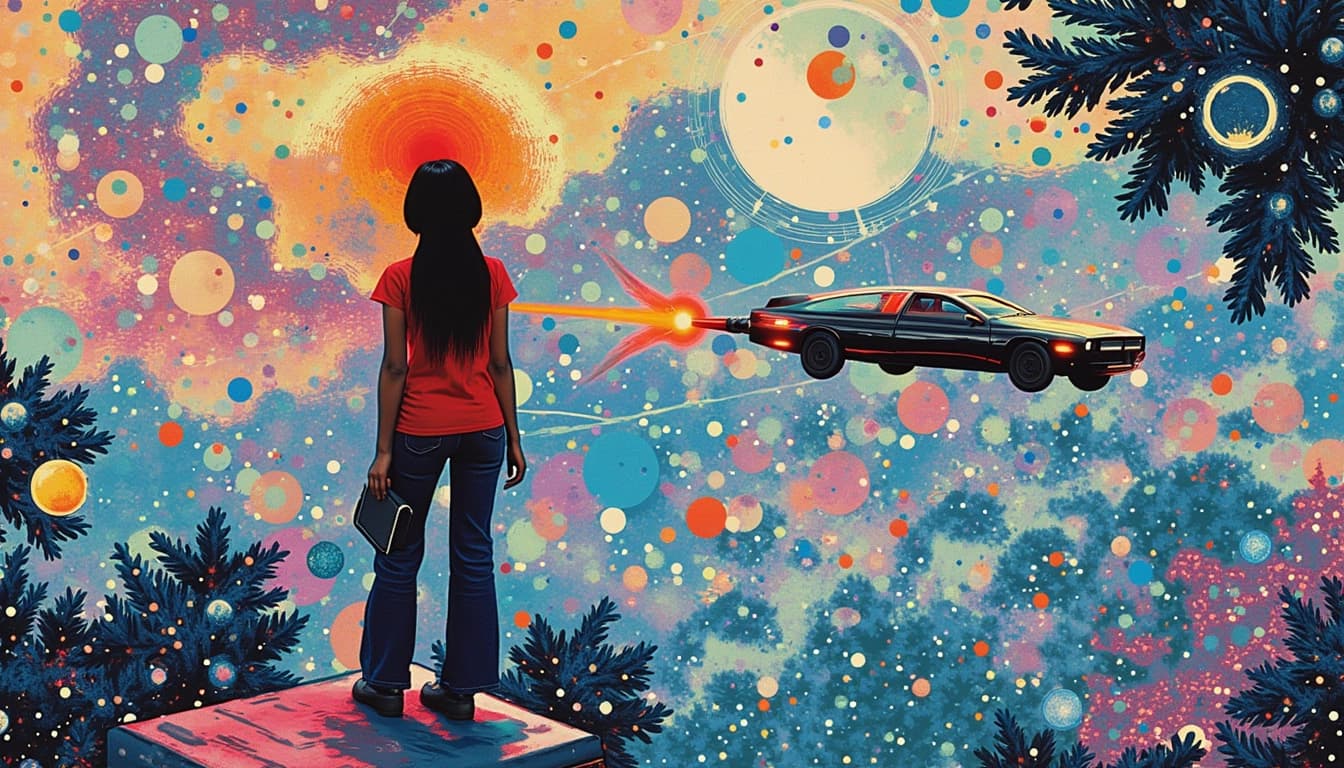
Getting Started with Kandinsky-2.2
To begin using Kandinsky-2.2, you can explore the official GitHub repository for documentation and code examples. The Replicate API offers a straightforward way to integrate the model into your applications, while Colab notebooks provide hands-on experience with inference and fine-tuning. Whether you're a developer, designer, or content creator, these resources make it easy to harness the power of Kandinsky-2.2 for your projects.
Conclusion & Next Steps
Kandinsky-2.2 is a powerful and flexible tool that opens up new possibilities for text-to-image generation. Its multilingual support, high-resolution output, and ControlNet integration set it apart from other models in the field. By exploring its features and experimenting with its capabilities, you can unlock its full potential for your creative and professional endeavors. The next step is to dive in, test the model, and see how it can enhance your workflow.
- Explore the GitHub repository for documentation and examples.
- Use the Replicate API for easy integration into your projects.
- Experiment with Colab notebooks for hands-on learning and fine-tuning.
Kandinsky-2.2 is a cutting-edge text-to-image diffusion model developed by the Kandinsky community, designed to generate high-quality images from textual descriptions. It builds upon the success of its predecessors, incorporating advanced features like multilingual support and ControlNet integration, making it a versatile tool for various applications.
Key Features of Kandinsky-2.2
The model stands out due to its multilingual capabilities, leveraging a robust text encoder to handle multiple languages effectively. This makes it ideal for global projects where content needs to be generated in diverse linguistic contexts. Additionally, its ControlNet support allows for precise control over image generation, enabling users to guide the output with specific inputs like depth maps or edge maps.
Multilingual Support
Kandinsky-2.2's multilingual text encoder ensures that it can process and generate content in various languages, making it a valuable asset for international applications. This feature is particularly beneficial for projects targeting audiences across different regions, as it eliminates language barriers in image generation.
ControlNet Integration
The integration of ControlNet allows users to condition the model on additional inputs, such as depth or edge maps, to achieve more controlled and precise image outputs. This is especially useful for tasks requiring specific structural guidance, such as architectural visualizations or detailed art projects.
Technical Architecture
Kandinsky-2.2's architecture includes several advanced components, such as a text encoder, a diffusion image prior, a CLIP image encoder, a latent diffusion U-Net, and a MoVQ encoder/decoder. These components work together to ensure high-quality image synthesis, supporting tasks like text-to-image, image-to-image, and inpainting.
Applications and Use Cases
The model's versatility makes it suitable for a wide range of applications, from creative art projects to practical uses like marketing and design. Its ability to generate controlled and multilingual content opens up possibilities for global campaigns, educational materials, and more.
Conclusion & Next Steps
Kandinsky-2.2 represents a significant advancement in text-to-image generation, offering multilingual support and precise control features. Its robust architecture and wide range of applications make it a powerful tool for both creative and practical projects. Future developments may further enhance its capabilities, making it even more versatile and user-friendly.
- Explore multilingual text-to-image generation
- Experiment with ControlNet for precise image control
- Integrate Kandinsky-2.2 into your creative or professional projects
Kandinsky-2.2 is a cutting-edge text-to-image diffusion model developed by the Kandinsky community, building on the success of its predecessor, Kandinsky-2.1. This new version introduces several enhancements, including improved text understanding, higher image quality, and better performance with multilingual prompts. The model is designed to generate highly detailed and realistic images, especially in wider scenes and higher resolutions, such as 1024x1024 pixels.
Model Features and Capabilities
Kandinsky-2.2 excels in generating images with intricate details and realistic textures, making it a strong contender in the text-to-image generation space. The model supports various tasks, including text-to-image, image-to-image, inpainting, and ControlNet-depth applications. Its ability to handle multilingual inputs and complex prompts sets it apart from other models like Stable Diffusion, particularly in creative and controlled generation scenarios.
Checkpoints and Resources
The model provides specialized checkpoints for different tasks, such as the Prior Checkpoint for initial processing and the Decoder Checkpoint for text-to-image and image-to-image tasks. Additionally, there are dedicated checkpoints for inpainting and ControlNet-depth, offering flexibility for various use cases. These resources, along with Jupyter notebooks available on GitHub, make it easier for developers to implement and experiment with the model.
Comparative Analysis with Other Models
When compared to models like Stable Diffusion, Kandinsky-2.2 demonstrates superior performance in text understanding and image quality, especially for creative prompts. However, some users have noted an 'airbrushed' effect in certain outputs, which may not be desirable for all applications. Despite this, the model's strengths in multilingual support and controlled generation make it a valuable tool for diverse projects.
Practical Implementation
Implementing Kandinsky-2.2 is straightforward, thanks to the provided code snippets and documentation. For example, generating a text-to-image can be done with just a few lines of Python code, as shown in the article. The model's flexibility and ease of use make it accessible to both beginners and experienced developers.
Conclusion & Next Steps
Kandinsky-2.2 represents a significant advancement in text-to-image generation, offering improved quality and versatility. Its ability to handle complex prompts and multilingual inputs makes it a powerful tool for creative projects. Future developments could focus on refining the model's output to reduce the 'airbrushed' effect and further enhancing its performance in niche applications.
- Explore the model's checkpoints for different tasks
- Experiment with multilingual prompts to leverage its strengths
- Compare outputs with other models like Stable Diffusion for specific use cases
Kandinsky-2.2 is a significant advancement in AI-driven image generation, offering multilingual text-to-image capabilities and enhanced control features. It builds upon its predecessor, Kandinsky-2.1, by incorporating improved text understanding and image quality, making it a versatile tool for various applications.
Key Features of Kandinsky-2.2
The model supports multiple languages, enabling users to generate images from prompts in different tongues, which is particularly useful for global projects. Additionally, it integrates with ControlNet, allowing for precise control over image generation using sketches or depth maps. This feature is ideal for applications requiring detailed and specific visual outputs.
Multilingual Capabilities
Kandinsky-2.2's ability to process prompts in various languages sets it apart from many other models. This makes it highly suitable for international marketing campaigns, educational materials, and other projects requiring multilingual support. The model ensures that the generated images are culturally and contextually relevant across different regions.
Comparison with Other Models
While Kandinsky-2.2 excels in multilingual support and control features, it has some limitations in generating photorealistic textures compared to models like SDXL 0.9. However, its open-source nature and permissive license make it accessible for a wide range of real-world applications, as highlighted in various blog posts and articles.
Best Use Cases in Real Projects
Kandinsky-2.2 is particularly well-suited for projects that benefit from its multilingual and control capabilities. Below are some detailed use cases where the model can be effectively utilized to achieve high-quality results.
Global Marketing and Advertising
The model can generate images for campaigns in multiple languages, ensuring culturally relevant visuals. For example, creating ads in English, Spanish, and Mandarin for a global brand launch. This reduces the need for separate models and streamlines content creation for international markets.
Artistic Projects with Specific Controls
Using ControlNet, users can guide image generation with sketches or depth maps, making it ideal for architectural visualizations, art restoration, or game asset creation. For instance, generating a realistic building image from a floor plan becomes straightforward with precise control features.
Conclusion & Next Steps
Kandinsky-2.2 offers a robust set of features that make it a valuable tool for various applications, from marketing to education. Its multilingual support and control capabilities provide unique advantages, though it may not match some competitors in photorealism. Future developments could focus on enhancing texture generation to broaden its appeal further.
- Multilingual text-to-image generation
- Integration with ControlNet for precise control
- Open-source and permissive licensing
- Ideal for global marketing and artistic projects
Kandinsky-2.2 is an advanced text-to-image diffusion model developed by Sber AI, building upon the capabilities of its predecessor, Kandinsky-2.1. It integrates multilingual support, allowing users to generate images from prompts in multiple languages, including English, Russian, Chinese, and more. The model also features ControlNet integration, enabling precise control over generated images through additional inputs like sketches or depth maps.
Key Features of Kandinsky-2.2
One of the standout features of Kandinsky-2.2 is its multilingual text encoder, which supports a wide range of languages, making it accessible to a global audience. The model also includes enhanced image quality and resolution, with outputs reaching up to 1024x1024 pixels. Additionally, the integration of ControlNet allows for more detailed and controlled image generation, which is particularly useful for professional applications.
Multilingual Support
The multilingual capabilities of Kandinsky-2.2 are powered by a custom text encoder that can process inputs in various languages. This feature is especially beneficial for users who need to generate images based on non-English prompts, as it eliminates the need for manual translation and ensures more accurate results.
ControlNet Integration
ControlNet integration allows users to guide the image generation process using additional inputs such as edge maps, depth maps, or segmentation masks. This level of control is invaluable for applications requiring precise adherence to specific structural or compositional guidelines, such as architectural visualization or product design.
Performance and Benchmarks
Kandinsky-2.2 has demonstrated superior performance in various benchmarks, particularly in multilingual and high-resolution image generation tasks. According to evaluations, it outperforms many open-source alternatives in terms of both quality and versatility. The model's ability to handle complex prompts and produce detailed, coherent images makes it a strong contender in the text-to-image space.
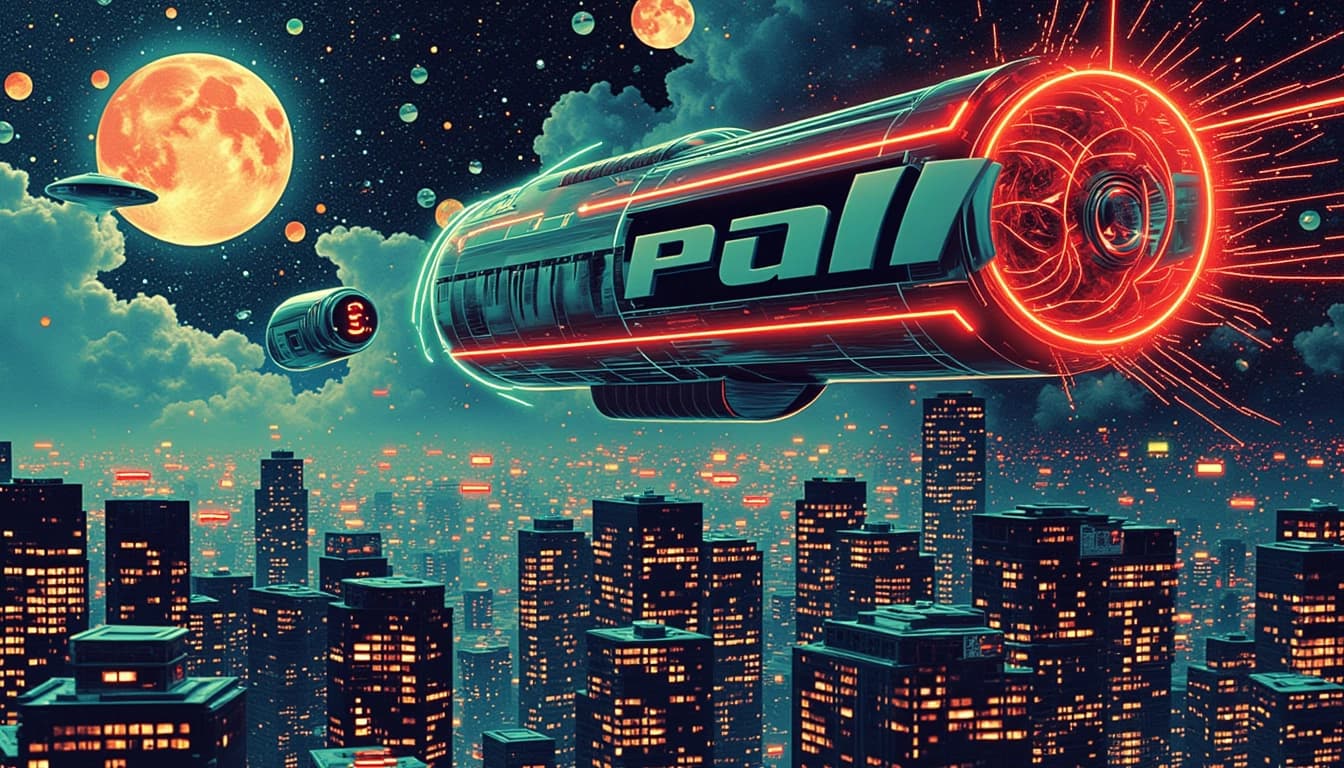
Use Cases and Applications
Kandinsky-2.2 is well-suited for a variety of applications, including marketing, education, entertainment, and design. For instance, marketers can use it to create visually appealing ads from multilingual text descriptions, while educators can generate illustrative content for teaching materials. The model's ControlNet features also make it ideal for architectural and product design, where precision is key.
Marketing and Advertising
In the marketing sector, Kandinsky-2.2 can be used to quickly generate high-quality visuals for campaigns targeting diverse linguistic audiences. The ability to produce images from non-English prompts ensures that the content resonates with local markets, enhancing engagement and effectiveness.
Education and E-Learning
Educators and e-learning platforms can leverage Kandinsky-2.2 to create custom illustrations and diagrams based on textual descriptions. This is particularly useful for subjects that require visual aids, such as science and history, where accurate and engaging visuals can significantly improve learning outcomes.
Conclusion & Next Steps
Kandinsky-2.2 represents a significant advancement in text-to-image generation, offering multilingual support, high-resolution outputs, and precise control through ControlNet. Its versatility and performance make it a valuable tool for a wide range of applications, from marketing to education. As the model continues to evolve, we can expect even more features and improvements that will further enhance its capabilities.
- Explore the official GitHub repository for code and documentation
- Experiment with the Replicate API for easy deployment
- Try out the Colab notebooks for quick inference examples
Kandinsky-2.2 is a state-of-the-art multilingual text-to-image diffusion model that builds upon its predecessor, Kandinsky-2.1, with significant improvements in quality and versatility. It leverages a latent diffusion architecture and integrates advanced components like the MoVQ encoder/decoder and a multilingual CLIP model, enabling high-quality image generation from text prompts in multiple languages.
Key Features and Innovations
Kandinsky-2.2 introduces several groundbreaking features, including a new image encoder, U-Net architecture, and a multilingual text encoder. These enhancements allow for better control over image generation, higher resolution outputs, and improved performance across diverse languages. The model also supports advanced techniques like inpainting and image-to-image transformations, making it a versatile tool for creative applications.
Multilingual Capabilities
One of the standout features of Kandinsky-2.2 is its ability to process text prompts in multiple languages, thanks to the integration of the XLM-Roberta multilingual text encoder. This makes it particularly useful for global applications, where users can generate images from prompts in their native language without needing translation.
Performance and Quality
Kandinsky-2.2 has been benchmarked against other leading models like Stable Diffusion and Midjourney, showing competitive results in terms of image quality and prompt adherence. The model excels in generating detailed and aesthetically pleasing images, though some users note a slight 'airbrushed' effect in photorealistic scenarios.
Open-Source and Accessibility
Kandinsky-2.2 is fully open-source, released under the Apache 2.0 license, allowing developers to freely use, modify, and distribute the model. The team behind Kandinsky-2.2 has also provided comprehensive resources, including Colab notebooks for text-to-image, image-to-image, and inpainting tasks, making it easy for developers to get started.
Community and Collaboration
The open-source nature of Kandinsky-2.2 has fostered a vibrant community of developers and researchers who contribute to its continuous improvement. Collaborative efforts have led to the development of additional tools and integrations, such as the Kandinsky-2.2 API on Replicate, which simplifies deployment for real-world applications.
Practical Applications
Kandinsky-2.2 is being used across various industries, from marketing and advertising to education and design. Its ability to generate high-quality images from multilingual prompts makes it particularly valuable for global campaigns and educational materials. The model's fine-tuning capabilities also allow for specialized use cases, such as generating medical illustrations or architectural visualizations.
Conclusion and Future Directions
Kandinsky-2.2 represents a significant leap forward in text-to-image generation, combining multilingual support, high-quality outputs, and open-source accessibility. Future developments may focus on enhancing photorealism, reducing computational requirements, and expanding the model's capabilities to include video generation and other multimedia formats.
- Multilingual text-to-image generation
- High-quality outputs with advanced diffusion techniques
- Open-source and community-driven development
- Versatile applications across industries
Kandinsky-2.2 is the latest iteration of the open-source text-to-image diffusion model developed by the Kandinsky community. It builds upon the success of its predecessors, offering enhanced capabilities in generating high-quality images from textual descriptions. The model is designed to be versatile, supporting various tasks such as inpainting and depth control through ControlNet integration.
Key Features of Kandinsky-2.2
Kandinsky-2.2 stands out due to its modular architecture, which includes separate checkpoints for the prior and decoder components. This design allows for greater flexibility in fine-tuning and customization. The model also supports inpainting, enabling users to modify specific parts of an image while preserving the rest. Additionally, the integration of ControlNet-depth provides advanced control over the spatial composition of generated images.
Prior and Decoder Checkpoints
The prior checkpoint is responsible for generating latent representations from textual inputs, while the decoder checkpoint translates these representations into high-resolution images. This separation of tasks ensures efficient training and inference, making the model more accessible for researchers and developers.
Getting Started with Kandinsky-2.2
To begin using Kandinsky-2.2, users can access the model checkpoints on Hugging Face. The community provides detailed documentation and example notebooks to facilitate quick adoption. For instance, the inference example Colab notebook demonstrates how to generate images from text prompts, while the fine-tuning notebook guides users through the process of adapting the model to specific use cases using LoRA.
Applications and Use Cases
Kandinsky-2.2 is suitable for a wide range of applications, from creative art generation to practical tasks like image editing and augmentation. Its open-source nature encourages collaboration and innovation, allowing the community to continuously improve and expand its capabilities.
Conclusion & Next Steps
Kandinsky-2.2 represents a significant advancement in open-source AI image models, offering robust performance and flexibility. Whether you're a researcher, developer, or creative professional, this model provides a powerful tool for exploring the possibilities of text-to-image generation. The next steps involve experimenting with the provided notebooks, fine-tuning the model for specific tasks, and contributing to the community's efforts to enhance its features.
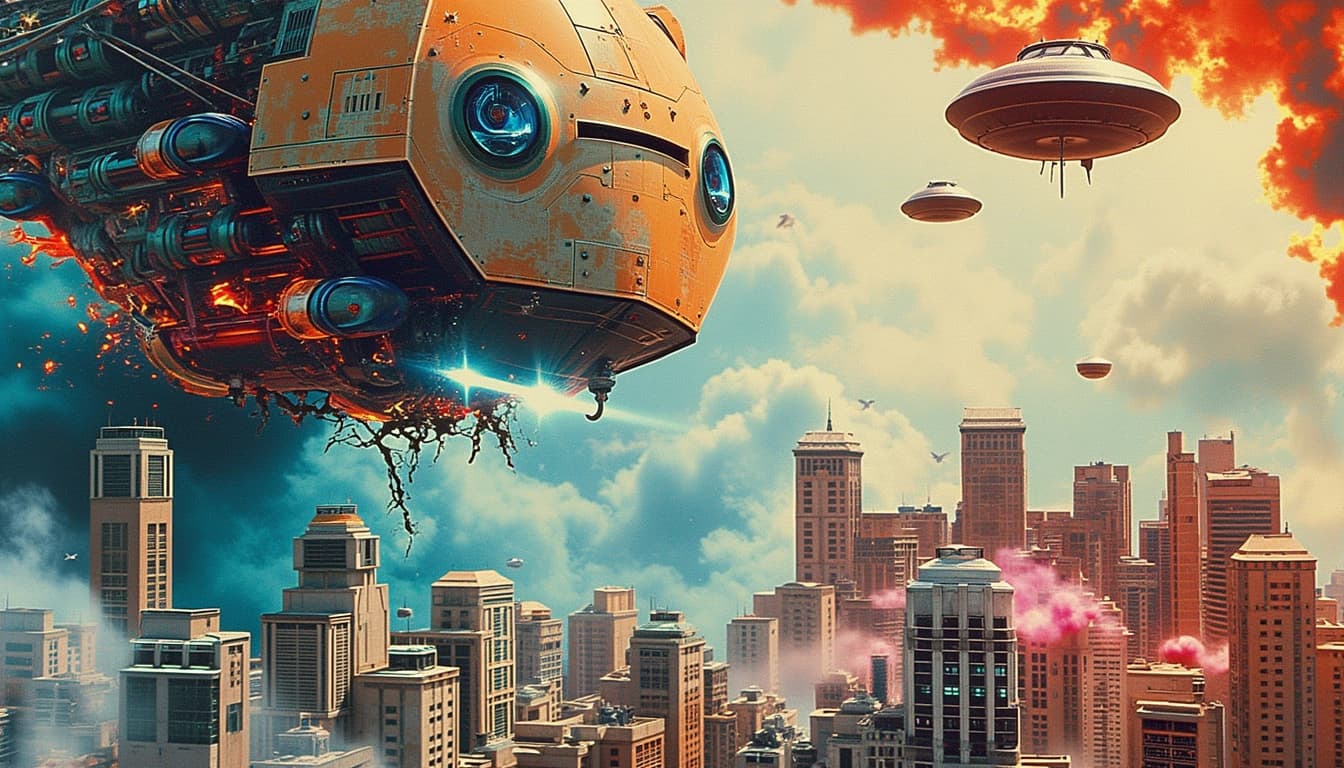
- Explore the Kandinsky-2.2 checkpoints on Hugging Face
- Try out the inference and fine-tuning Colab notebooks
- Join the Kandinsky community to contribute and share your work