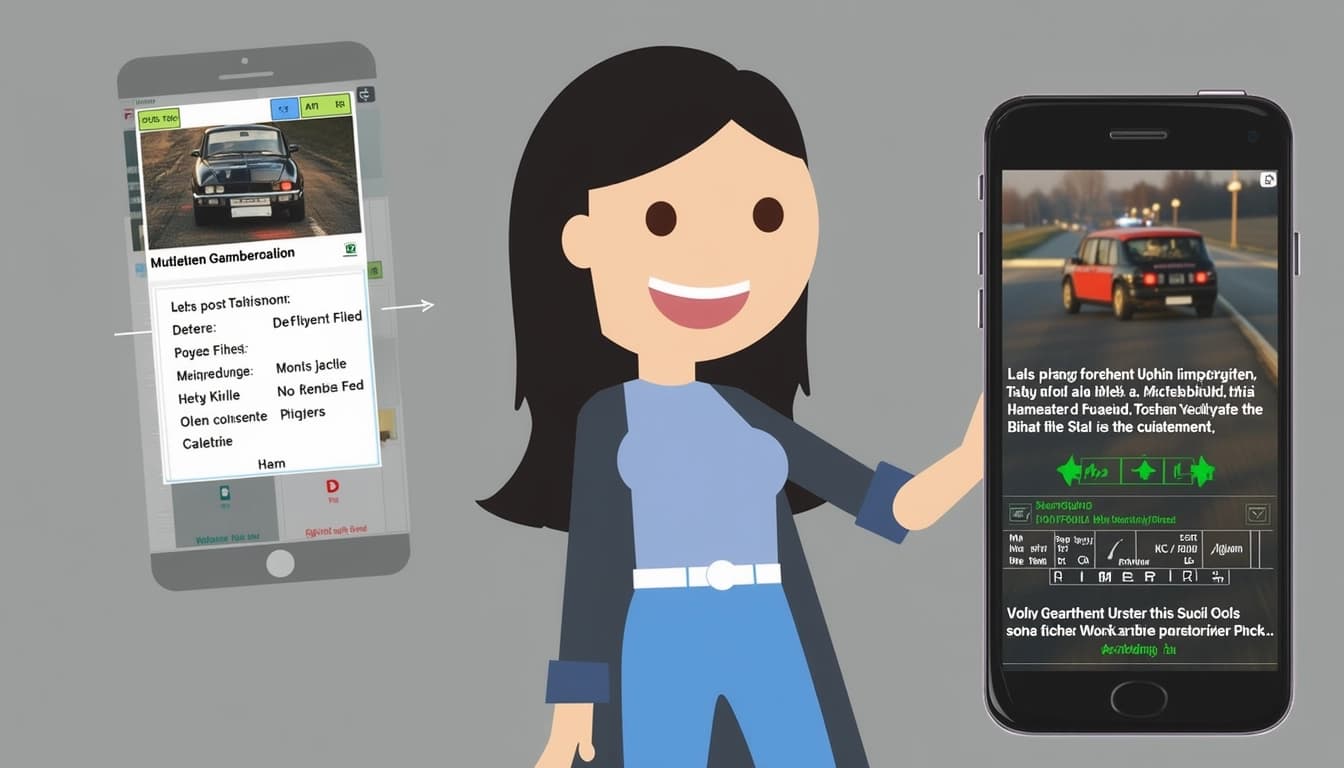
Is BLIP-3 the New Standard for Multilingual Image Captioning?
By John Doe 5 min
Is BLIP-3 the New Standard for Multilingual Image Captioning?
BLIP-3, also known as xGen-MM, is a recent advancement in the field of Large Multimodal Models (LMMs) developed by Salesforce AI Research. It builds on the success of its predecessors, BLIP and BLIP-2, aiming to enhance vision-language tasks. However, when it comes to generating image captions in multiple languages, known as multilingual image captioning, BLIP-3's capabilities are limited. This article explores whether BLIP-3 can be considered the new standard for this specific task and provides a detailed analysis of its features and limitations.
Key Points
- Research suggests BLIP-3 is not designed for multilingual image captioning, focusing mainly on English.
- It seems likely that BLIP-3 excels in multimodal tasks but lacks native support for generating captions in multiple languages.
- The evidence leans toward other models, like mBLIP, being better suited for multilingual image captioning.
Capabilities and Focus
BLIP-3 is designed to handle a variety of vision-language tasks, such as image captioning, visual question answering, and text-rich image understanding. It uses curated datasets like BLIP3-KALE for high-quality captions, BLIP3-OCR-200M for OCR annotations, and BLIP3-GROUNDING-50M for visual grounding. These datasets, however, are primarily centered around English, with annotations and evaluations conducted in English. For instance, BLIP3-OCR-200M leverages PaddleOCR, which supports over 80 languages for text detection, but the resulting captions are in English, incorporating OCR data as additional information.
This English-centric approach means BLIP-3 is not inherently built to generate captions in languages other than English. While it can process images with text in multiple languages due to its OCR capabilities, its output for image captioning remains in English. This limitation makes it less suitable as a standard for multilingual image captioning, where the goal is to produce captions in various languages directly.
BLIP-3 is an advanced multimodal model developed by Salesforce, designed to handle complex tasks involving both images and text. It builds upon previous models by integrating OCR and grounding capabilities, making it highly versatile for various applications.
Key Features of BLIP-3
BLIP-3 introduces several innovative features, including the use of large-scale datasets like BLIP3-OCR-200M and BLIP3-GROUNDING-50M. These datasets enhance the model's ability to process and understand text within images, providing more accurate and context-aware outputs.
OCR and Grounding Capabilities
The model leverages OCR annotations from PaddleOCR, which supports over 80 languages, to extract text from images. This allows BLIP-3 to generate detailed captions and perform tasks like image grounding, where it can identify and describe specific elements within an image.
Training and Architecture
BLIP-3 simplifies the training process with a unified loss function across all stages, improving efficiency. The model comes in various architectures, including base, instruction-tuned, and safety-tuned versions, ensuring robustness across different benchmarks.
Multilingual Capabilities
While BLIP-3 primarily focuses on English language tasks, its integration with PaddleOCR allows it to process text in multiple languages. However, the generated captions remain in English, which may limit its use in fully multilingual applications without additional modifications.
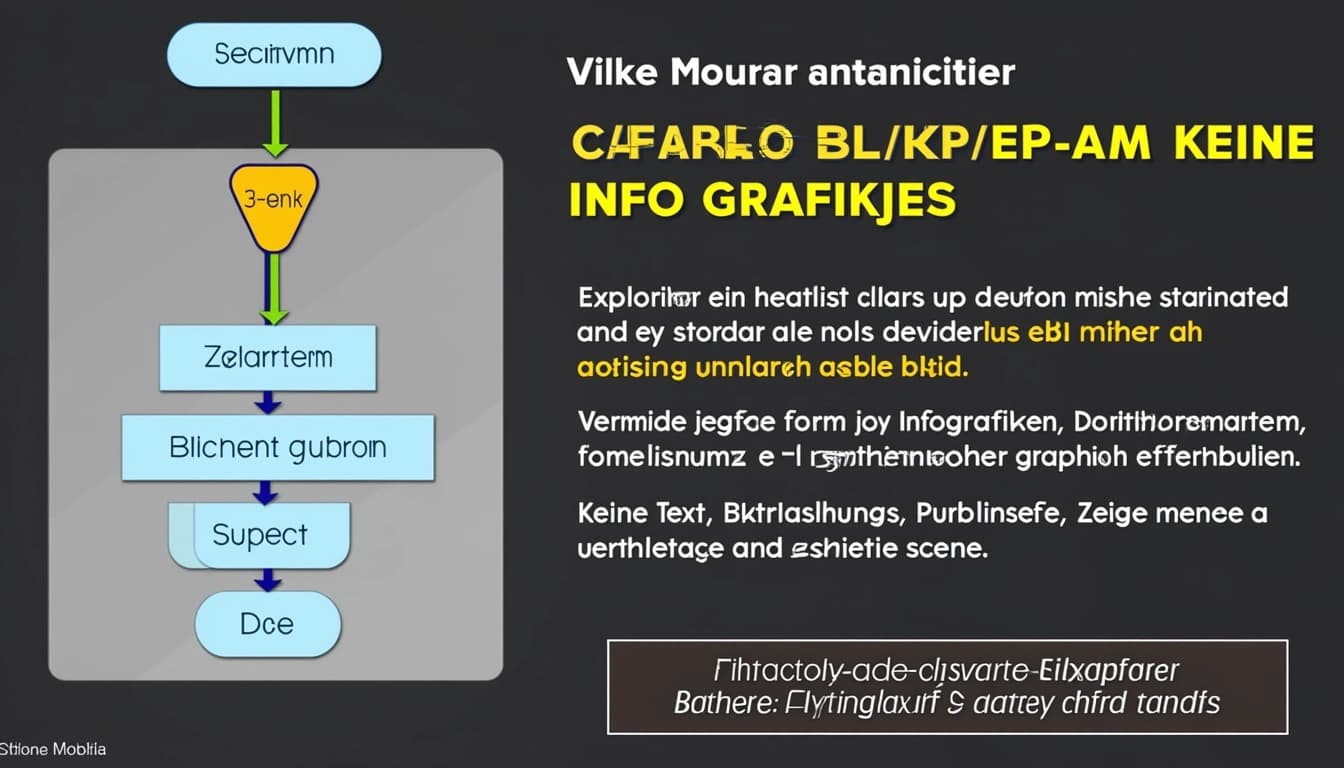
Applications and Future Directions
BLIP-3's capabilities make it suitable for a wide range of applications, from automated image captioning to complex multimodal tasks. Future developments may focus on expanding its multilingual support and enhancing its grounding accuracy for even broader use cases.
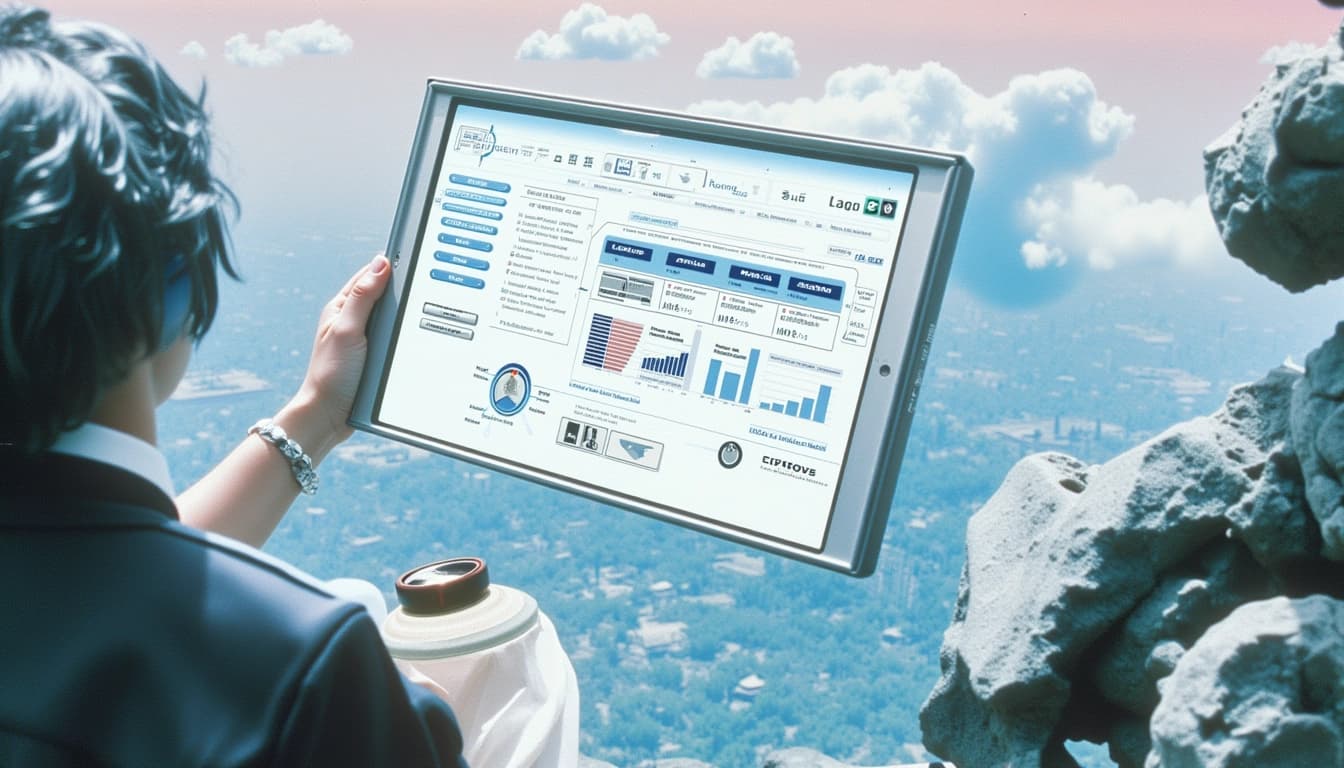
- Automated image captioning
- Multimodal task processing
- Text extraction from images
BLIP-3 is a powerful multimodal model designed for tasks like image captioning and visual question answering. It leverages advanced techniques to understand and generate text based on visual inputs, making it a versatile tool for various applications.
OCR Capabilities in BLIP-3
BLIP-3 integrates Optical Character Recognition (OCR) to detect and recognize text within images. This feature allows the model to process images containing text, such as signs or documents, and incorporate the recognized text into its outputs. The OCR functionality is built on robust frameworks like PaddleOCR, ensuring high accuracy in text detection and recognition.
Supported Languages in OCR
The OCR component in BLIP-3 supports multiple languages, including but not limited to English, Arabic, Traditional Chinese, Korean, and Japanese. This multilingual support enhances the model's ability to process diverse textual content within images, making it useful for global applications.
Multilingual Image Captioning
While BLIP-3 excels in English-based tasks, its support for multilingual image captioning is limited. The model primarily generates captions in English, even when processing images with text in other languages. This limitation stems from its training data and architecture, which are optimized for English-centric benchmarks.
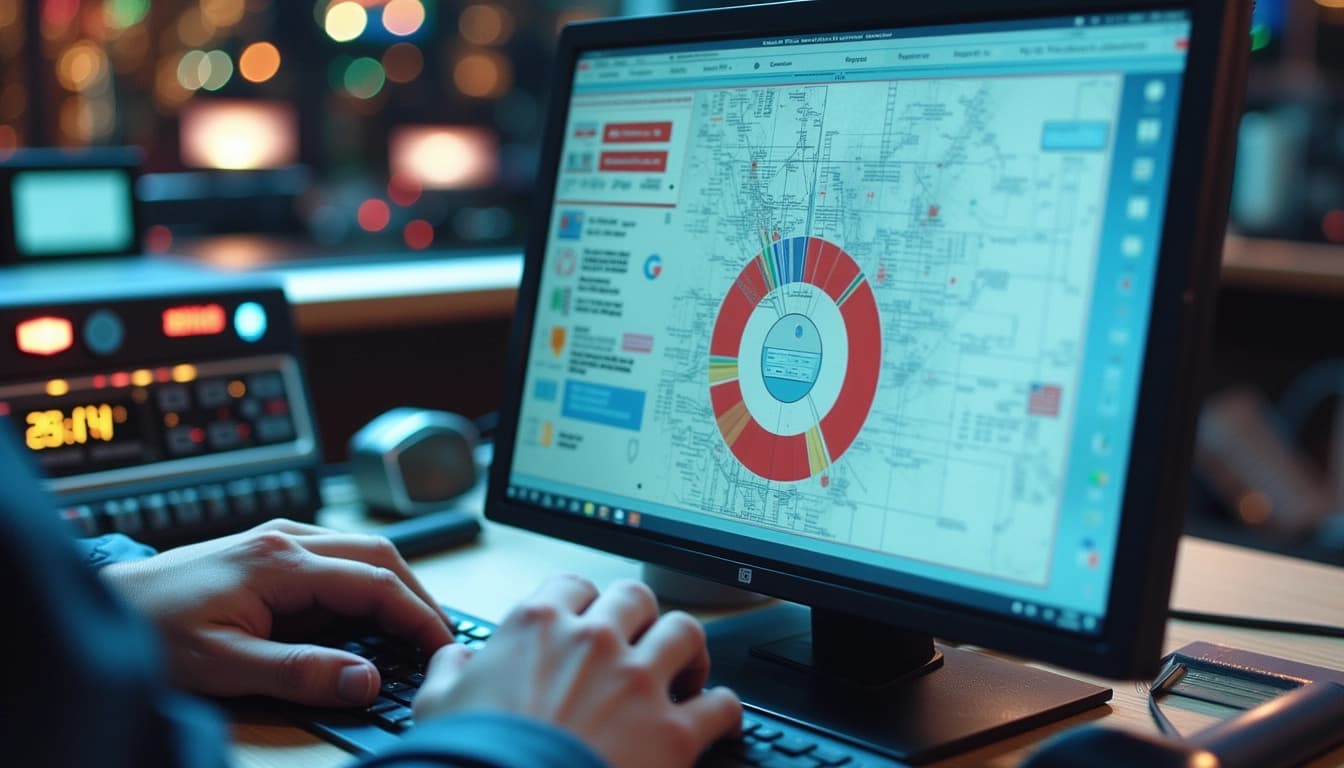
Comparison with mBLIP
Unlike BLIP-3, models like mBLIP are specifically designed for multilingual tasks. mBLIP aligns its vision and language components to a multilingual LLM, enabling it to generate captions in numerous languages. This makes mBLIP a better choice for applications requiring diverse language support.
Conclusion & Future Directions
BLIP-3 is a robust model for English-based multimodal tasks, but its multilingual capabilities are currently limited. Future developments could focus on expanding its language support to match the versatility of models like mBLIP, thereby broadening its applicability in global contexts.
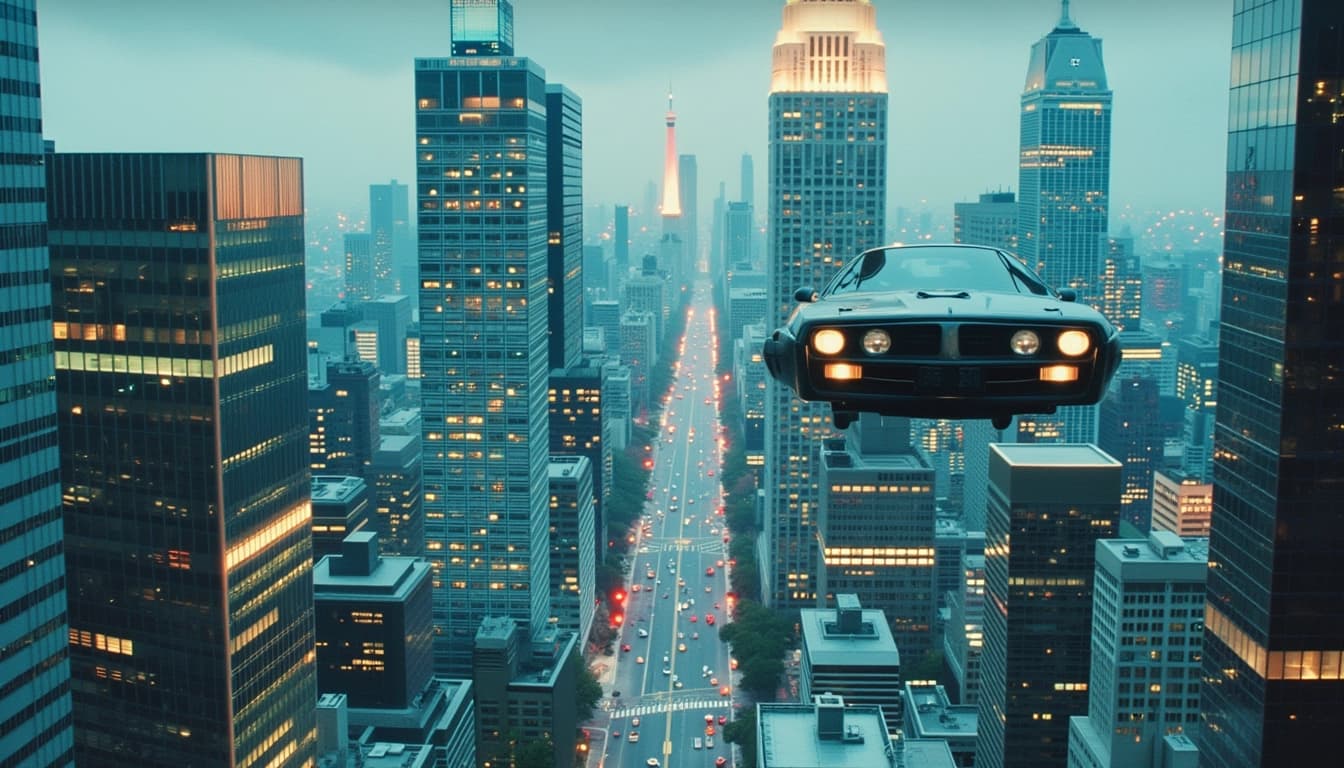
- BLIP-3 supports OCR for multiple languages
- Primary captioning output is in English
- mBLIP offers broader multilingual support
BLIP-3 is a powerful multimodal model developed by Salesforce, designed for tasks like image captioning and visual question answering. It builds on the success of previous BLIP models, incorporating advanced techniques to improve performance on English-centric datasets.
Multilingual Capabilities of BLIP-3
While BLIP-3 excels in English multimodal tasks, it does not natively support multilingual image captioning. The model is trained primarily on English datasets, such as BLIP3-OCR-200M, and its caption generation is limited to English. This makes it less suitable for applications requiring multilingual support out of the box.
Comparison with mBLIP
In contrast, mBLIP, a variant of BLIP-2, is specifically designed for multilingual image captioning. It supports over 80 languages and is trained on multilingual datasets like mBART50. This makes mBLIP a better choice for applications requiring caption generation in multiple languages.
Potential for Multilingual Adaptation
Researchers could fine-tune BLIP-3 on multilingual datasets to extend its capabilities. However, this would require additional effort, such as translating existing datasets or creating new ones. Projects like LMCap demonstrate alternative approaches using retrieval-augmented language models for few-shot multilingual captioning.
Comparative Analysis
The table below highlights the key differences between BLIP-3 and mBLIP in terms of multilingual capabilities. While BLIP-3 is robust for English tasks, mBLIP is better suited for multilingual applications.
- BLIP-3: English-centric, no native multilingual support
- mBLIP: Supports over 80 languages, trained on multilingual datasets
- BLIP-3 requires fine-tuning for multilingual use, mBLIP works out of the box
Conclusion & Next Steps
BLIP-3 is not the new standard for multilingual image captioning due to its focus on English. For multilingual applications, mBLIP or similar models are more appropriate. Future work could explore fine-tuning BLIP-3 on multilingual datasets or integrating it with retrieval-augmented approaches like LMCap.
https://arxiv.org/abs/2305.19821BLIP-3 is a family of open large multimodal models designed to handle various tasks involving images and text. It includes components for image captioning, visual question answering, and object grounding, making it versatile for different applications. The model leverages a combination of pre-trained vision and language models to achieve high performance in these tasks.
Key Features of BLIP-3
BLIP-3 stands out due to its ability to perform zero-shot tasks, meaning it can generalize to new tasks without additional training. It also includes an OCR component for extracting text from images, which is useful for applications like document analysis. The model is trained on large datasets, ensuring robust performance across diverse scenarios.
OCR Capabilities
The OCR component in BLIP-3 is particularly powerful, capable of extracting text from images with high accuracy. This feature is beneficial for tasks like digitizing printed documents or analyzing screenshots. The model's performance in this area is comparable to specialized OCR tools like PaddleOCR.
Multilingual Support
While BLIP-3 excels in English-language tasks, its support for other languages is limited compared to specialized multilingual models like mBLIP. For applications requiring outputs in multiple languages, users may need to consider alternative models. Future updates to BLIP-3 may address this limitation.
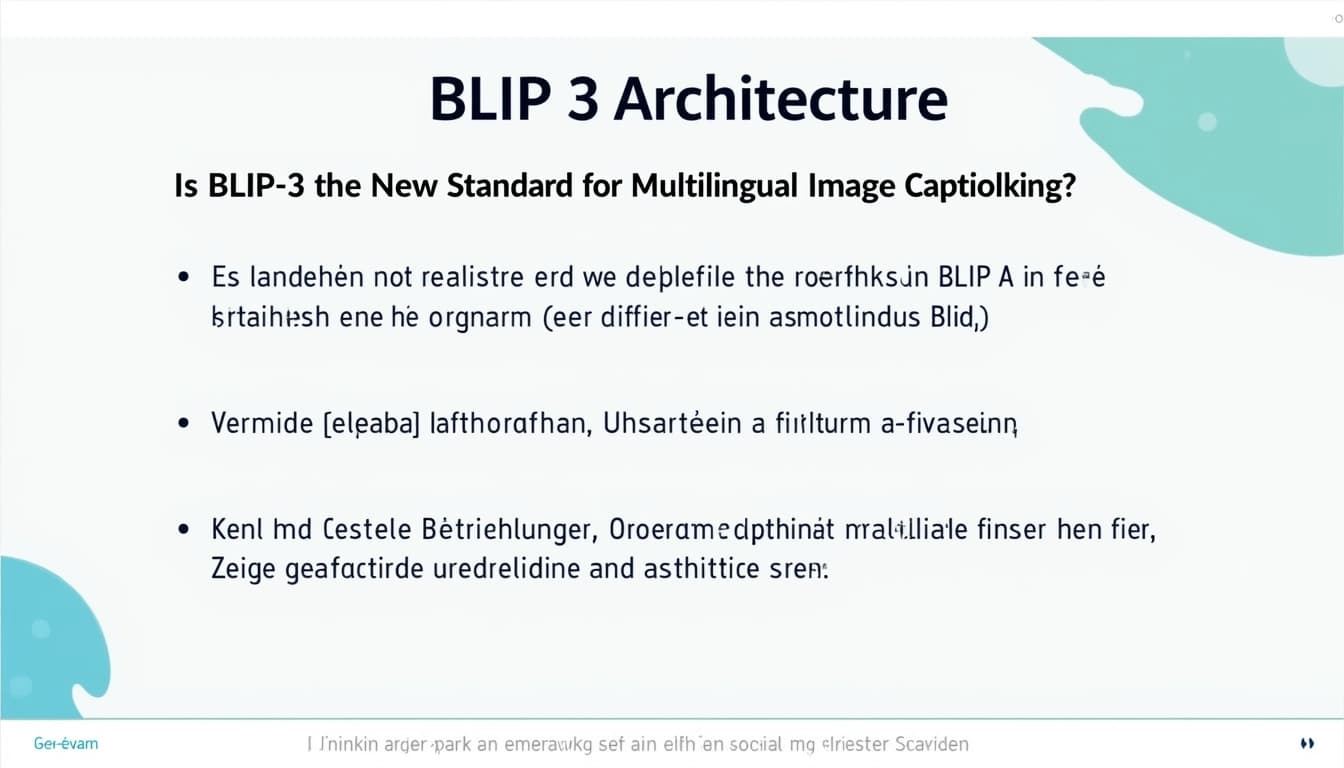
Applications and Use Cases
BLIP-3 is well-suited for a variety of applications, including automated image captioning, visual question answering, and object detection. Its versatility makes it a valuable tool for developers working on AI-driven projects. The model's open nature also allows for customization to specific needs.
Image Captioning
One of the primary uses of BLIP-3 is generating descriptive captions for images. This feature is useful for accessibility applications, such as providing alt text for visually impaired users. The model's ability to understand context ensures that the captions are accurate and meaningful.
Conclusion & Next Steps
BLIP-3 represents a significant advancement in multimodal AI, offering robust performance across a range of tasks. While it has some limitations, such as limited multilingual support, its strengths make it a compelling choice for many applications. Future developments may further enhance its capabilities.
- BLIP-3 excels in zero-shot tasks
- OCR capabilities are highly accurate
- Limited multilingual support compared to specialized models