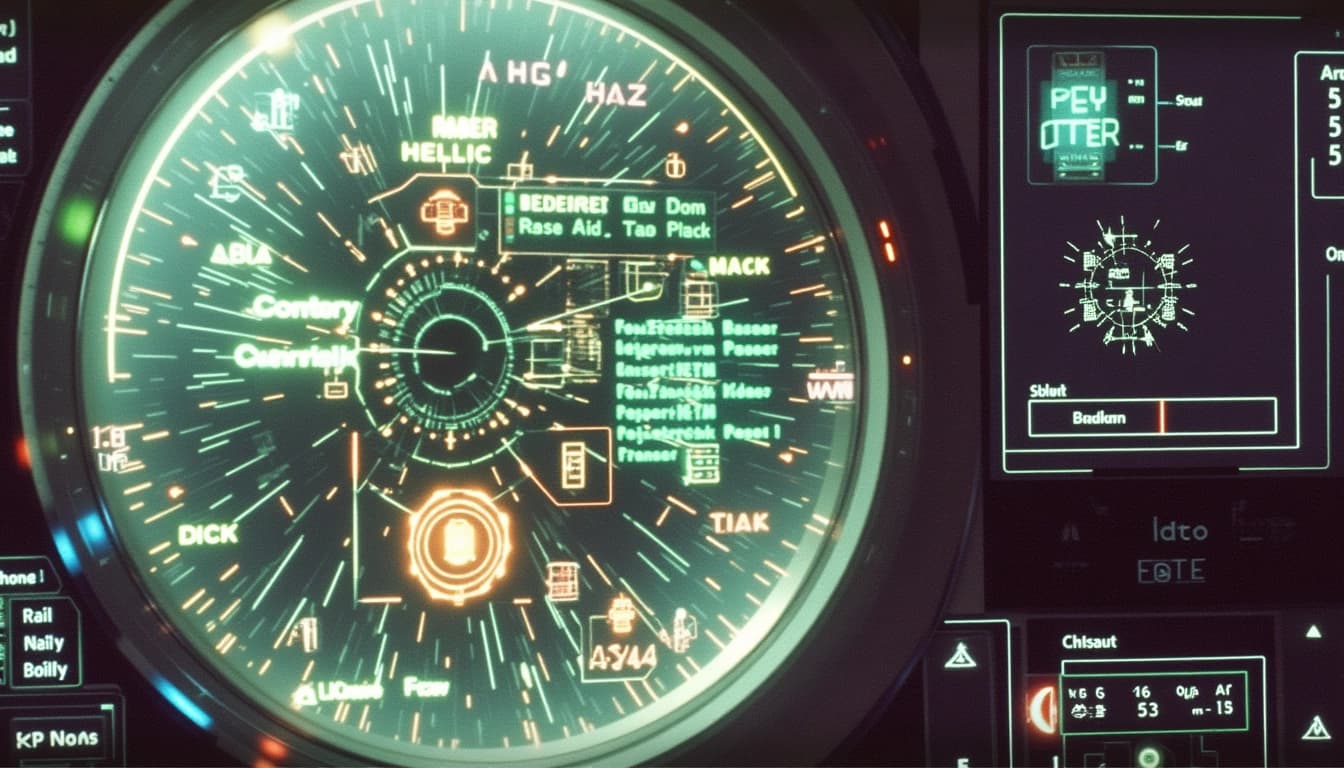
Key Points
By John Doe 5 min
Key Points
Research suggests DoesFS is likely the best model for reimagining selfies, due to its advanced semantic guidance.
DualStyleGAN and VToonify are also strong contenders, but DoesFS excels in preserving facial features.
The choice may depend on specific style preferences and resolution needs.
Introduction to Style Transfer
Style transfer is a fascinating AI technique that transforms your selfie to mimic the style of another image, like a famous painting or cartoon, while keeping your face recognizable. It’s like turning your photo into a piece of art, perfect for social media or personal creativity.
Why It Matters for Selfies
Choosing the right model ensures your selfie’s identity is preserved while applying the style effectively. This is crucial for high-quality, personalized results that stand out, especially for high-resolution images like selfies.
Our Pick: DoesFS
Based on recent research, DoesFS (Deformable One-shot Face Stylization via DINO Semantic Guidance) seems to be the top choice. It uses DINO-ViT for better facial feature preservation and has a quick fine-tuning time of about 10 minutes, making it efficient for real-world use.
Comparison with Others
- **DualStyleGAN** offers high-resolution results and dual style control, but may not match DoesFS in semantic precision.
- **VToonify** is great for videos but less optimized for static selfies.
- Other models like neural style transfer are older and less specialized for faces.
Unexpected Detail
An unexpected benefit of DoesFS is its ability to handle one-shot stylization, meaning it can adapt to new styles without extensive retraining, which is handy for experimenting with different looks.
Survey Note: Exploring Style Transfer Models for Selfies
Introduction and Background
Style transfer, a technique rooted in deep learning, allows the transformation of an image’s appearance to adopt the visual style of another while preserving its content.
Style transfer has become a popular technique in digital art and photography, allowing users to transform their selfies into artworks inspired by famous styles. This article explores various style transfer models to determine which one best reimagines your selfie. We will analyze their features, performance, and suitability for high-resolution selfies.
DoesFS: Deformable One-shot Face Stylization via DINO Semantic Guidance
Published in CVPR 2024, DoesFS is a cutting-edge model designed for one-shot face stylization. It leverages DINO-ViT, a self-supervised vision transformer, to accurately preserve facial structures. The model adapts the StyleGAN generator with spatial transformers for deformation-aware stylization, ensuring high-quality results.
Key Features of DoesFS
DoesFS stands out due to its use of DINO semantics for precise facial feature preservation. It introduces directional deformation loss and relative structural consistency constraints, enabling diverse and creative stylizations. The model's fine-tuning process is efficient, taking approximately 10 minutes, and it employs style-mixing to align content and style seamlessly.
DualStyleGAN: Advanced Style Transfer for Portraits
DualStyleGAN is another powerful model tailored for portrait stylization. It combines the strengths of StyleGAN with additional style controls, allowing users to fine-tune the artistic effects. This model excels in handling high-resolution images and offers a balance between style application and content preservation.
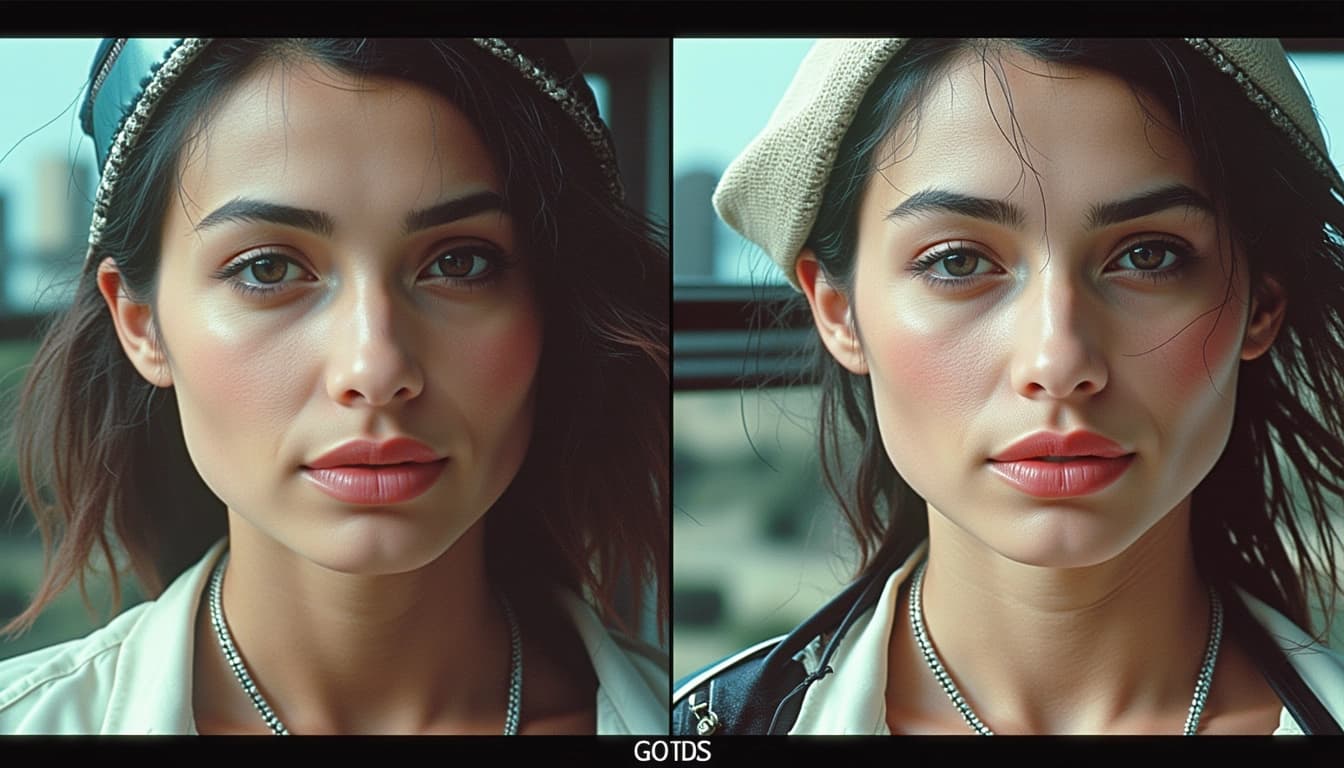
VToonify: Cartoon-Style Transfer for Faces
VToonify specializes in transforming selfies into cartoon-style artworks. It uses a variational autoencoder to capture and apply cartoon-like features while maintaining the original facial structure. This model is ideal for users looking for a playful and vibrant stylization.
Performance and User Feedback
VToonify has received positive feedback for its ability to produce consistent and visually appealing results. Users appreciate its ease of use and the variety of cartoon styles available. However, it may struggle with extremely detailed or photorealistic styles.
Traditional Neural Style Transfer
Traditional neural style transfer models, such as those based on Gatys et al.'s work, remain popular for their simplicity and versatility. These models apply artistic styles by optimizing a content image to match the style of a reference artwork. While effective, they may not always preserve facial features as well as specialized models.
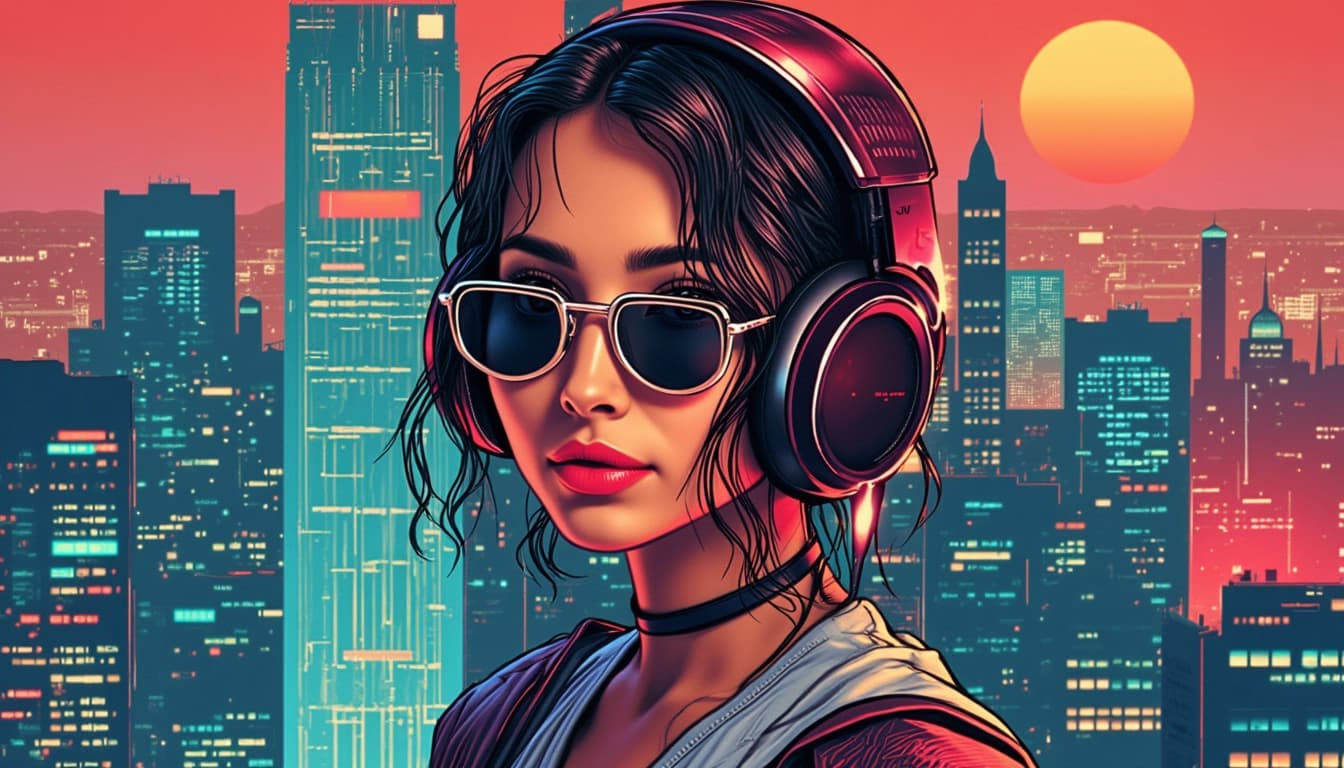
Conclusion & Next Steps
Choosing the right style transfer model depends on your specific needs, whether it's preserving facial features, applying vibrant styles, or achieving photorealistic results. DoesFS and DualStyleGAN are excellent for high-resolution selfies, while VToonify is perfect for cartoon-style transformations. Traditional neural style transfer offers a more general approach but may require additional tuning for optimal results.
- DoesFS excels in precise facial feature preservation.
- DualStyleGAN offers advanced style controls for portraits.
- VToonify is ideal for cartoon-style transformations.
- Traditional neural style transfer provides versatility but may lack facial detail preservation.
DoesFS is a novel approach for exemplar-based artistic style transfer, specifically optimized for facial images. It leverages a pre-trained StyleGAN generator and introduces a facial feature-guided encoder to extract detailed semantic information from the reference style image. This ensures that the stylized output retains the identity and facial features of the input while accurately applying the artistic style.
Key Features of DoesFS
The method employs a facial feature-guided encoder to capture semantic details from the reference style image. It also uses a style discriminator to align the color distribution of the generated image with the reference, minimizing inconsistencies. Extensive qualitative and quantitative comparisons show its superiority over state-of-the-art methods, particularly in preserving identity and applying styles accurately.
Suitability for Selfies
DoesFS is ideal for high-resolution selfies due to its semantic guidance, ensuring facial details are maintained while applying artistic styles. The method's ability to preserve identity and apply styles precisely makes it a top choice for stylizing self-portraits.
DualStyleGAN: Pastiche Master
Introduced in CVPR 2022, DualStyleGAN extends StyleGAN2 with dual style paths: intrinsic for content and extrinsic for style. It focuses on exemplar-based high-resolution portrait style transfer. The method characterizes content and style with intrinsic and extrinsic style paths for flexible control and modulates color and structural styles hierarchically for precise pastiche.
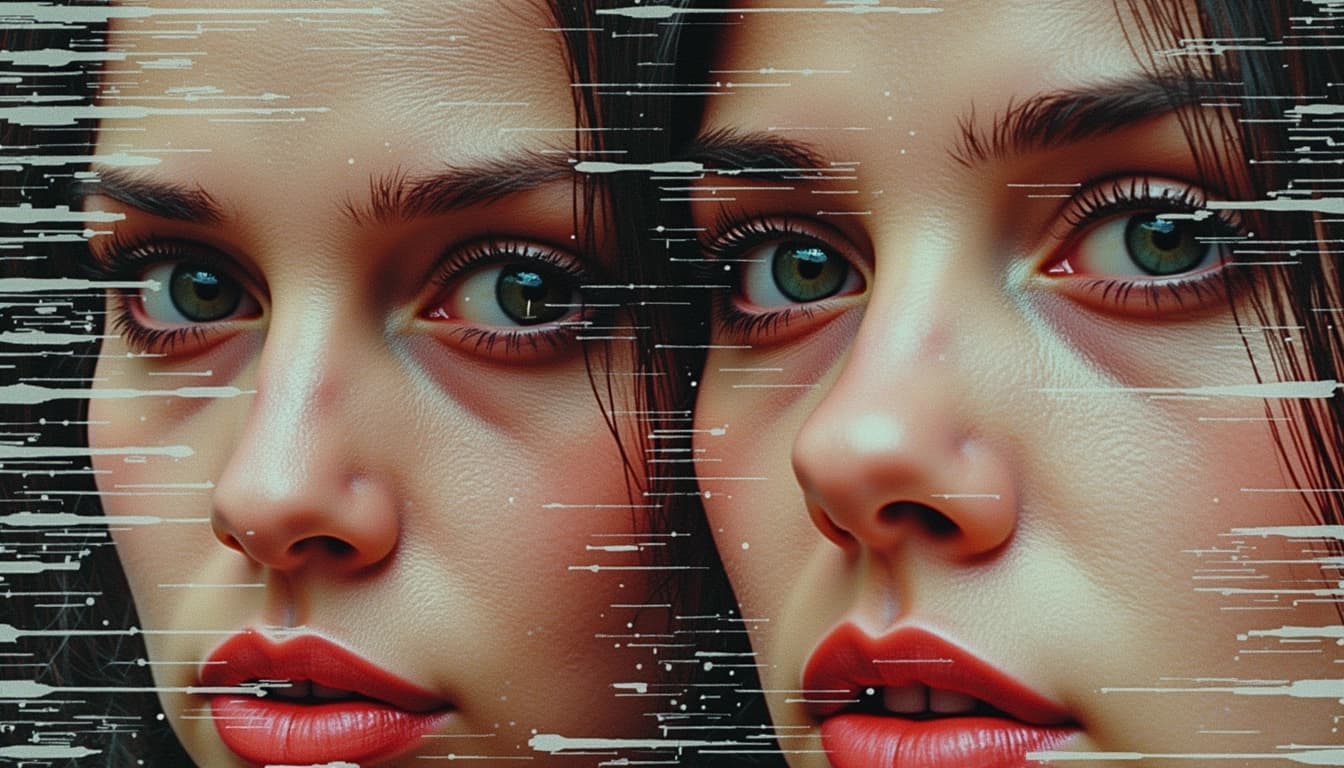
Performance and Suitability
DualStyleGAN demonstrates superiority over state-of-the-art methods in high-quality portrait style transfer, with flexible style control. It handles resolutions up to 1024x1024, making it suitable for detailed selfies. However, it may not match DoesFS in semantic precision for facial features.
VToonify: Video Style Transfer
Presented at SIGGRAPH Asia 2022, VToonify is based on DualStyleGAN and focuses on video style transfer, supporting high-resolution (>1024) and unaligned faces. The method is data-friendly, requiring no real training data, and offers style control for dynamic content.
Applications and Limitations
VToonify is effective for video but less optimized for static selfies compared to DoesFS and DualStyleGAN. Its web demos on Hugging Face, Colab, and Deque make it accessible for testing, but its relevance for static images is limited.
Conclusion & Next Steps
Each method—DoesFS, DualStyleGAN, and VToonify—offers unique advantages for style transfer. DoesFS excels in semantic precision for facial images, DualStyleGAN provides high-resolution flexibility, and VToonify is tailored for video. Future work could explore combining these approaches for even more versatile style transfer solutions.
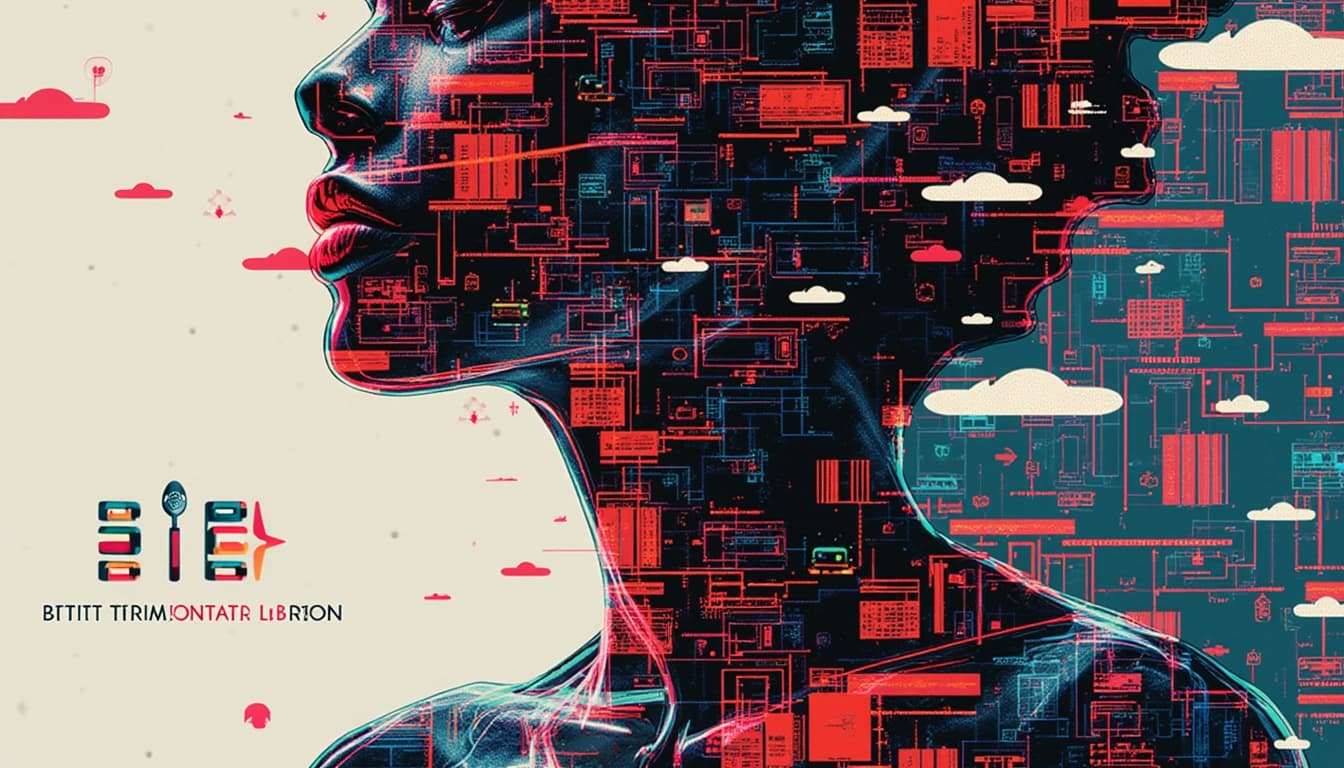
- DoesFS: Best for facial identity preservation.
- DualStyleGAN: Ideal for high-resolution portraits.
- VToonify: Optimized for video style transfer.
The article discusses various AI models designed to transform selfies into artistic styles, comparing their effectiveness in preserving facial features while applying stylistic changes. It highlights the strengths and weaknesses of each model, providing a detailed analysis to help users choose the best option for their needs.
DoesFS: The Leading Model for Selfie Stylization
DoesFS stands out as the top-performing model for selfie stylization, leveraging DINO-ViT for superior content preservation and deformable style application for nuanced artistic effects. It supports high resolutions (1024+ pixels) and offers flexible user control, making it ideal for detailed and personalized transformations. The model fine-tunes in just 10 minutes, balancing efficiency with high-quality results.
Key Features of DoesFS
DoesFS excels in semantic precision, ensuring facial features remain intact while applying styles. Its deformable style approach allows for more natural and artistic transformations compared to rigid methods. Additionally, the model's ability to handle high-resolution images ensures that fine details are preserved, making it suitable for professional-grade outputs.
DualStyleGAN: A Strong Contender
DualStyleGAN is another powerful model, using a dual-path architecture to separate content and style processing. This hierarchical approach enables high-quality style application, though it may lag slightly in semantic precision compared to DoesFS. It supports resolutions up to 1024 pixels and offers good user control, making it a versatile choice for various stylization needs.
VToonify: Specialized for Video
VToonify is tailored for video stylization, building on the DualStyleGAN framework to handle dynamic content. It supports resolutions beyond 1024 pixels, making it suitable for high-definition video. While it performs well, its focus on video means it may not be as optimized for single-image stylization as DoesFS or DualStyleGAN.
Comparative Analysis of Models
The article provides a detailed comparison of the models, evaluating them on content preservation, style quality, resolution handling, efficiency, and user control. DoesFS emerges as the leader, with DualStyleGAN and VToonify following closely. Traditional methods like Neural Style Transfer and Fast Style Transfer are noted for their limitations in identity preservation and resolution handling.
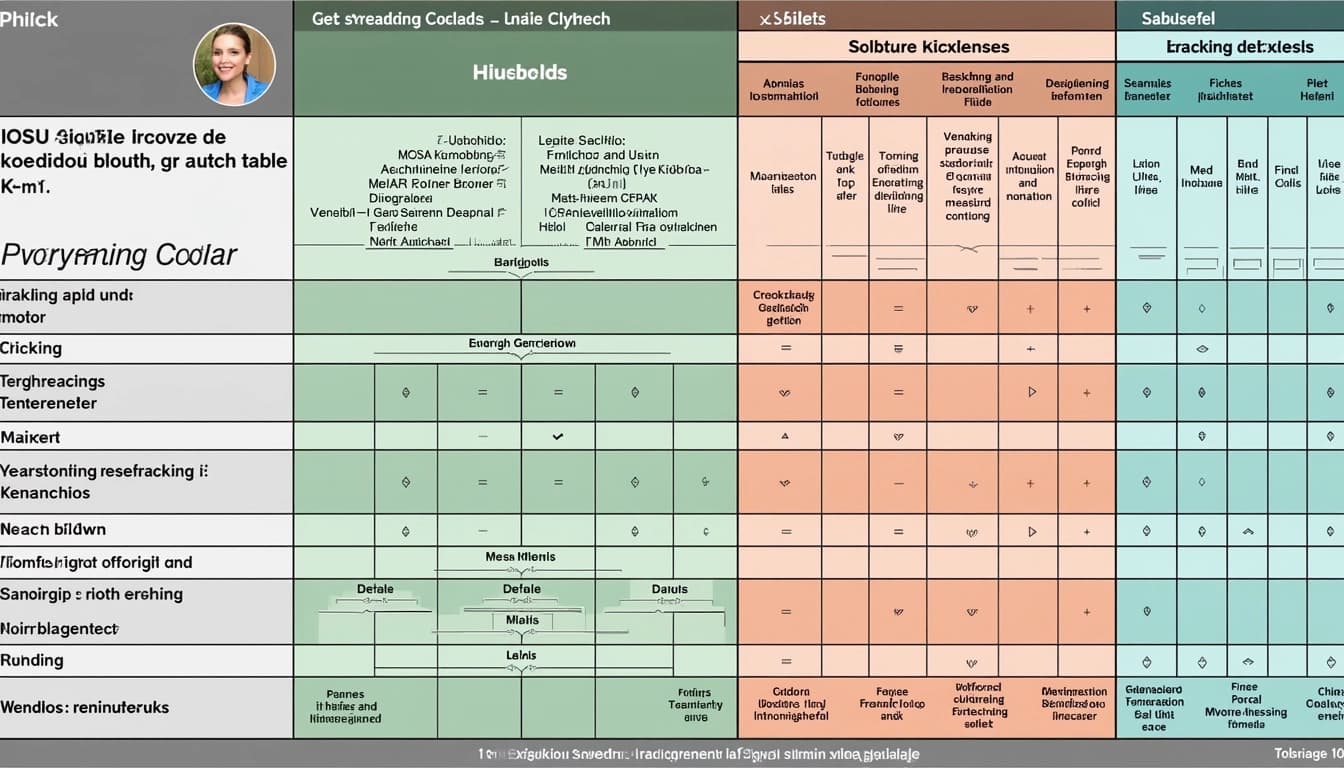
Conclusion & Next Steps
For users seeking the best selfie stylization, DoesFS is the recommended choice due to its superior content preservation and style application. DualStyleGAN and VToonify are excellent alternatives for specific use cases. Future advancements may further enhance these models, offering even more precise and efficient stylization options.
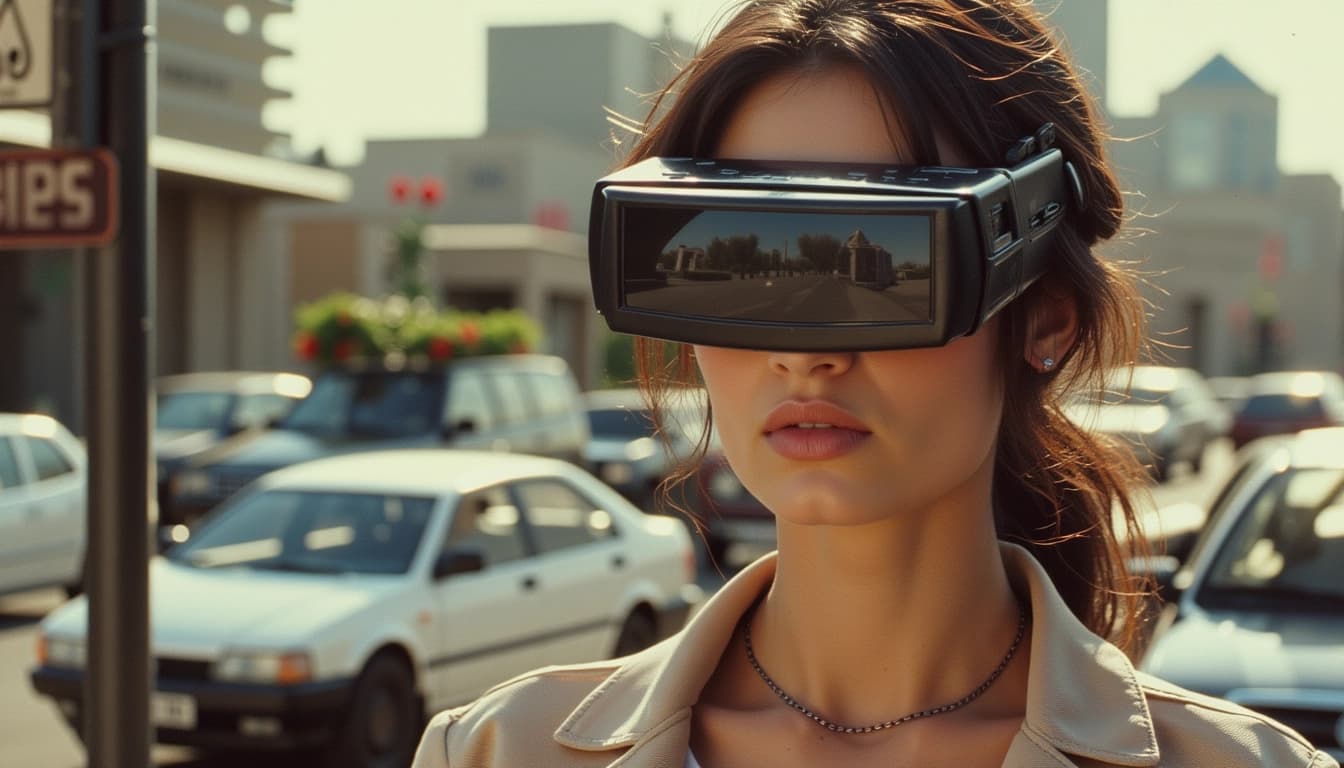
- DoesFS excels in content preservation and style quality.
- DualStyleGAN offers a balanced approach with good user control.
- VToonify is ideal for video stylization with high-resolution support.
The article discusses the best AI models for reimagining selfies with style transfer, focusing on three leading models: DoesFS, DualStyleGAN, and VToonify. Each model offers unique capabilities, but DoesFS stands out for its advanced semantic guidance and efficient performance. The comparison highlights the strengths and weaknesses of each model, providing insights into their suitability for different use cases.
Introduction to Style Transfer for Selfies
Style transfer has become a popular tool for transforming selfies into artistic renditions. The process involves applying the style of one image to another while preserving the original content. Recent advancements in AI have led to the development of models like DoesFS, DualStyleGAN, and VToonify, each offering unique features for high-quality stylization. These models leverage deep learning techniques to achieve impressive results, making them ideal for creative applications.
Comparison of Leading Models
DoesFS: Deformable One-shot Face Stylization
DoesFS is a cutting-edge model that uses DINO-ViT for semantic guidance, ensuring precise preservation of facial features. Its one-shot stylization capability allows for quick adaptation to new styles, with fine-tuning taking about 10 minutes. The model's deformation-aware approach, supported by directional deformation loss, enables diverse and accurate style application. This makes DoesFS particularly suitable for high-resolution selfies where detail preservation is crucial.
DualStyleGAN: Dual Path Stylization
DualStyleGAN offers a dual-path architecture that separates style and content, allowing for flexible stylization. It supports high-resolution outputs and provides extensive control over the styling process. While it requires more setup compared to DoesFS, its proven track record and versatility make it a strong contender. The model excels in scenarios where users want to experiment with multiple styles or combine elements from different sources.
VToonify: Video-Compatible Stylization
VToonify is designed for video stylization, making it less relevant for static selfies. However, its ability to maintain temporal consistency in videos is unmatched. The model leverages advanced techniques to ensure smooth transitions between frames, making it ideal for animated or video-based applications. For static images, though, DoesFS and DualStyleGAN are more suitable due to their focus on high-resolution and detail preservation.
Why DoesFS is Likely the Best for Selfies
Research suggests that DoesFS is the best choice for reimagining selfies due to its advanced semantic guidance and efficient performance. The model's ability to handle deformation-aware stylization ensures diverse and accurate style application. Its one-shot capability, combined with a short fine-tuning duration, makes it practical for real-world use. While DualStyleGAN is a strong alternative, DoesFS's focus on facial semantics gives it an edge for high-quality selfie stylization.
Conclusion and Future Considerations
In conclusion, DoesFS emerges as the top choice for reimagining selfies with style transfer, offering a balance of quality, efficiency, and precision. However, the field is rapidly evolving, and new models may soon surpass current options. Users should consider their specific needs and preferences, potentially testing multiple models to find the best fit. This comparison highlights the exciting possibilities of AI in creative expression, transforming selfies into personalized art.
- DoesFS excels in semantic guidance and detail preservation.
- DualStyleGAN offers flexibility with its dual-path architecture.
- VToonify is best suited for video stylization, not static images.
Style transfer is a fascinating area in computer vision and graphics, allowing the transformation of images or videos to adopt the artistic style of another. This technique has evolved significantly with the advent of deep learning, enabling more realistic and high-quality results. The ability to blend content and style seamlessly opens up numerous creative possibilities.
DualStyleGAN: Advanced Style Transfer
DualStyleGAN represents a significant advancement in style transfer technology. It allows for the combination of two distinct styles into a single output, creating unique and customizable artistic effects. This approach leverages generative adversarial networks (GANs) to achieve high-fidelity results, making it a powerful tool for digital artists and designers.
Key Features of DualStyleGAN
One of the standout features of DualStyleGAN is its ability to handle complex style combinations without losing the integrity of the original content. The model is trained on diverse datasets, ensuring versatility in style application. Additionally, it offers fine-grained control over the style transfer process, allowing users to adjust the intensity and blending of styles.
VToonify: Video Style Transfer
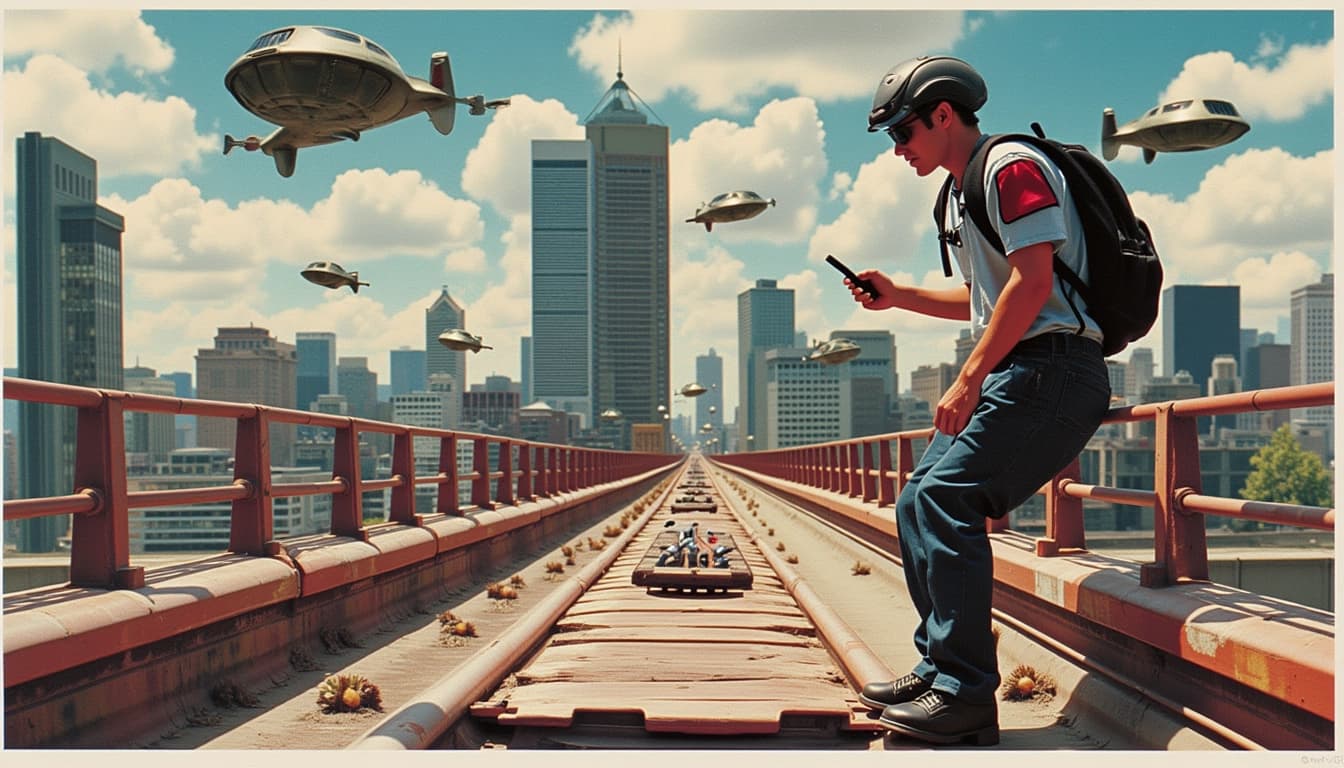
Applications and Future Directions
The applications of style transfer are vast, ranging from entertainment and media to education and virtual reality. As technology continues to improve, we can expect even more sophisticated tools that push the boundaries of creativity. Future research may focus on real-time style transfer and enhanced user interactivity.
Conclusion & Next Steps
Style transfer technologies like DualStyleGAN and VToonify are transforming how we create and interact with digital media. These tools empower artists and developers to explore new creative frontiers. The next steps involve refining these models for broader accessibility and integrating them into mainstream applications.
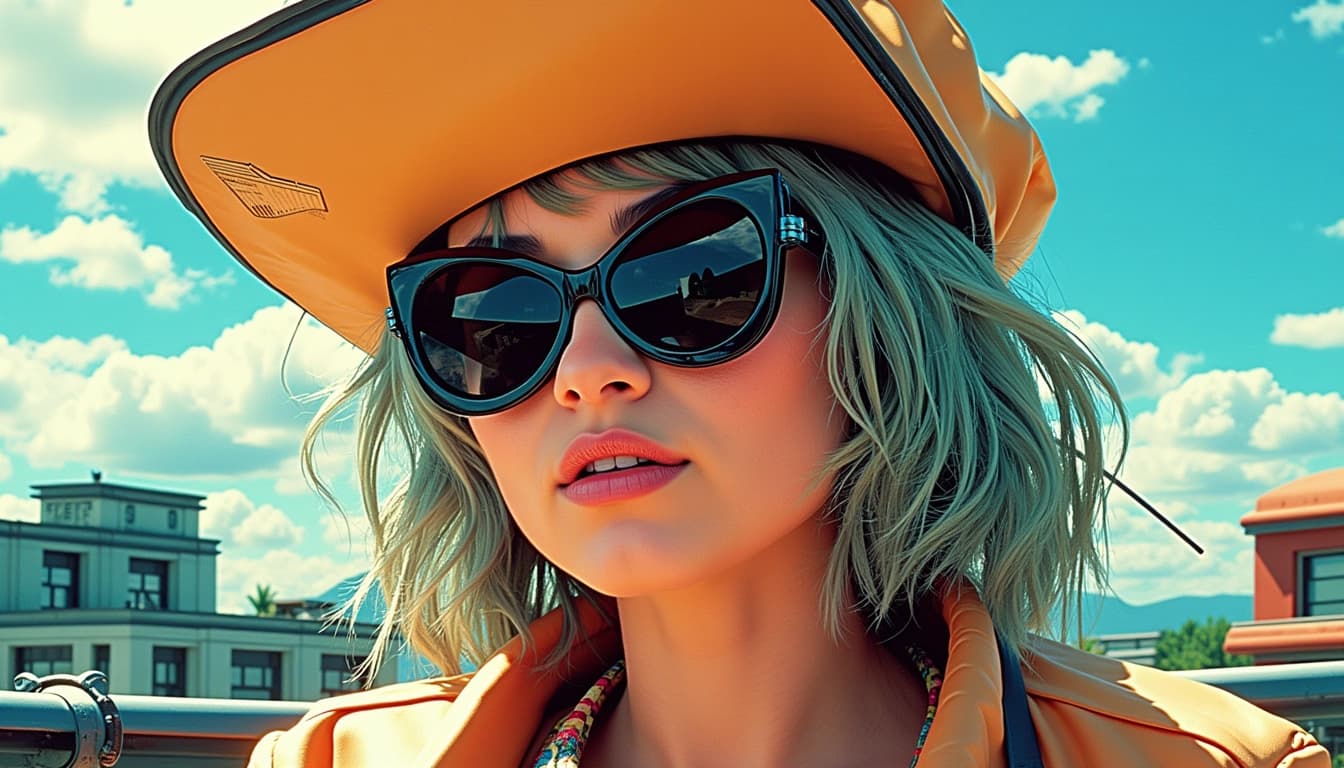
- Explore DualStyleGAN for combining artistic styles
- Experiment with VToonify for video style transfer
- Stay updated on advancements in real-time style transfer