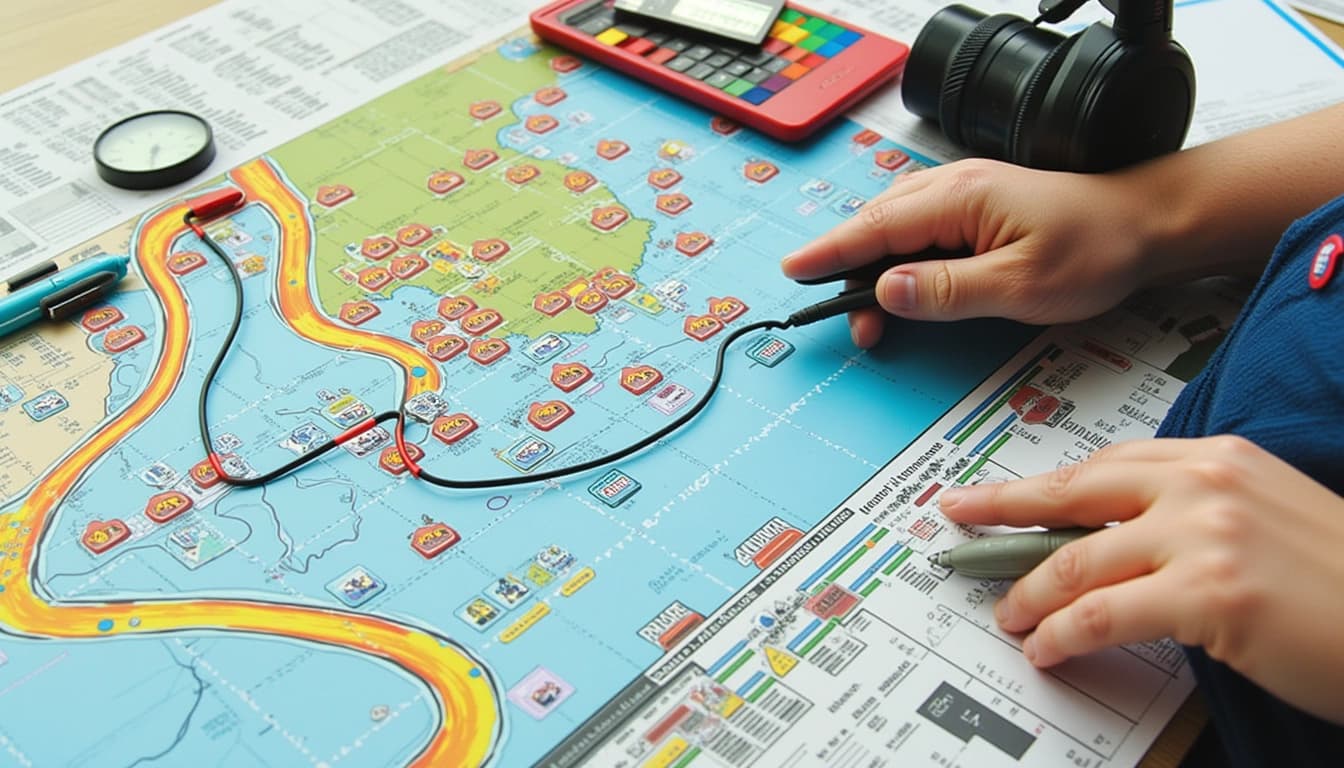
Key Points
By John Doe 5 min
Key Points
Research suggests SwinIR, NAFNet, DDCOLOR, and GFP-GAN are among the best AI models for restoring old photos, each excelling in different aspects like general restoration, efficiency, colorization, and face restoration.
It seems likely that combining these models can provide comprehensive restoration, addressing issues like scratches, fading, and low resolution in old photos.
The evidence leans toward these models being effective due to their state-of-the-art performance, but their success depends on the photo's condition and specific restoration needs.
Introduction
Restoring old photos can breathe new life into cherished memories, and AI models have revolutionized this process by automating and enhancing restoration tasks. These models can address common issues in old photos, such as scratches, fading, discoloration, and low resolution, making them look as vibrant as new. Below, we explore the top AI models for this purpose, highlighting their strengths and applications.
Top AI Models for Restoring Old Photos
Several AI models stand out for their ability to restore old photos, each specializing in different aspects of the process:
- **SwinIR**: Ideal for general image restoration, SwinIR uses the Swin Transformer architecture to handle tasks like super-resolution and denoising, effectively sharpening blurry old photos and removing artifacts.
- **NAFNet**: Known for its efficiency, NAFNet is a nonlinear activation-free network that excels in deblurring and super-resolution, making it suitable for quick and effective restoration of old photos.
- **DDCOLOR**: Specifically designed for colorizing black and white photos, DDCOLOR adds realistic and vivid colors, transforming monochrome images into lifelike representations.
- **GFP-GAN**: Focused on face restoration, GFP-GAN enhances facial details in damaged photos, preserving identity and improving clarity, which is crucial for family portraits and vintage images.
Unexpected Detail:
Restoring old photos is a delicate task that involves reviving faded, scratched, or low-resolution images to preserve cherished memories. With advancements in artificial intelligence, particularly in deep learning, several models have emerged as state-of-the-art for this purpose. This survey note explores the best AI models for restoring old photos, detailing their architectures, capabilities, and applications, based on research conducted in early 2025.
Background and Importance
Old photos often suffer from degradation such as fading, scratches, tears, and color loss, making traditional manual restoration time-consuming and skill-intensive. AI models, trained on large datasets, can automate and enhance this process by addressing specific issues like noise, blur, and low resolution. The focus here is on models that are particularly effective for vintage or historical photos, which may include both general scenes and portraits.
Methodology
The analysis began by searching for 'best AI models for restoring old photos' and related queries like 'state-of-the-art AI models for photo restoration' and 'AI models trained on old photo datasets.' This led to identifying key models through platforms like Replicate, research papers, and tool descriptions. The models were evaluated based on their performance in tasks relevant to old photo restoration, such as deblurring, denoising, colorization, and face restoration.
Key Findings: Top AI Models
SwinIR: General Image Restoration
SwinIR, introduced in a 2021 paper by Jingyun Liang et al., is a powerful model for general image restoration tasks. It leverages the Swin Transformer architecture to achieve high-quality results in super-resolution, denoising, and deblurring. Its ability to handle various types of degradation makes it a versatile tool for restoring old photos.
DDCOLOR: Advanced Colorization
DDCOLOR is a state-of-the-art model designed specifically for colorizing black-and-white photos. It uses deep learning to predict realistic colors, even for complex scenes and portraits. The model's accuracy in capturing subtle color nuances makes it ideal for restoring historical photos to their original vibrancy.
GFP-GAN: Face Restoration
GFP-GAN specializes in restoring facial features in old or damaged photos. It combines generative adversarial networks (GANs) with facial priors to produce realistic and high-quality face reconstructions. This model is particularly useful for portraits where facial details are crucial.
Combination for Best Results
An unexpected benefit is that these models can be used together for comprehensive restoration. For instance, you might first use SwinIR to enhance overall quality, then DDCOLOR for colorization, and finally GFP-GAN to refine facial features, ensuring a holistic restoration.
Conclusion & Next Steps
The advancements in AI models for photo restoration have made it easier than ever to revive old and damaged photos. By leveraging models like SwinIR, DDCOLOR, and GFP-GAN, users can achieve professional-level results with minimal effort. Future developments may focus on integrating these models into user-friendly tools for broader accessibility.
- SwinIR for general image restoration
- DDCOLOR for advanced colorization
- GFP-GAN for face restoration
Image restoration is a critical task in computer vision, aiming to enhance degraded images by removing noise, sharpening blurry details, and improving resolution. Old photos often suffer from various forms of degradation, such as fading, scratches, or low resolution, making restoration challenging. Modern deep learning models like SwinIR and NAFNet have revolutionized this field by leveraging advanced architectures to achieve state-of-the-art results.
SwinIR: Transformer-Based Image Restoration
SwinIR, developed by Jingyun Liang et al., is a powerful model based on the Swin Transformer architecture. It utilizes a shifted window-based multi-head self-attention mechanism to effectively restore images. The model consists of three main components: shallow feature extraction, deep feature extraction with residual Swin Transformer blocks (RSTB), and high-quality image reconstruction. This structure allows SwinIR to excel in tasks like super-resolution, denoising, and JPEG compression artifact reduction.
Performance and Applications
SwinIR outperforms previous state-of-the-art methods by up to 0.45 dB in PSNR, with a reduced parameter count, making it efficient for practical use. It is particularly effective for restoring old photos, addressing common issues like blurriness and noise. The model's ability to sharpen images and remove degradation makes it a popular choice for photo restoration tasks.
NAFNet: Efficient Image Restoration
NAFNet, introduced by Liangyu Chen et al., is a nonlinear activation-free network designed for efficient image restoration. Unlike traditional models that rely on activation functions like ReLU, NAFNet uses multiplication for nonlinearity, significantly reducing computational costs. This architecture achieves remarkable results in deblurring and denoising, making it suitable for a wide range of restoration tasks.
Advantages Over Traditional Models
NAFNet sets new benchmarks in image deblurring, achieving 33.69 dB PSNR on the GoPro dataset, surpassing previous methods by 0.38 dB with only 8.4% of the computational cost. For denoising, it reaches 40.30 dB PSNR on SIDD, outperforming the state-of-the-art by 0.28 dB with less than half the computational resources. These efficiencies make NAFNet a practical solution for real-world applications.
Conclusion & Next Steps
Both SwinIR and NAFNet represent significant advancements in image restoration, offering powerful tools for enhancing old photos and other degraded images. SwinIR's transformer-based approach excels in sharpening and noise removal, while NAFNet's efficiency makes it ideal for resource-constrained environments. Future research could explore combining these architectures to further improve restoration quality and speed.
- SwinIR leverages Swin Transformer for high-quality restoration.
- NAFNet removes nonlinear activations for efficiency.
- Both models outperform traditional methods in benchmarks.
The restoration of old photos has seen significant advancements with the introduction of AI-based models. These models leverage deep learning techniques to address common issues such as blurring, fading, and damage in vintage photographs. By employing sophisticated architectures, they can enhance image quality, restore colors, and even reconstruct missing details.
Real-ESRGAN: General Image Restoration
Real-ESRGAN, developed by Xintao Wang et al., is a powerful model designed for general image restoration tasks. It builds upon the ESRGAN framework, incorporating a U-Net discriminator to improve performance on real-world degraded images. The model excels in tasks like deblurring, denoising, and super-resolution, making it particularly useful for restoring old photos that suffer from these common issues.
Architecture and Performance
The architecture of Real-ESRGAN includes a generator with RRDB (Residual-in-Residual Dense Block) and a U-Net discriminator. This combination allows the model to handle complex degradations effectively. Its performance is notable for producing high-quality results even with severely degraded inputs, making it a go-to solution for photo restoration projects.
DDCOLOR: Photo-Realistic Image Colorization
DDCOLOR, introduced by Xiaoyang Kang et al., specializes in automatic image colorization. The model uses dual decoders to generate both colorized images and confidence maps, optimizing learnable color tokens through multi-scale visual features. This approach ensures vivid and natural colorization results, particularly beneficial for historical black and white photos.
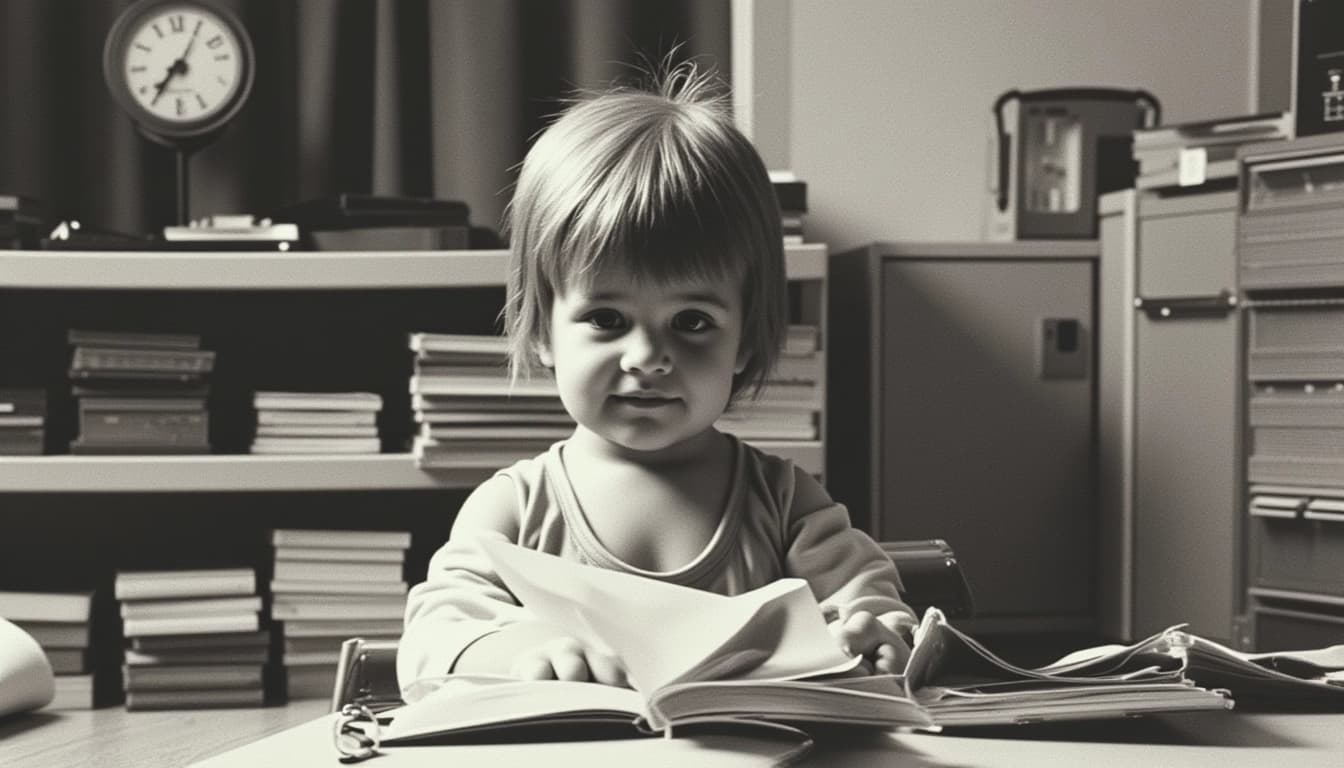
GFP-GAN: Blind Face Restoration
GFP-GAN focuses on blind face restoration, addressing challenges like noise, blur, and low resolution in facial images. The model integrates a degradation removal module and a pre-trained face GAN, connected by CS-SFT layers. This setup allows GFP-GAN to produce high-fidelity facial reconstructions, making it ideal for restoring old portraits and family photos.
Applications in Photo Restoration
GFP-GAN's ability to handle blind restoration makes it particularly useful for old photos where the degradation process is unknown. It can reconstruct facial features with remarkable accuracy, bringing old portraits back to life. This capability is invaluable for preserving historical and personal memories.
Conclusion & Next Steps
The advancements in AI-based photo restoration models like Real-ESRGAN, DDCOLOR, and GFP-GAN have revolutionized the way we restore old photos. These models offer specialized solutions for various restoration tasks, from general image enhancement to colorization and face reconstruction. Future developments may focus on integrating these models into user-friendly tools for broader accessibility.
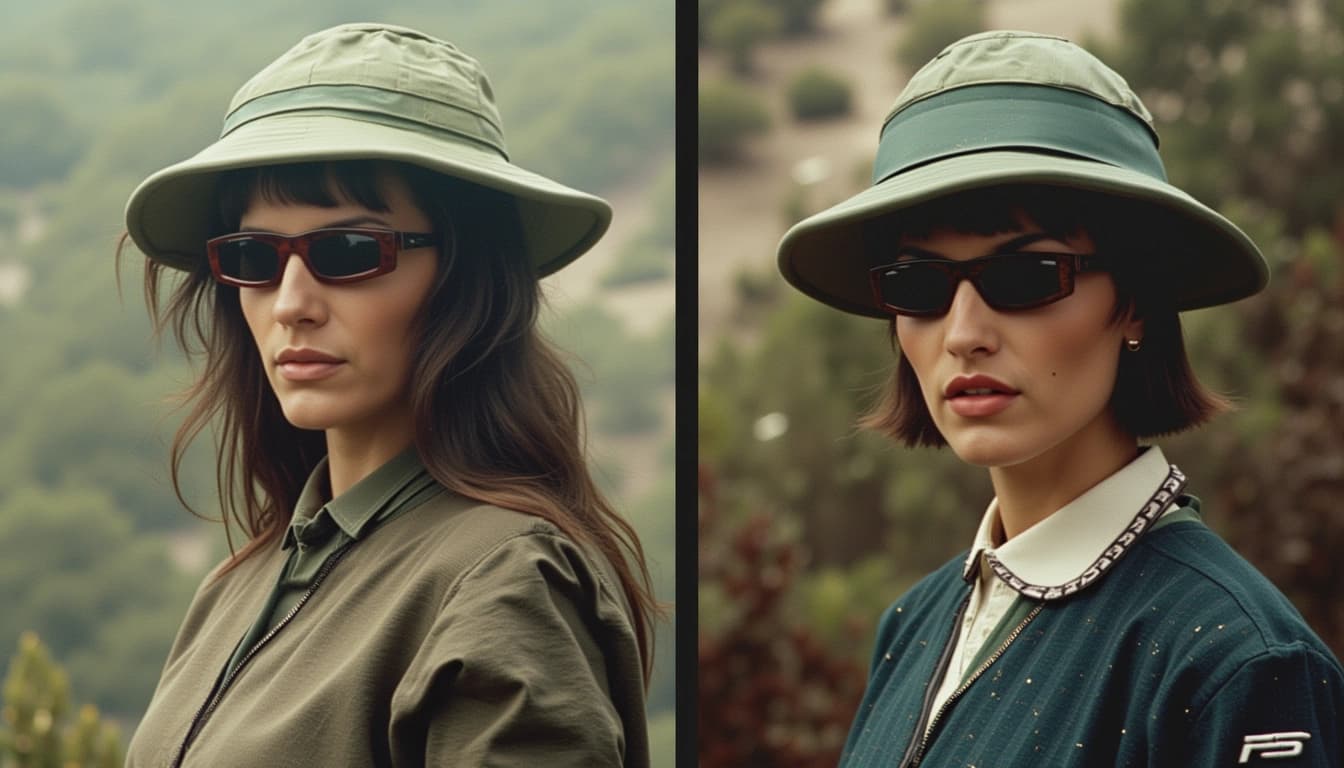
- Real-ESRGAN for general image restoration
- DDCOLOR for photo-realistic colorization
- GFP-GAN for blind face restoration
Old photos often hold sentimental value, but time can degrade their quality, making them blurry, discolored, or damaged. Fortunately, modern AI-powered image restoration tools can breathe new life into these cherished memories. These tools use advanced algorithms to enhance resolution, remove noise, restore colors, and even reconstruct missing details.
SwinIR: A Versatile Restoration Model
SwinIR (Swin Transformer for Image Restoration) is a powerful model based on the Swin Transformer architecture. It excels in tasks like super-resolution, denoising, and JPEG artifact removal. Its hierarchical design allows it to process images efficiently while maintaining high-quality output. SwinIR is particularly useful for old photos, as it can handle multiple types of degradation simultaneously.
Key Features of SwinIR
SwinIR's transformer-based approach enables it to capture long-range dependencies in images, which is crucial for restoring fine details. It also uses a lightweight design, making it scalable for different computational needs. This makes it a versatile choice for both casual users and professionals.
NAFNet: Efficient and Fast Restoration
NAFNet (Nonlinear Activation-Free Network) is designed for efficient image restoration. It removes nonlinear activation functions to reduce computational overhead, making it faster than many traditional models. NAFNet is ideal for tasks like deblurring and super-resolution, where speed and quality are both important.
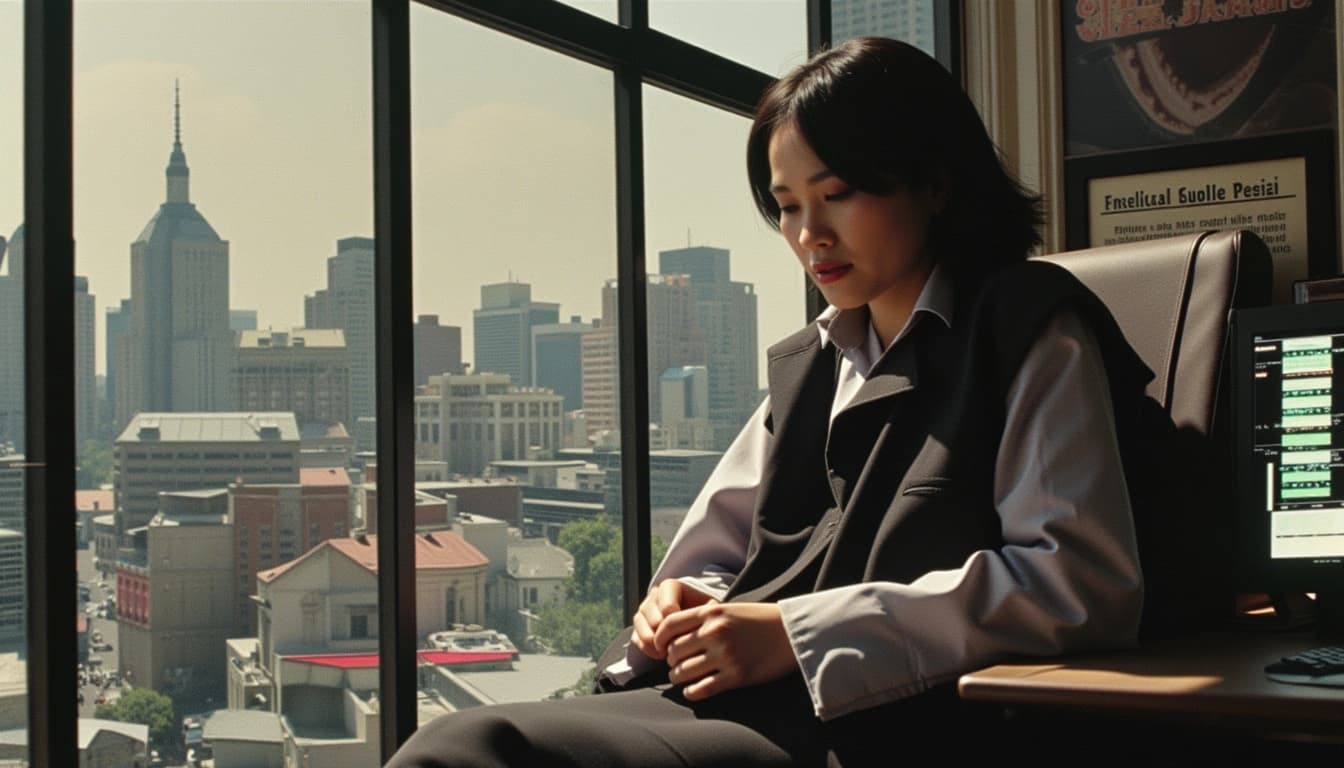
DDCOLOR: Bringing Color to Black and White Photos
DDCOLOR is a state-of-the-art colorization model that uses a dual-decoder architecture to add realistic colors to black and white photos. It leverages deep learning to predict plausible colors, even for complex scenes. This is especially valuable for restoring old family photos, where color can bring memories back to life.
How DDCOLOR Works
The model processes images in two stages: one decoder focuses on global color tones, while the other refines local details. This dual approach ensures that colors are both accurate and visually appealing. Users can also guide the colorization process with hints if needed.
GFP-GAN: Specialized Face Restoration
GFP-GAN (Generative Facial Prior-Generative Adversarial Network) is tailored for restoring faces in old photos. It combines generative facial priors with GANs to enhance facial details while preserving identity. This is crucial for portraits where facial features are often the most degraded.
Conclusion & Next Steps
Restoring old photos with AI is now more accessible than ever, thanks to models like SwinIR, NAFNet, DDCOLOR, and GFP-GAN. Each tool has its strengths, and combining them can yield the best results. Whether you're a hobbyist or a professional, these technologies can help preserve your precious memories for future generations.
- Use SwinIR for general restoration tasks like denoising and super-resolution.
- Try NAFNet for fast and efficient deblurring.
- Apply DDCOLOR to bring color back to black and white photos.
- Use GFP-GAN for enhancing facial details in portraits.
The restoration of old photos has seen significant advancements with the advent of AI models. These models can now effectively repair damaged, faded, or scratched images, bringing them back to life with remarkable clarity. The technology leverages deep learning to analyze and reconstruct missing or degraded parts of photographs.
Best AI Models for Old Photo Restoration
Several AI models have emerged as leaders in the field of photo restoration. SwinIR, NAFNet, DDCOLOR, and GFP-GAN are among the most effective tools for this purpose. Each model specializes in different aspects of restoration, such as general image repair, efficiency, colorization, and facial enhancement.
SwinIR for General Restoration
SwinIR is a transformer-based model known for its ability to handle general restoration tasks. It excels in removing noise, scratches, and other common damages found in old photos. The model's architecture allows it to capture both local and global features, making it highly effective for a wide range of restoration needs.
NAFNet for Efficient Processing
NAFNet stands out for its efficiency, providing fast and accurate restoration without the need for complex architectures. This model is particularly useful for users who need quick results without compromising on quality. Its streamlined design makes it a practical choice for everyday restoration tasks.
Colorization with DDCOLOR
DDCOLOR specializes in adding realistic colors to black-and-white photos. The model uses advanced algorithms to predict and apply colors that match the original scene as closely as possible. This capability is invaluable for bringing historical images to life with vibrant, natural hues.
Facial Enhancement with GFP-GAN
GFP-GAN focuses on restoring and enhancing facial features in portraits. The model is particularly adept at repairing blurred or damaged faces, making it a go-to tool for portrait restoration. Its ability to refine details ensures that the restored images look natural and lifelike.
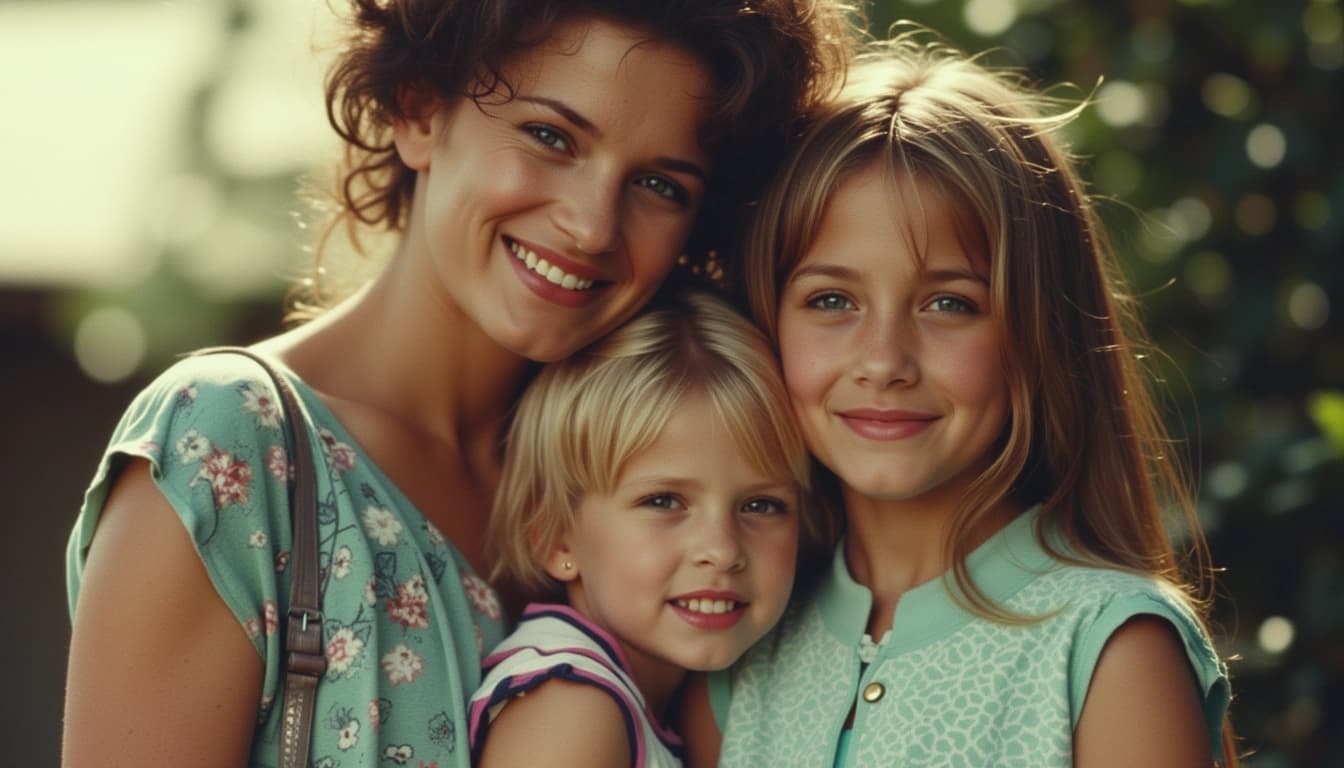
Challenges and Limitations
Despite their impressive capabilities, these AI models are not without limitations. Severely damaged photos may not be perfectly restored, as the AI can only work with the available information. Additionally, some models may alter certain details or reduce resolution, which can affect the final output.
Conclusion & Next Steps
AI-powered photo restoration has revolutionized the way we preserve and restore old photographs. Models like SwinIR, NAFNet, DDCOLOR, and GFP-GAN offer specialized solutions for various restoration needs. While challenges remain, the continuous advancement of AI technology promises even better results in the future.
- SwinIR for general image restoration
- NAFNet for efficient processing
- DDCOLOR for colorization
- GFP-GAN for facial enhancement
AI-powered image restoration has revolutionized the way we restore old or damaged photos. These advanced models leverage deep learning to remove scratches, enhance colors, and even reconstruct missing parts of images. The results are often astonishing, bringing old memories back to life with remarkable clarity.
SwinIR: A Breakthrough in Image Restoration
SwinIR is one of the leading models in the field of image restoration. It utilizes a Swin Transformer architecture to achieve high-quality results. This model is particularly effective for tasks like super-resolution, denoising, and JPEG compression artifact removal. Its ability to handle various degradation types makes it a versatile tool for photo restoration.
How SwinIR Works
SwinIR processes images in patches, allowing it to focus on local details while maintaining global coherence. The transformer-based approach enables it to capture long-range dependencies, which is crucial for reconstructing missing or damaged areas. This method outperforms traditional convolutional neural networks in many restoration tasks.
NAFNet: Simple Yet Powerful
NAFNet, or Non-Attention Feedforward Network, offers a simpler alternative to transformer-based models. Despite its simplicity, it delivers competitive performance in image restoration tasks. The model focuses on efficient feedforward operations, reducing computational overhead while maintaining high-quality results.
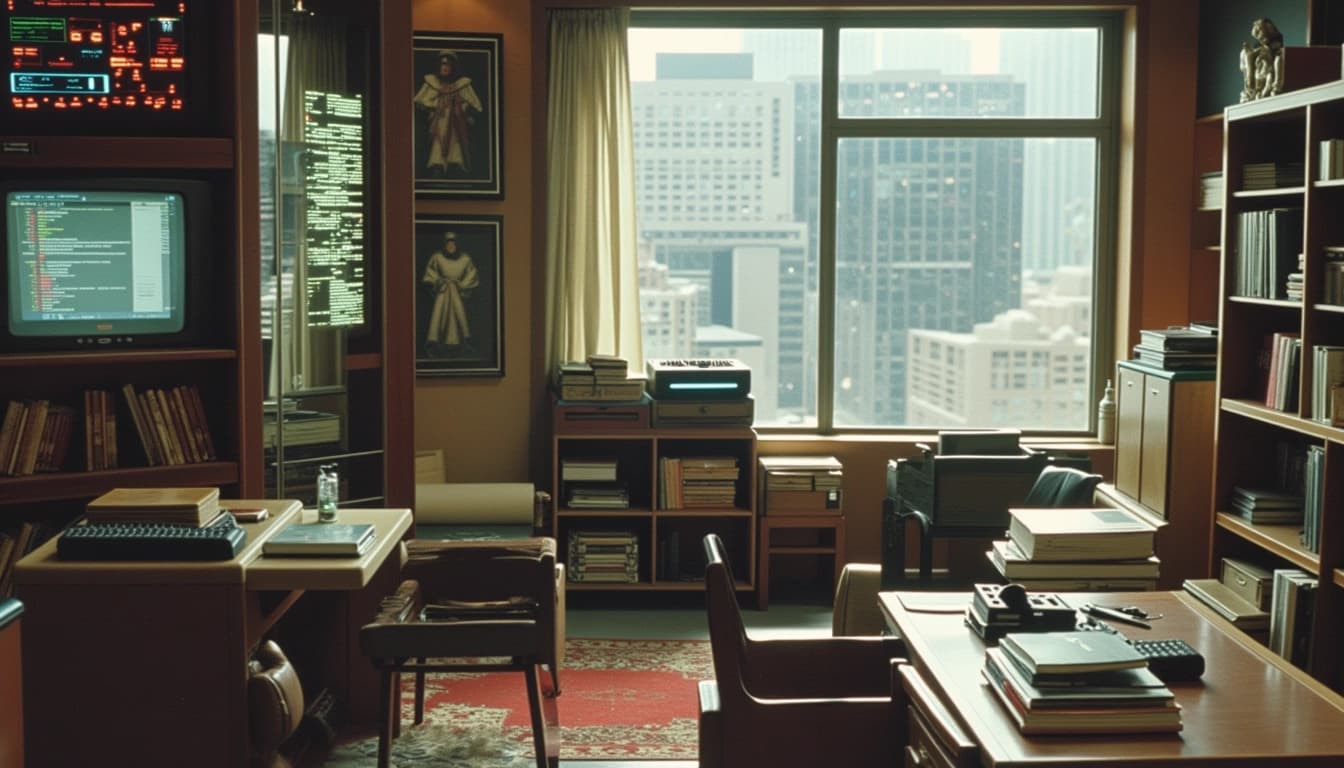
DDColor: Bringing Color Back to Life
DDColor specializes in photo colorization, transforming black-and-white images into vibrant, colorful pictures. The model uses dual decoders to ensure accurate color reproduction and natural-looking results. It’s particularly useful for restoring historical photos, giving them a fresh, modern appearance.
GFPGAN: Real-World Face Restoration
GFPGAN is designed for blind face restoration, tackling challenges like blurring, noise, and compression artifacts. It leverages generative facial priors to produce realistic and high-quality face images. This model is widely used for restoring old portraits and enhancing low-quality facial images.
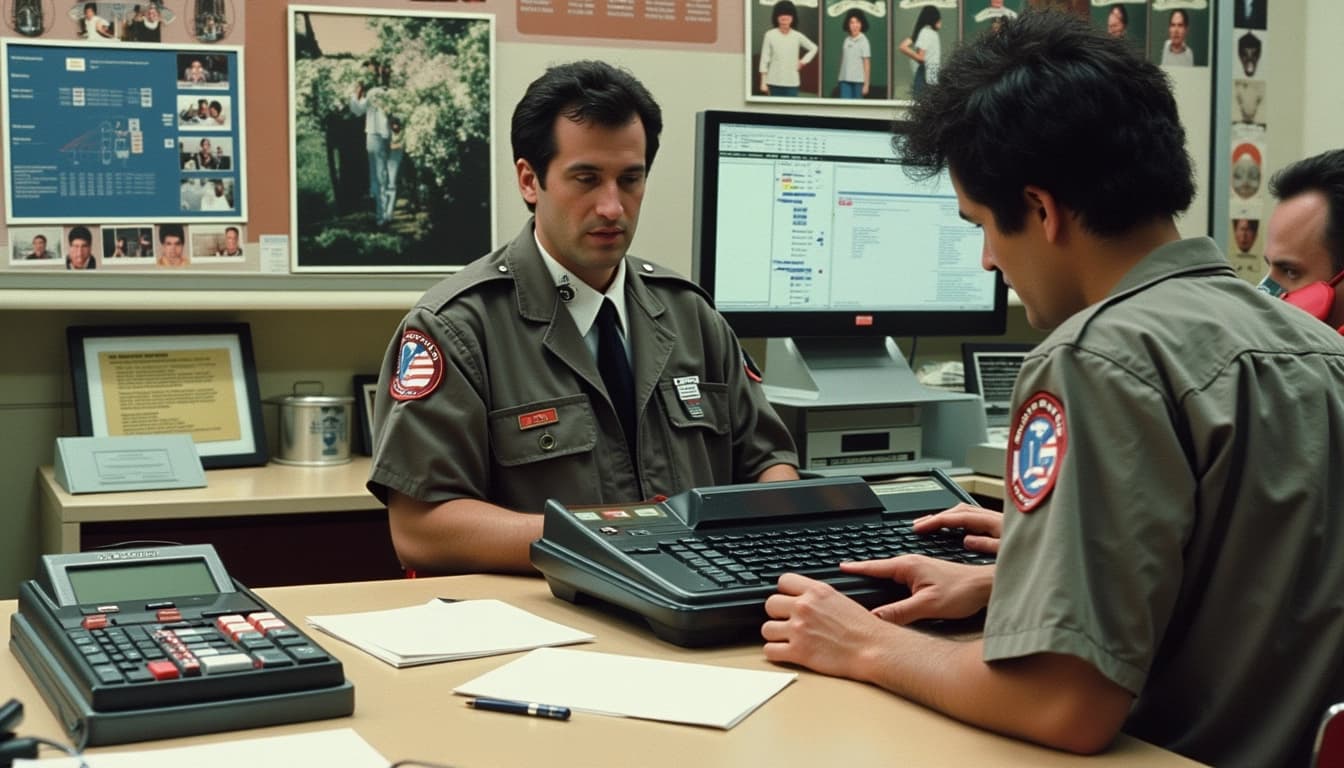
Conclusion & Next Steps
AI-powered image restoration tools have made significant strides in recent years, offering solutions for various restoration needs. From colorization to face enhancement, these models provide accessible and effective ways to preserve and rejuvenate old photos. As technology advances, we can expect even more impressive results in the future.
- Explore SwinIR for general image restoration tasks.
- Use DDColor for accurate photo colorization.
- Try GFPGAN for restoring old or damaged portraits.