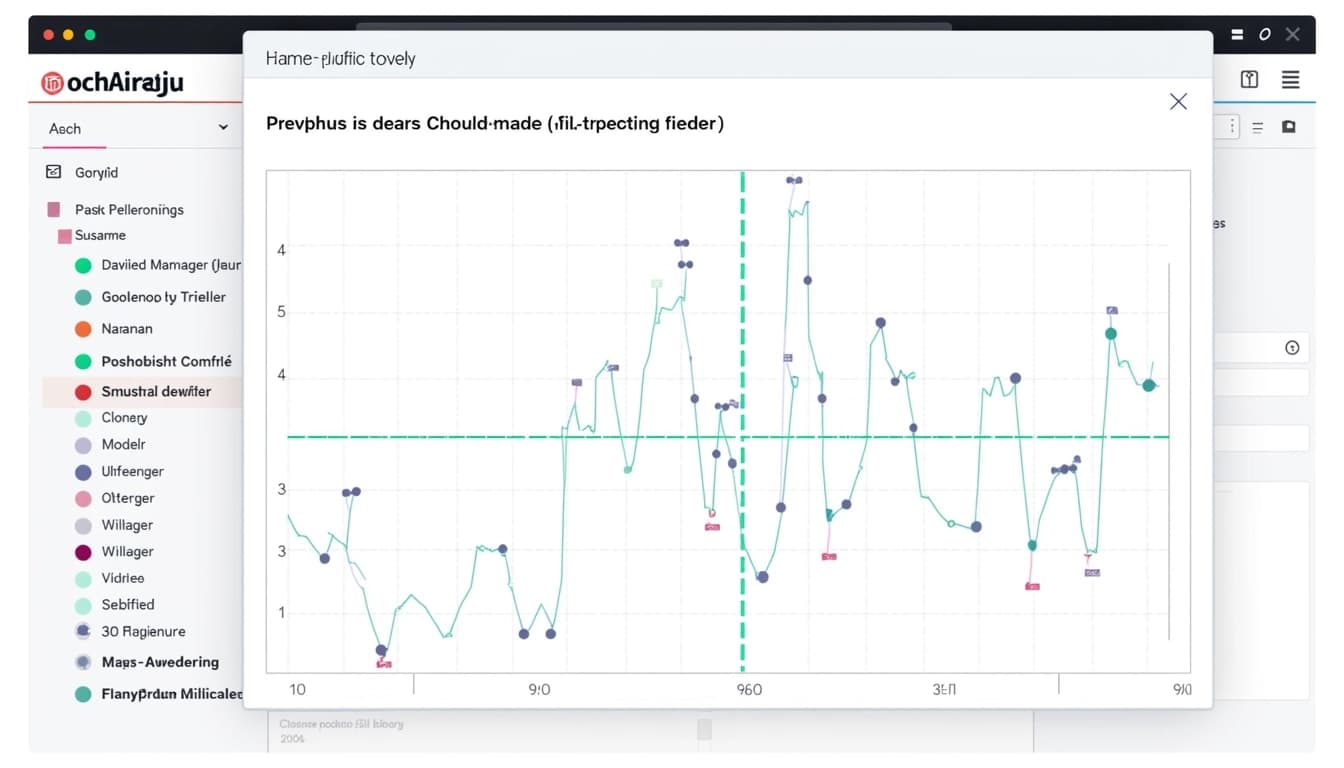
Key Points and Survey Note on AI Upscaling Models
By John Doe 5 min
Key Points
Es scheint wahrscheinlich, dass KI-Upscaling-Modelle kleine Bilder in große Kunstwerke verwandeln, indem sie tiefes Lernen und GANs nutzen.
Die Forschung legt nahe, dass diese Modelle durch das Training mit LR- und HR-Bildpaaren detaillierte, realistische Ergebnisse erzielen.
Es gibt Kontroversen über Artefakte und unnatürliche Details, insbesondere bei sehr niedriger Auflösung.
Was ist KI-Upscaling?
KI-Upscaling, auch als Super-Resolution bekannt, ist ein Prozess, bei dem kleine, niedrig aufgelöste Bilder (LR) durch künstliche Intelligenz in größere, hochauflösende Bilder (HR) umgewandelt werden. Diese Modelle lernen aus großen Datensätzen, um fehlende Details hinzuzufügen und die Bildqualität zu verbessern.
Wie funktioniert es?
Diese Modelle verwenden tiefes Lernen, insbesondere konvolutionale neuronale Netze (CNNs), und oft Generative Adversarial Networks (GANs). GANs bestehen aus einem Generator, der LR-Bilder in HR-Bilder umwandelt, und einem Diskriminator, der echte von generierten Bildern unterscheidet. Durch kompetitives Training verbessert sich der Generator, um realistischere Bilder zu erzeugen.
Anwendungen
KI-Upscaling wird für die Restaurierung alter Fotos, die Verbesserung von Videospielgrafiken und die Aufwertung von E-Commerce-Bildern verwendet. Ein unerwartetes Detail ist, dass es auch für die Verbesserung von Überwachungsvideos genutzt wird, um Details klarer zu machen.
Survey Note: Detailed Exploration of AI Upscaling Models
In the digital era of 2025, the demand for high-quality images has surged, driven by the proliferation of high-resolution displays and the need for professional visuals across various platforms. This survey note delves into how AI upscaling models transform small, low-resolution images into large, detailed artworks, exploring the technology, methodologies, applications, limitations, and future directions. The analysis is grounded in extensive research and aims to provide a compr
Image upscaling, the process of increasing an image's size while maintaining or enhancing its quality, is crucial in fields like photography, design, and digital content creation. Traditional methods, such as nearest neighbor, bilinear, and bicubic interpolation, often result in pixelated or blurry images, especially at high scaling factors. These methods rely on mathematical estimations based on existing pixel values, which fail to add new details effectively.
AI-based upscaling, or super-resolution, offers a revolutionary approach by leveraging machine learning to predict and generate missing details. This technology is particularly vital in 2025, where users expect crisp, high-definition images for social media, print media, and digital art, even from originally low-quality sources.
Technological Foundations: Deep Learning and GANs
AI upscaling models primarily use deep learning, with convolutional neural networks (CNNs) being a cornerstone due to their ability to process grid-like data like images. One of the earliest models, SRCNN (Super-Resolution Convolutional Neural Network), introduced in 2014, mapped low-resolution (LR) to high-resolution (HR) images using a simple three-layer CNN, marking the beginning of AI-driven super-resolution.
A significant advancement came with Generative Adversarial Networks (GANs), first applied to super-resolution with SRGAN in 2016. GANs consist of two networks: the generator, which creates upscaled images from LR inputs, and the discriminator, which distinguishes between these generated images and actual HR images. The competitive training process, where the generator aims to fool the discriminator, leads to increasingly realistic outputs.
Enhancements with ESRGAN
ESRGAN, an enhanced version of SRGAN, introduced in 2018, improved upon this by modifying network architecture (using Residual-in-Residual Dense Blocks without batch normalization) and loss functions. These changes allowed for sharper and more detailed images, setting a new benchmark in the field of AI-based upscaling.
Applications and Future Directions
The applications of AI upscaling are vast, ranging from restoring old photographs to enhancing medical imaging. Future directions include integrating these models into real-time applications, such as video streaming, and further improving the perceptual quality of upscaled images through advanced training techniques.
Conclusion & Next Steps
AI-based image upscaling represents a significant leap forward in digital imaging technology. By leveraging deep learning and GANs, it addresses the limitations of traditional methods, offering superior results. As the technology continues to evolve, we can expect even more impressive advancements in the near future.
- Explore different AI upscaling tools available in the market.
- Experiment with various models to find the best fit for your specific needs.
- Stay updated with the latest research and advancements in the field.
AI image upscaling leverages deep learning to enhance low-resolution images, transforming them into high-quality visuals with remarkable detail. This technology has evolved significantly, moving beyond traditional methods like bicubic interpolation to sophisticated neural networks that predict and fill in missing details. The process involves training models on vast datasets of image pairs, enabling them to learn intricate patterns and textures.
The Technology Behind AI Upscaling
Modern AI upscaling relies on convolutional neural networks (CNNs) and generative adversarial networks (GANs) to achieve superior results. CNNs analyze the image at multiple scales, capturing both local and global features, while GANs introduce a discriminator network to ensure the generated images are photorealistic. These models, such as ESRGAN and Real-ESRGAN, have set new benchmarks in the field, offering unprecedented clarity and detail in upscaled images.
Key Innovations in Neural Networks
Recent advancements include the use of residual connections and attention mechanisms, which allow networks to focus on critical image regions and preserve fine details. Techniques like perceptual loss and adversarial training further refine the output, ensuring that upscaled images not only look sharp but also natural. These innovations have made AI upscaling a go-to solution for professionals and hobbyists alike.
Applications Across Industries
AI upscaling is revolutionizing fields such as photography, gaming, and surveillance. Photographers can restore old or damaged photos, while gamers enhance textures for a more immersive experience. In surveillance, low-resolution footage is upscaled to identify critical details, aiding in security and investigations. The versatility of this technology makes it invaluable across diverse sectors.
Challenges and Future Directions
Despite its successes, AI upscaling faces challenges like computational intensity and the need for large datasets. Future research aims to reduce these barriers, making the technology more accessible. Innovations like lightweight models and unsupervised learning could democratize AI upscaling, enabling real-time applications on mobile devices and other low-power platforms.
Conclusion & Next Steps
AI image upscaling represents a significant leap forward in digital image processing, offering tools to enhance visual content like never before. As the technology continues to evolve, we can expect even more sophisticated solutions that push the boundaries of what's possible. For those interested in exploring this field, experimenting with open-source models and datasets is a great starting point.
- Explore open-source models like ESRGAN
- Experiment with different datasets
- Stay updated on the latest research papers
AI image upscaling has revolutionized the way we enhance low-resolution images, transforming them into high-quality visuals with remarkable detail. This technology leverages advanced machine learning models to predict and fill in missing pixels, making it invaluable for various industries.
How AI Image Upscaling Works
AI image upscaling employs deep learning models like ESRGAN and SRCNN to analyze low-resolution images and generate high-resolution versions. These models are trained on vast datasets of image pairs, learning to predict missing details and textures. The process involves feeding the low-res image into the model, which then outputs an upscaled version with enhanced clarity and detail.
Key Technologies Behind AI Upscaling
Technologies such as Generative Adversarial Networks (GANs) and Convolutional Neural Networks (CNNs) are at the core of AI upscaling. GANs, for instance, use a generator to create high-res images and a discriminator to evaluate their quality, iteratively improving the output. CNNs, on the other hand, focus on extracting and enhancing features from the input image.
Applications of AI Image Upscaling
AI upscaling is widely used in fields like photography, surveillance, and e-commerce. Photographers can restore old or low-quality images, while surveillance systems benefit from clearer footage for better identification. E-commerce platforms use upscaling to improve product images, enhancing the shopping experience.
Limitations and Challenges
Despite its advantages, AI upscaling has limitations. The quality of the output depends heavily on the input image, and very low-resolution images may not upscale well. Additionally, models can introduce artifacts or hallucinate details, and the computational resources required can be significant.
Future Directions
The future of AI upscaling lies in advanced network designs, such as transformers, and hybrid approaches combining multiple techniques. These innovations aim to improve accuracy and reduce computational costs, making the technology more accessible and effective.
- Enhanced detail prediction
- Reduced computational costs
- Broader application across industries
AI upscaling models have revolutionized the way we enhance low-resolution images, transforming them into high-quality, detailed visuals. These models leverage advanced deep learning techniques to predict and generate missing details, making them invaluable for photo restoration, digital art, and gaming. The technology has evolved significantly over the years, offering solutions that were once considered impossible.
The Evolution of AI Upscaling Models
The journey of AI upscaling began with simple convolutional neural networks (CNNs) like SRCNN, which mapped low-resolution (LR) images directly to high-resolution (HR) ones. While effective, these early models struggled with generating fine details. The introduction of Generative Adversarial Networks (GANs) marked a turning point, enabling models like SRGAN to produce more realistic textures through adversarial training. Subsequent advancements, such as ESRGAN and Real-ESRGAN, further refined these techniques, addressing artifacts and improving visual quality.
Key Breakthroughs in AI Upscaling
One of the most significant breakthroughs was the integration of perceptual loss functions, which prioritize visual quality over pixel-level accuracy. This approach allows models to generate sharper edges and more natural textures. Another milestone was the development of multi-frame super-resolution, which leverages multiple LR frames from videos to enhance temporal consistency and detail preservation. These innovations have expanded the applications of AI upscaling beyond static images to dynamic content like films and video games.
Applications of AI Upscaling
AI upscaling is now widely used in various industries, from restoring old photographs to enhancing medical imaging. In the entertainment sector, it enables the remastering of classic films in 4K resolution, breathing new life into outdated footage. Digital artists also benefit from these tools, as they can upscale sketches or low-res artwork without losing quality. Additionally, gaming companies use AI upscaling to improve textures and reduce rendering times, enhancing the player experience.
Challenges and Future Directions
Despite its successes, AI upscaling faces challenges such as computational demands and occasional artifacts in complex scenes. Researchers are actively working on solutions, including lightweight models for real-time applications and user-interactive upscaling, where users can guide the process. The future of AI upscaling lies in combining these advancements with emerging technologies like neural radiance fields (NeRFs) for even more realistic results.
Conclusion & Next Steps
AI upscaling has come a long way, offering powerful tools to transform low-quality images into stunning high-resolution visuals. As the technology continues to evolve, we can expect even more sophisticated models capable of handling diverse use cases with greater efficiency. For those interested in exploring this field, experimenting with open-source tools like Real-ESRGAN or commercial solutions like Topaz Gigapixel is a great starting point.
- Experiment with open-source AI upscaling tools
- Explore commercial solutions for specific needs
- Stay updated on the latest research and advancements
AI image upscaling has revolutionized the way we enhance low-resolution images, transforming them into high-definition masterpieces. This technology leverages advanced algorithms to predict and fill in missing details, making it invaluable for photographers, designers, and content creators. The process involves sophisticated neural networks that analyze patterns and textures to produce sharp, clear images.
The Technology Behind AI Image Upscaling
AI image upscaling primarily relies on deep learning models, such as Generative Adversarial Networks (GANs) and Convolutional Neural Networks (CNNs). These models are trained on vast datasets of high- and low-resolution image pairs, learning to predict the most accurate high-resolution version of any given low-resolution input. The result is a seamless upscaling process that preserves details and reduces artifacts.
Generative Adversarial Networks (GANs)
GANs consist of two neural networks, a generator and a discriminator, that work in tandem to produce highly realistic images. The generator creates upscaled images, while the discriminator evaluates them against real high-resolution images, providing feedback to improve the generator's output. This adversarial process continues until the generator produces images indistinguishable from real ones.
Popular AI Upscaling Tools
Several AI-powered tools have emerged as leaders in the image upscaling space, offering users the ability to enhance their photos with minimal effort. Tools like Upscayl and LetsEnhance provide intuitive interfaces and powerful algorithms, making high-quality upscaling accessible to both professionals and hobbyists. These platforms often support batch processing, allowing users to upscale multiple images simultaneously.
Challenges and Limitations
Despite its advancements, AI image upscaling is not without its challenges. One common issue is the introduction of artifacts or unnatural textures in the upscaled images, particularly when the input quality is extremely low. Additionally, the computational resources required for high-quality upscaling can be significant, limiting real-time applications on less powerful devices.
Future of AI Image Upscaling
The future of AI image upscaling looks promising, with ongoing research focused on improving accuracy and efficiency. Innovations such as real-time upscaling and integration with mobile devices are expected to become more prevalent. As algorithms continue to evolve, we can anticipate even more realistic and detailed upscaled images, further blurring the line between original and enhanced content.
Conclusion & Next Steps
AI image upscaling has undeniably transformed digital imaging, offering unparalleled enhancements to low-resolution photos. While challenges remain, the technology's potential is vast, with applications ranging from photography to medical imaging. As tools become more accessible and algorithms more refined, the possibilities for creative and practical uses will only expand.
- Explore AI upscaling tools like Upscayl and LetsEnhance
- Experiment with different upscaling settings to achieve optimal results
- Stay updated on the latest advancements in AI image enhancement