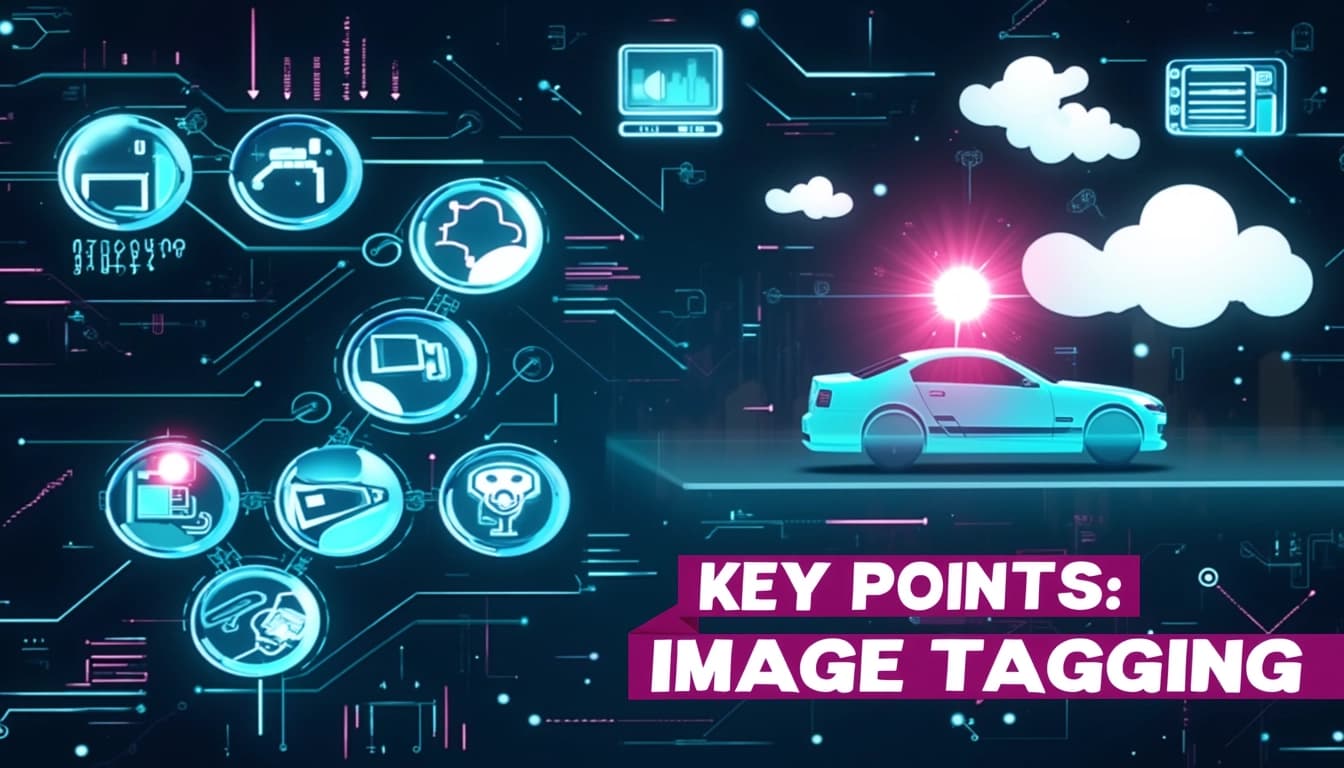
Key Points on AI Image Tagging
By John Doe 5 min
Key Points
It seems likely that "vcd-image-tagger" is not a widely recognized tool, possibly a niche or custom AI image tagging solution.
AI image tagging automates labeling images with keywords, enhancing searchability and organization, using machine learning to detect objects and scenes.
Tools like PhotoTag.ai, Imagga, and Azure AI Vision offer unique features, but specific differences for vcd-image-tagger are unclear without more information.
Research suggests that AI image taggers vary in accuracy, speed, and customization, with some supporting metadata integration and others focusing on user-friendly interfaces.
Introduction to AI Image Tagging
AI image tagging is a technology that uses artificial intelligence, particularly computer vision and machine learning, to automatically assign relevant keywords or tags to images. This process helps in organizing, searching, and managing large collections of visual content, making it easier for businesses, photographers, and individuals to find specific images quickly. By analyzing the content of an image—such as objects, scenes, colors, and even text—AI can generate descriptive tags that enhance accessibility and usability.
What Makes AI Image Tagging Tools Different?
While the specific tool "vcd-image-tagger" is not widely documented, AI image tagging tools generally differ in several key areas:
- Accuracy and Training: Some tools, like Imagga, use deep learning models trained on thousands of objects, offering high precision, while others can be customized for specific needs.
- Integration and Workflow: Tools like PhotoTag.ai integrate seamlessly with platforms like Lightroom, providing metadata export, whereas others, such as Azure AI Vision, offer API access for developers.
- User Interface: Some tools focus on user-friendly interfaces for non-technical users, while others provide advanced features for developers.
AI image tagging is revolutionizing how we manage and organize digital images. By leveraging machine learning and computer vision, these tools can automatically assign relevant tags to images, making them easier to search and categorize. This technology is particularly beneficial for industries like e-commerce, media, and research, where large volumes of images need to be processed efficiently.
Understanding AI Image Tagging
AI image tagging works by analyzing the content of an image to identify objects, scenes, colors, and even text. Advanced models, trained on vast datasets, can recognize thousands of objects and contexts. For example, tools like Imagga can detect over 3,000 objects, while Azure AI Vision focuses on contextual tags such as 'indoor' or 'outdoor.' This automation significantly reduces manual effort and improves accuracy.
Key Features of AI Image Tagging
The key features of AI image tagging include object recognition, scene detection, and text extraction. These features enable semantic search, allowing users to find images based on their content rather than just filenames. Additionally, some tools offer customization options, letting users train models on specific datasets to improve tagging accuracy for niche applications.
Comparing vcd-image-tagger with Other Tools
While many AI image tagging tools are available, vcd-image-tagger may offer unique advantages depending on its design. For instance, it might excel in metadata handling or specialized object recognition. Comparing it with other tools like Cloudinary or Contentful's AI Image Tagging can help users determine which solution best fits their needs, whether it's ease of use, scalability, or cost-effectiveness.
Conclusion & Next Steps
AI image tagging is a powerful tool for managing digital assets, offering significant time savings and improved accuracy. Whether you choose vcd-image-tagger or another solution, it's important to evaluate your specific requirements, such as the volume of images, customization needs, and budget. By doing so, you can select the best tool to streamline your workflow and enhance your image management processes.
- Evaluate your image tagging needs
- Compare different AI tagging tools
- Test the selected tool with a sample dataset
- Implement the tool into your workflow
AI image tagging tools are revolutionizing how we organize and search for images. These tools use machine learning to automatically generate descriptive tags, saving time and improving accuracy. From personal photo libraries to large-scale digital asset management, AI tagging is becoming indispensable.
Key Features of AI Image Tagging Tools
Modern AI image taggers offer a range of features designed to streamline workflows. They can identify objects, scenes, and even emotions in images. Some tools allow for custom training to recognize specific categories, making them adaptable to various industries. The best solutions integrate seamlessly with existing platforms like Lightroom or cloud storage services.
Accuracy and Customization
Accuracy is a critical factor when choosing an AI tagging tool. Many services boast high precision rates, but performance can vary depending on image quality and content. Customization options, such as the ability to add user-defined tags or exclude certain categories, can significantly enhance usability for specialized needs.
Popular AI Image Tagging Solutions
Several leading solutions dominate the AI image tagging market. PhotoTag.ai is known for its creative workflow integration, while Imagga offers extensive customization through training. Cloudinary provides a versatile API that works with multiple AI providers, and Azure AI Vision delivers enterprise-grade scalability for large organizations.
Implementation Considerations
When implementing an AI tagging solution, several factors should be considered. API costs can add up quickly for large image libraries, making local processing options attractive. Privacy concerns may also influence the choice between cloud-based and on-premises solutions. Integration capabilities with existing systems are another crucial aspect to evaluate.
Future of AI Image Tagging
The future of AI image tagging looks promising, with advancements in computer vision and natural language processing. We can expect more contextual understanding, where the AI recognizes relationships between objects in images. Real-time tagging for video content is another area of rapid development that will expand the applications of this technology.
Conclusion
AI image tagging tools are transforming how we manage visual content. From personal use to enterprise applications, these solutions offer significant time savings and improved organization. As the technology continues to evolve, we'll see even more sophisticated capabilities that further bridge the gap between human and machine understanding of visual content.
- Evaluate accuracy and customization options when choosing a tool
- Consider API costs versus local processing solutions
- Look for seamless integration with existing workflows
- Stay updated on advancements in contextual understanding
The article discusses the concept of AI image tagging, highlighting its importance in organizing and categorizing digital images efficiently. It explores various tools and technologies available in the market that leverage artificial intelligence to automate the tagging process.
Overview of AI Image Tagging
AI image tagging refers to the use of machine learning models to automatically assign relevant tags to images based on their content. This technology is widely used in digital asset management, e-commerce, and social media platforms to enhance searchability and organization. The accuracy of these tags depends on the training data and the algorithms used.
Key Features of AI Image Tagging Tools
Modern AI image tagging tools offer features like multi-label classification, object detection, and facial recognition. These tools can identify not only objects but also emotions, colors, and even contextual elements within an image. Some advanced solutions also provide metadata embedding and integration with cloud storage services.
Popular AI Image Tagging Solutions
Several platforms provide AI-powered image tagging services, each with unique strengths. For instance, some focus on high accuracy for specific industries, while others prioritize scalability and ease of integration. Open-source solutions are also available for developers who prefer customizable options.
Challenges in AI Image Tagging
Despite its advantages, AI image tagging faces challenges such as handling ambiguous images, ensuring data privacy, and reducing computational costs. These issues are particularly relevant in industries like healthcare and finance, where accuracy and confidentiality are critical.
Future of AI Image Tagging
The future of AI image tagging lies in advancements like zero-shot learning, which allows models to tag images without prior training on specific categories. Integration with emerging AI models and improved user interfaces will further enhance the usability and accessibility of these tools.
Conclusion & Next Steps
AI image tagging is a rapidly evolving field with significant potential to transform how we manage and interact with digital images. Businesses and developers should explore the available tools and consider their specific needs when implementing these solutions. Staying updated with the latest advancements will be key to leveraging this technology effectively.
- Evaluate your image tagging needs
- Compare different AI tagging tools
- Consider privacy and scalability requirements
The AI Image Auto Tagger is a powerful tool designed to automatically assign relevant tags or keywords to images and videos. This technology leverages advanced machine learning algorithms to analyze visual content and generate descriptive metadata. The system can process vast collections of media files efficiently, saving time and effort for users who need to organize or search through large datasets.
How AI Image Auto Tagging Works
AI image auto tagging works by using deep learning models trained on large datasets of labeled images. These models can recognize objects, scenes, and even emotions depicted in the visuals. The process involves extracting features from the images, comparing them to known patterns, and assigning the most relevant tags. This technology is continuously improving as more data is fed into the system, enhancing its accuracy and reliability.
Key Technologies Behind Auto Tagging
Several key technologies power AI image auto tagging, including convolutional neural networks (CNNs) and transfer learning. CNNs are particularly effective for image recognition tasks due to their ability to capture spatial hierarchies in visual data. Transfer learning allows models to leverage pre-trained networks, reducing the need for extensive training data and computational resources.
Applications of AI Image Auto Tagging
AI image auto tagging has a wide range of applications across various industries. In e-commerce, it helps categorize products and improve search functionality. Media companies use it to organize and retrieve assets quickly. Educational institutions benefit from automated tagging for digital libraries. The technology is also useful in healthcare for managing medical imaging data.
Benefits of Using AI for Image Tagging
Using AI for image tagging offers numerous benefits, including increased efficiency, consistency, and scalability. Manual tagging is time-consuming and prone to human error, while AI can process thousands of images in minutes with high accuracy. Additionally, AI systems can adapt to new tagging requirements and improve over time, making them a cost-effective solution for long-term use.
Challenges and Limitations
Despite its advantages, AI image auto tagging is not without challenges. The accuracy of tags depends on the quality and diversity of the training data. Biases in the data can lead to incorrect or unfair tagging. Furthermore, the technology may struggle with abstract or ambiguous visuals that require human interpretation. Ongoing research and development are essential to address these limitations.
Future Trends in AI Image Tagging
The future of AI image tagging looks promising, with advancements in multimodal AI and real-time processing. Multimodal AI combines visual and textual data to generate more comprehensive tags. Real-time tagging enables instant categorization of live video streams. These innovations will further enhance the utility and accessibility of AI-powered tagging solutions.
Conclusion & Next Steps
AI image auto tagging is revolutionizing how we manage and interact with visual content. By automating the tagging process, it saves time, reduces errors, and improves accessibility. Organizations looking to implement this technology should evaluate their specific needs, choose the right tools, and ensure proper training data. As AI continues to evolve, the capabilities of image tagging will only expand.
- Evaluate your image tagging needs
- Choose an AI tagging solution that fits your requirements
- Ensure high-quality training data for accurate results
- Monitor and update the system regularly
Image tagging is a powerful tool for organizing and managing digital photos. By assigning descriptive keywords or labels to images, users can easily search and retrieve specific photos from large collections. This process is essential for photographers, marketers, and anyone dealing with extensive image libraries.
Benefits of Image Tagging
Image tagging offers numerous advantages, including improved searchability and organization. With proper tags, users can quickly locate images based on specific criteria, such as location, event, or subject. Additionally, tagging enhances collaboration by making it easier for teams to share and access relevant images.
Enhanced Searchability
One of the primary benefits of image tagging is the ability to find images quickly. Instead of scrolling through hundreds or thousands of photos, users can simply search for a tag and instantly access the desired images. This is particularly useful for professionals who need to manage large portfolios or marketing materials.
Popular Image Tagging Tools
There are several tools available for image tagging, each offering unique features. Some popular options include Adobe Lightroom, Google Photos, and specialized AI-powered tools like Plugger.ai. These tools often come with advanced features like automatic tagging, facial recognition, and cloud storage integration.
AI and Image Tagging
Artificial Intelligence is revolutionizing image tagging by automating the process. AI-powered tools can analyze images and assign relevant tags without human intervention. This not only saves time but also improves accuracy, as AI can recognize patterns and details that might be missed by humans.
Zero-Shot Image Tagging
Zero-shot image tagging is an advanced AI technique that allows models to tag images without prior training on specific categories. This approach is highly flexible and can adapt to new tagging requirements, making it ideal for dynamic environments where tagging needs may change frequently.
Conclusion & Next Steps
Image tagging is an essential practice for anyone managing large collections of digital photos. Whether you're a professional photographer or a casual user, leveraging the right tools and techniques can significantly enhance your workflow. Consider exploring AI-powered solutions for even greater efficiency and accuracy.
- Explore different image tagging tools
- Consider AI-powered solutions for automation
- Regularly update and refine your tags for better organization