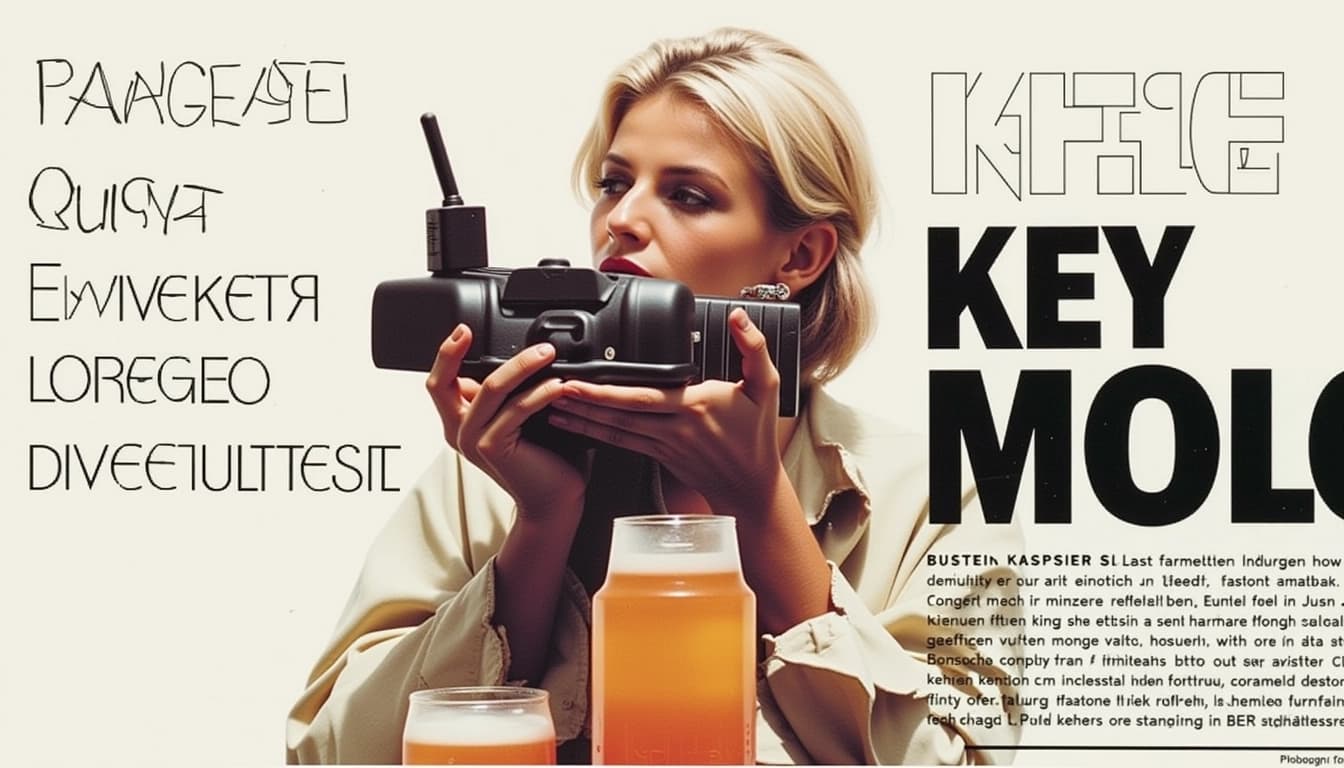
Key Points on Molmo-7b
By John Doe 5 min
Key Points
Research suggests molmo-7b is a step forward for open-ended visual question answering (VQA), outperforming previous open-source models like Pixtral 12B.
It achieves strong results on benchmarks like VQA v2.0, with Molmo-7B-D scoring 85.6% compared to Pixtral 12B's 78.6%.
The model is fully open-source, enhancing accessibility and community development, which is an unexpected benefit for researchers and developers.
Its training on the high-quality PixMo dataset, with 1 million image-text pairs, likely contributes to its effectiveness.
Introduction
Molmo-7b, developed by the Allen Institute for AI, has emerged as a notable advancement in the field of open-ended visual question answering (VQA). This task involves answering free-form questions based on images, requiring both visual understanding and natural language generation. Given its recent performance and open-source nature, it seems likely that molmo-7b represents a significant step forward, particularly when compared to previous models.
Performance Comparison
Molmo-7b, specifically the 7B-D variant, demonstrates impressive results on academic benchmarks. For instance, it scores 85.6% on the VQA v2.0 test, which is higher than Pixtral 12B's 78.6% on the same metric. This suggests molmo-7b is more effective at handling open-ended VQA tasks, even with fewer parameters (7 billion vs. 12 billion for Pixtral 12B). Additionally, human evaluations show molmo-7b models have higher Elo scores, indicating better user preference in pairwise comparisons.
Open-Source and Accessibility
An unexpected detail is molmo-7b's commitment to openness. It is fully open-source, with model weights, the PixMo dataset, and training code available for public use. This transparency fosters community involvement and reproducibility, making advanced AI more accessible to researchers and developers, especially those working on multimodal applications.
Dataset and Architecture
The evidence leans toward molmo-7b's effectiveness being tied to its training on the high-quality PixMo dataset, which contains 1 million image-text pairs. This dataset likely provides the diverse and rich training examples needed for robust VQA performance.
Molmo-7b, a family of vision-language models developed by the Allen Institute for AI, has garnered attention for its potential to advance open-ended visual question answering (VQA). Open-ended VQA involves generating free-form natural language answers to questions based on images, a task that requires robust multimodal understanding.
Performance Analysis
Molmo-7b's performance has been evaluated on 11 academic benchmarks, including AI2D test, ChartQA test, VQA v2.0 test, DocQA test, InfographicVQA test, TextVQA val, RealWorldQA, MMMU val, MathVista testmini, CountBenchQA, and Flickr Count. The Molmo-7B-D variant achieves an average score of 77.3 across these benchmarks, with a notable 85.6% on VQA v2.0, which is an open-ended VQA task.
Dataset and Training
The model's success is attributed to its training on the PixMo dataset, which includes 1 million high-quality, human-annotated image-text pairs. This focus on data quality, combined with its use of advanced architectures like Qwen2-7B and OpenAI's CLIP for vision, likely enhances its ability to process both images and text effectively.
Open-Source Nature
Molmo-7b is fully open-source, allowing researchers and developers to access, modify, and build upon its architecture. This openness fosters collaboration and innovation in the field of multimodal AI, setting it apart from proprietary models that restrict access.
Architectural Innovations
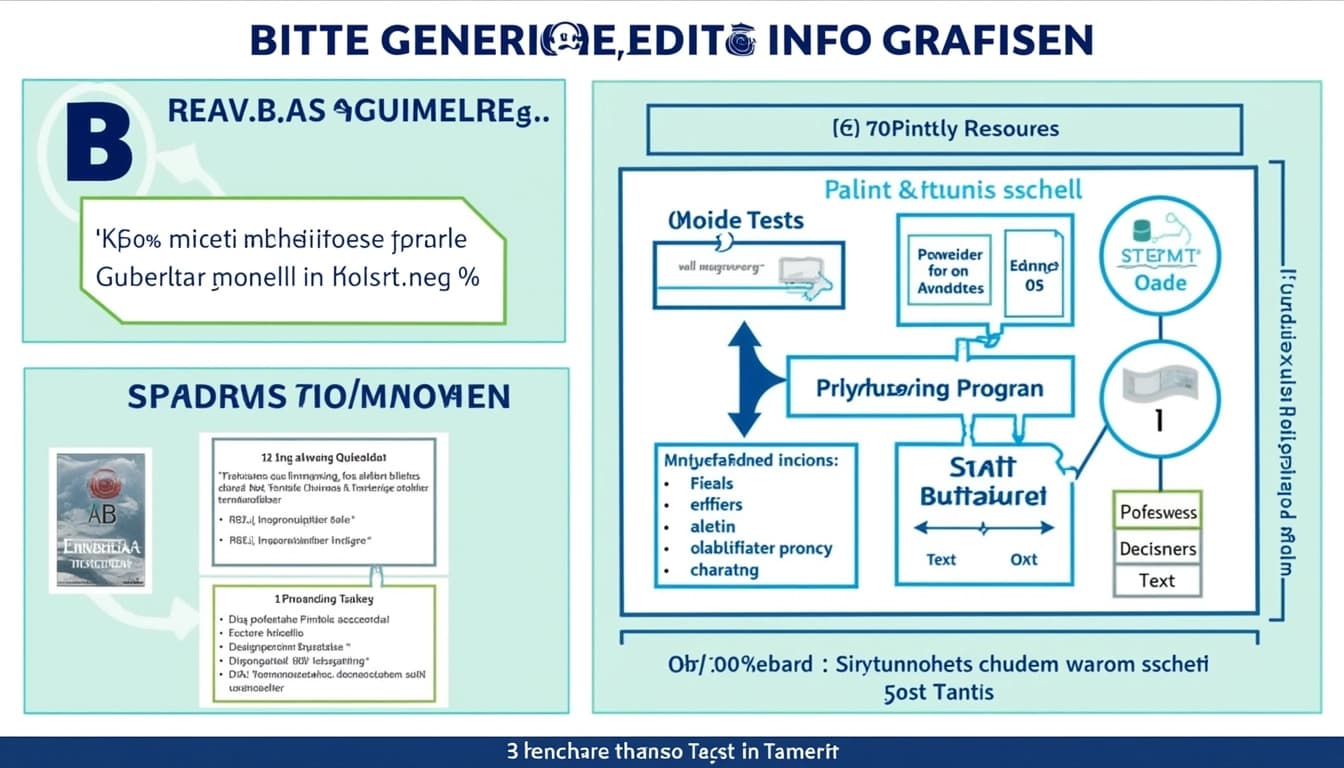
The model leverages a combination of Qwen2-7B for language processing and OpenAI's CLIP for vision, enabling seamless integration of visual and textual data. This hybrid approach ensures robust performance across diverse VQA tasks.
Conclusion & Next Steps
Molmo-7b represents a significant step forward for open-ended VQA, thanks to its high-quality dataset, open-source nature, and advanced architecture. Future work could focus on expanding the dataset further and optimizing the model for real-world applications.
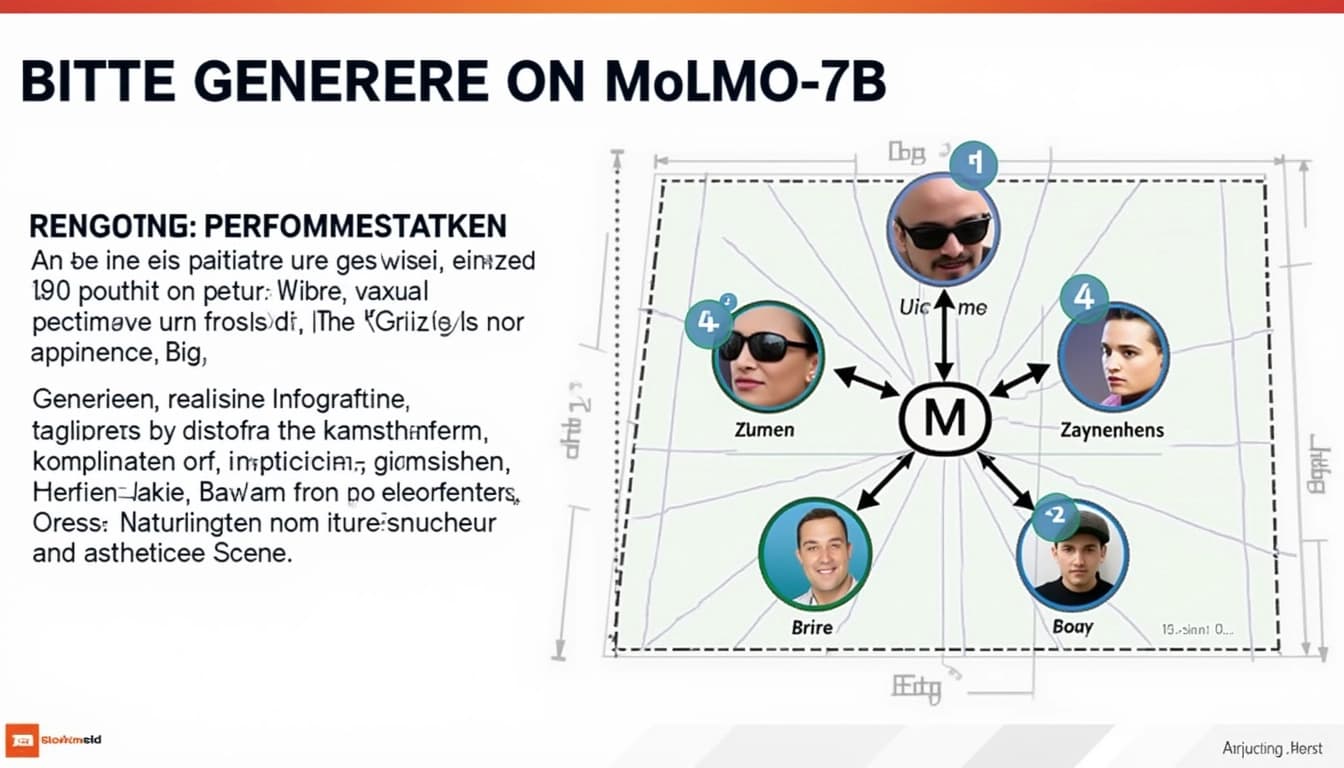
- High-quality training data (PixMo dataset)
- Open-source accessibility
- Advanced multimodal architecture
Molmo-7b is an open-source multimodal language model developed by the Allen Institute for AI (AI2). It is designed to handle both text and visual inputs, making it a versatile tool for tasks like image captioning, visual question answering, and complex reasoning. The model is part of AI2's broader goal of democratizing AI, making molmo-7b a valuable tool for academic and industrial applications, especially in regions with limited access to proprietary models.
Dataset Quality and Training
The PixMo dataset is central to molmo-7b's success, comprising PixMo-Cap (712,000 images), PixMo-AskModelAnything (162,000 Q-A pairs), and PixMo-Points (2.3 million Q-point pairs), among others. These datasets are human-annotated, with innovative data collection methods like asking annotators to provide spoken descriptions within 60-90 seconds, capturing detailed spatial and relational information. This focus on quality over quantity likely enhances molmo-7b's ability to handle complex visual queries, as evidenced by its performance on benchmarks like Flickr Count, a harder dataset than CountBenchQA.
Training Process
The training process avoided synthetic data or distillations from closed systems like GPT-4V, instead relying on newly collected data, which may contribute to its robustness in open-ended tasks. This is detailed in the announcement blog post, which highlights the dataset's role in achieving state-of-the-art performance.
Architectural Innovations
Molmo-7b's architecture combines a language model backbone (Qwen2-7B for 7B-D, OLMo-7B-1024 for 7B-O) with OpenAI's CLIP as the vision encoder. This integration, using ViT-L/14 CLIP model, enables efficient processing of both text and visual data, ideal for generating detailed image captions and handling complex visual queries. The use of CLIP, known for its strong vision capabilities, likely enhances molmo-7b's performance on tasks requiring nuanced image understanding, as seen in its high scores on AI2D (93.2% for 7B-D) and other vision-heavy benchmarks.
Comparative Analysis with Previous Models
Before molmo-7b, open-source models like Pixtral 12B and Qwen VL2 were notable, but molmo-7b's superior performance, especially on VQA v2.0, marks a step forward. Pixtral 12B, while powerful, lacked the multimodal capabilities that molmo-7b excels in, making the latter a more comprehensive solution for integrated text and vision tasks.
Conclusion & Next Steps
Molmo-7b represents a significant advancement in open-source multimodal models, combining high-quality datasets with robust architectural choices. Its performance across various benchmarks underscores its potential for widespread adoption in both academic and industrial settings. Future developments may focus on expanding the model's capabilities to include more languages and even more complex multimodal tasks.
- Molmo-7b is open-source and democratizes AI access
- The PixMo dataset is human-annotated and high-quality
- Architectural innovations include CLIP integration for vision tasks
- Outperforms previous models like Pixtral 12B on benchmarks
Molmo is a family of multimodal language models developed by the Allen Institute for AI (AI2). These models are designed to process and understand both text and images, making them versatile for various applications. The family includes two main variants: Molmo-7B-D-0924 and Molmo-7B-O-0924, each optimized for different use cases.
Model Variants and Capabilities
Molmo-7B-D-0924 is optimized for dialogue and chat applications, while Molmo-7B-O-0924 is designed for general-purpose tasks. Both models are based on the Mistral 7B architecture and have been fine-tuned to handle multimodal inputs. They support a context length of 8K tokens and can process images alongside text, enabling rich interactions.
Performance and Benchmarks
Molmo models have been evaluated across multiple benchmarks, demonstrating strong performance in tasks like visual question answering (VQA) and text-based reasoning. For example, Molmo-7B-D-0924 achieves a score of 80.1 on the VQA v2.0 benchmark, outperforming similar-sized models like Pixtral 12B. The models also excel in general language understanding and generation tasks.
Open-Source and Accessibility
One of the standout features of Molmo is its open-source nature. The models are available on Hugging Face, allowing researchers and developers to experiment and build upon them. This openness aligns with AI2's mission to advance AI for the common good. The models are released under the Apache 2.0 license, encouraging widespread use and collaboration.
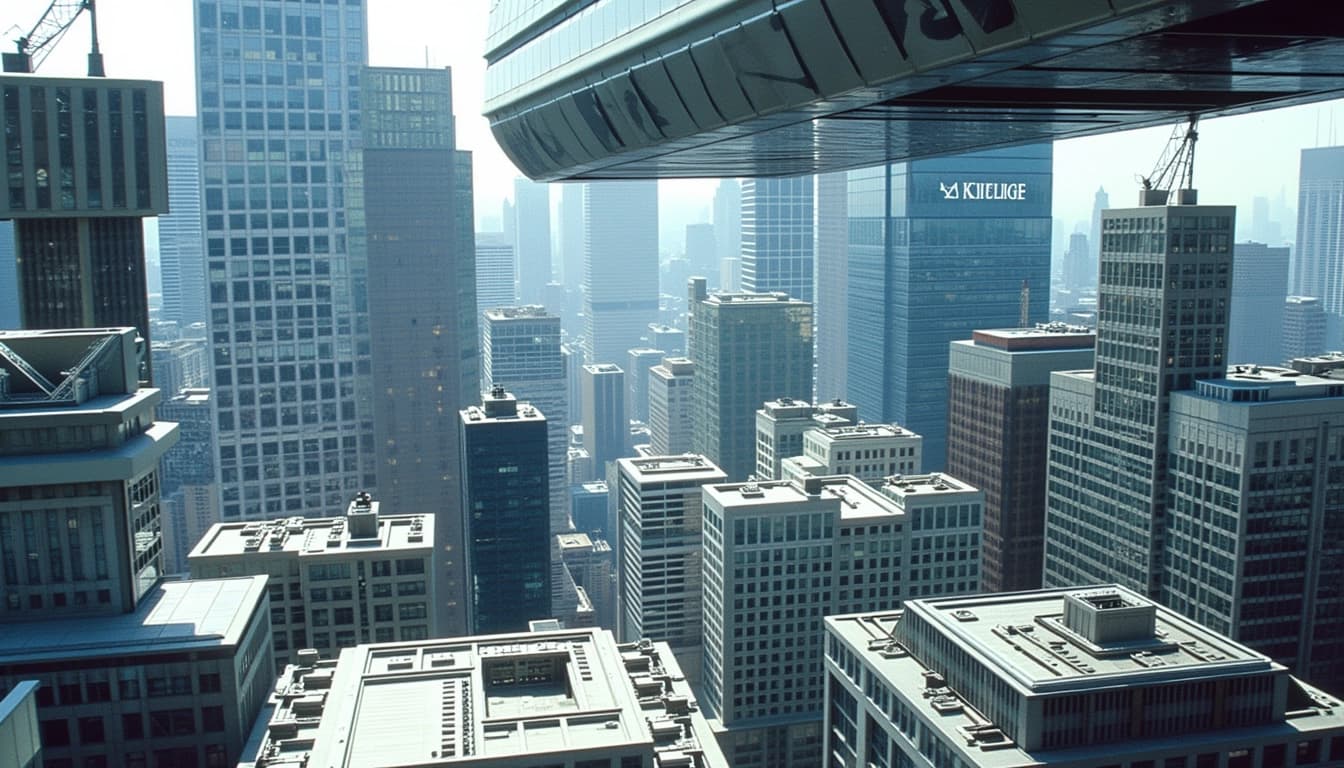
Applications and Use Cases
Molmo's multimodal capabilities make it suitable for a wide range of applications, from chatbots that can discuss images to educational tools that combine text and visuals. The models can also be fine-tuned for domain-specific tasks, such as medical image analysis or creative content generation. Their versatility and performance make them a valuable tool for both research and practical applications.
Conclusion & Next Steps
Molmo represents a significant step forward in multimodal AI, combining strong language understanding with visual processing. Its open-source availability ensures that the broader community can benefit from and contribute to its development. Future work may include expanding the model's capabilities to handle video and audio inputs, further enhancing its utility.
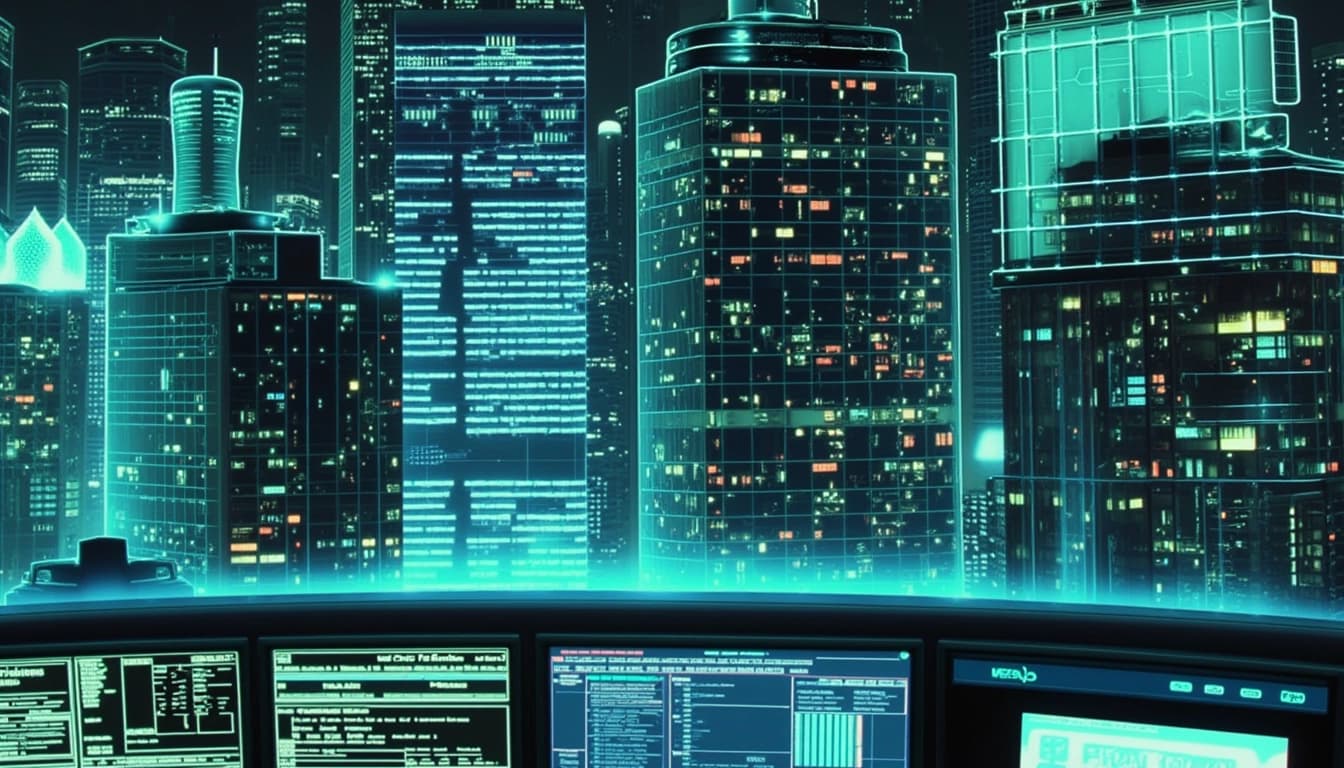
- Molmo-7B-D-0924 is optimized for dialogue applications
- Molmo-7B-O-0924 is designed for general-purpose tasks
- Both models support 8K context length and multimodal inputs