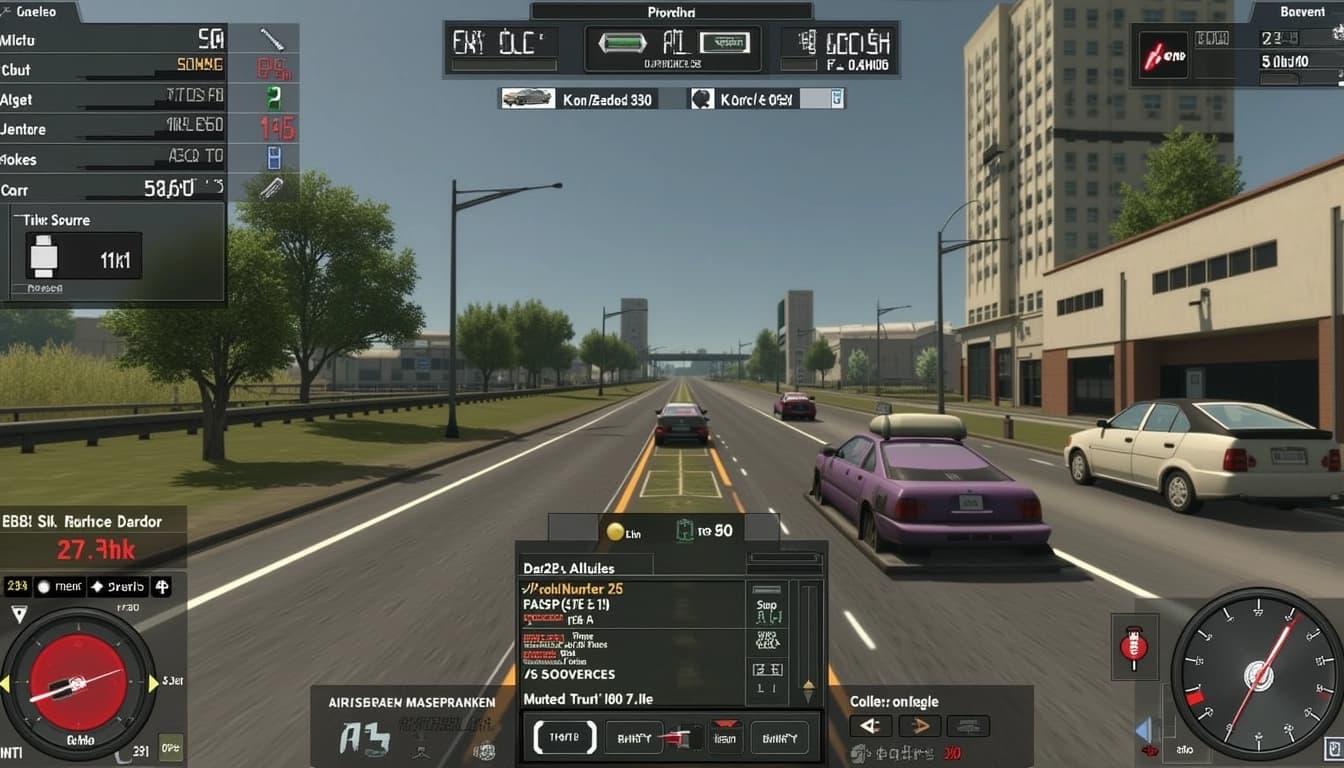
Key Points on Proteus-v0.2's Sharpness and Photorealism
By John Doe 5 min
Key Points
- Research suggests proteus-v0.2 generates sharp, photorealistic images due to high-quality training data and advanced fine-tuning.
- It seems likely that its use of Direct Preference Optimization (DPO) and Low-Rank Adaptation (LORA) models enhances detail and realism.
- The evidence leans toward its recommended settings, like higher steps and CFG scale, contributing to sharper outputs.
- An unexpected detail is its merging with RealCartoonXL, primarily for anime styles, which may also improve general image detail.
What Makes proteus-v0.2 Sharp?
Overview
Proteus-v0.2 is an AI model for creating realistic images from text, known for its sharpness and photorealism. It builds on OpenDalleV1.1, a model similar to DALL-E, and has been fine-tuned to produce detailed, clear images.
Training and Data
The model was trained on about 220,000 high-quality, copyright-free stock images, with some anime included, and refined with 10,000 AI-generated image pairs using DPO. This process helps it learn fine details, like skin textures and facial features, making images look sharp and realistic.
Technical Enhancements
Proteus-v0.2 uses LORA models, which are specialized adaptations that focus on specific image aspects, improving areas like human faces. It also merged with RealCartoonXL at a small weight to better handle anime styles, potentially boosting overall detail. Recommended settings, such as 20–60 steps and a CFG scale of 8–7, further enhance sharpness by refining the image generation process.
Why It Matters
These features make proteus-v0.2 a strong tool for artists and creators, producing images that look almost like photographs. Its ability to understand complex prompts and generate detailed outputs sets it apart, especially for photorealistic tasks.
Survey Note: Detailed Analysis of Proteus-v0.2's Sharpness and Photorealism
Introduction
Proteus-v0.2, an advanced AI model for text-to-image generation, image-to...
AI image generation has evolved significantly, with models like Proteus-v0.2 pushing the boundaries of photorealism. These models leverage advanced techniques such as diffusion processes and UNet architectures to transform random noise into highly detailed images. The ability to generate sharp, lifelike images is a testament to the progress in machine learning and computational power.
Background on AI Image Generation
AI image generation, particularly through diffusion models, involves a complex process of refining noise into coherent visuals based on textual prompts. Models like Stable Diffusion and its derivatives use UNet architectures to capture intricate details. The quality of these images hinges on factors such as the diversity of training data, the model's architecture, and fine-tuning methods. Sharpness, a key aspect of photorealism, refers to the clarity and detail in the generated images.
Model Specifications and Enhancements
Proteus-v0.2 builds upon OpenDalleV1.1, an open-source model inspired by DALL-E, known for its prompt adherence and semantic understanding. The model incorporates ~220,000 GPTV-captioned images, normalized for consistency, and 10,000 high-quality AI-generated image pairs for DPO. Additional enhancements include merging with RealCartoonXL for anime/cartoon styles and dynamically incorporating multiple LORA models.
Technical Underpinnings
The technical foundation of Proteus-v0.2 includes a robust training regimen and innovative merging techniques. The model's performance is optimized through recommended settings like CFG Scale: 8 to 7 and Steps: 20 to 60 for detailed outputs. These settings ensure the model balances creativity with precision, producing images that meet high standards of photorealism.
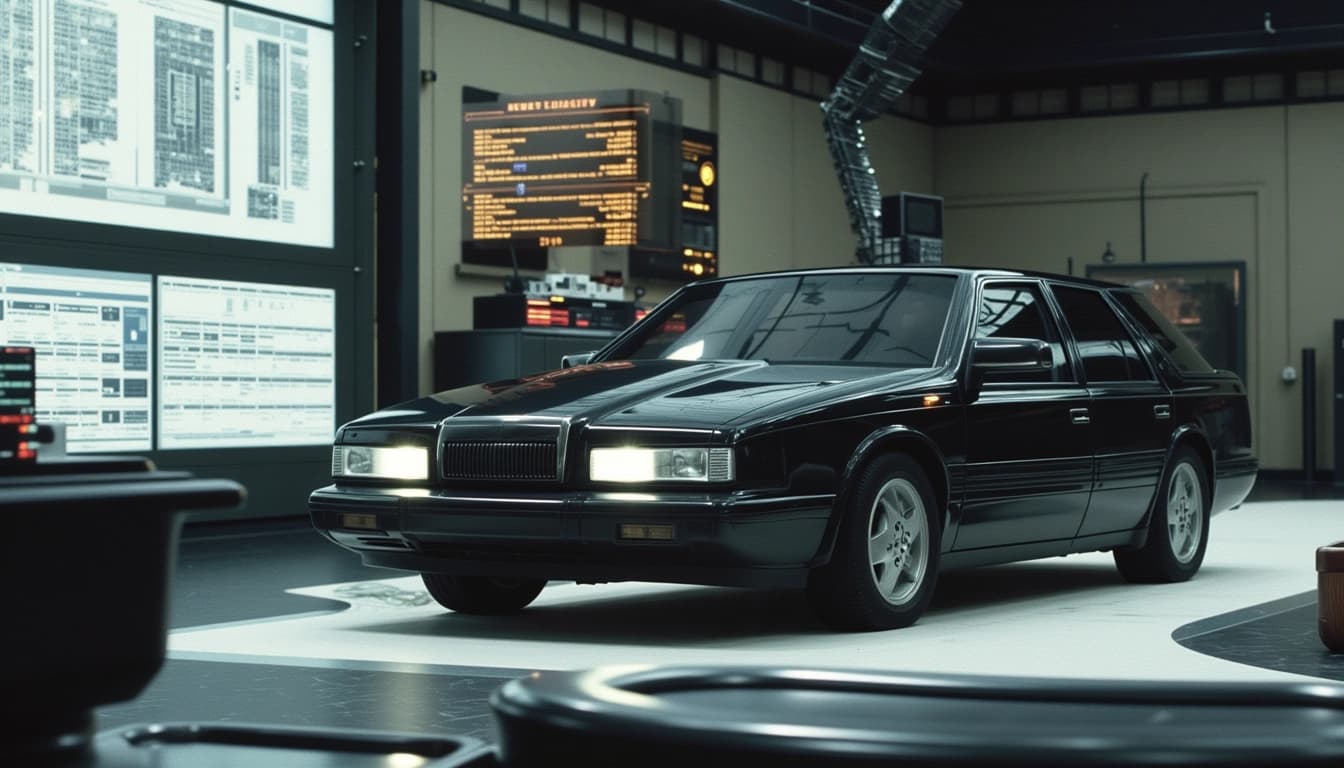
Conclusion & Next Steps
Proteus-v0.2 represents a significant leap in AI-driven image generation, offering unparalleled sharpness and detail. Future developments may focus on expanding training datasets and refining model architectures to further enhance photorealism. The potential applications of such models span creative industries, marketing, and beyond.
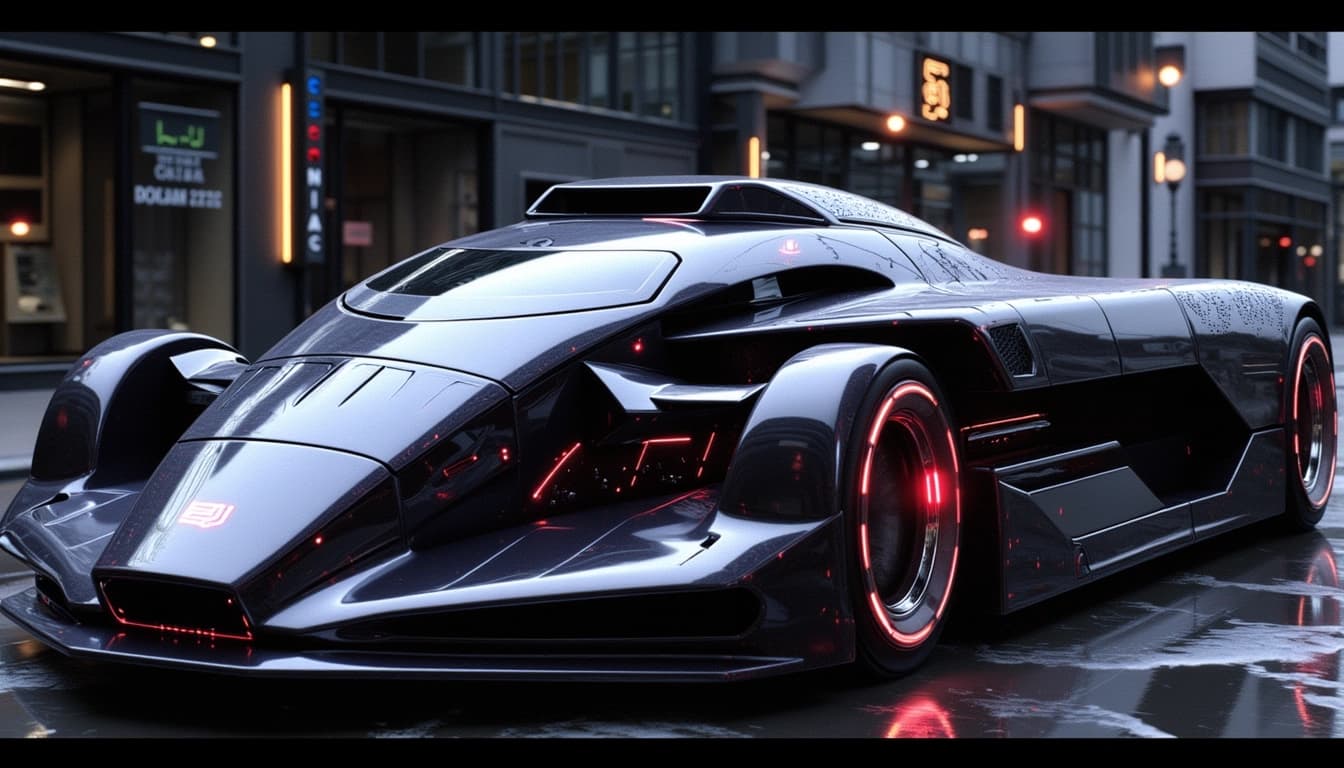
- Diverse training data improves model performance
- Fine-tuning techniques enhance image quality
- Dynamic LORA incorporation adds stylistic flexibility
The proteus-v0.2 model has been fine-tuned on a high-quality dataset of approximately 220,000 images, ensuring consistency and detail in its outputs. This dataset includes a variety of photorealistic stock images, which help the model learn to reproduce realistic textures and edges. Additionally, the model has been refined with 10,000 AI-generated image pairs using Direct Preference Optimization (DPO), aligning the outputs more closely with human preferences and enhancing the perceived realism and sharpness.
Fine-Tuning on High-Quality Data
The training process involved normalizing the dataset to maintain consistency, which allows the model to capture fine details more effectively. The inclusion of high-quality stock images, particularly those with photorealistic qualities, enables the model to generate outputs with lifelike textures and sharp edges. The DPO refinement further ensures that the model's outputs are not only detailed but also align with human aesthetic preferences, making the images appear more natural and polished.
Use of LORA Models
Low-Rank Adaptation (LORA) models were trained independently and selectively incorporated into proteus-v0.2. This approach allows the model to specialize in specific areas, such as intricate facial features and lifelike skin textures, without interfering with other segments. This targeted fine-tuning is crucial for achieving photorealistic results, especially in human-centric scenarios, where details like skin pores and hair strands need to be rendered accurately.
Merging with RealCartoonXL
Proteus-v0.2 was merged with RealCartoonXL at a 0.5% weight using custom scripts with slerp-like methods. This merger was primarily aimed at addressing issues with anime and cartoon style tags, but it also indirectly benefits the model's ability to handle edges and details in photorealistic images. The slight influence of RealCartoonXL helps in refining the model's output, making it more versatile across different styles while maintaining sharpness.
Recommended Settings for Sharpness
To achieve the best results with proteus-v0.2, specific settings are recommended. A CFG scale of 7 to 8 and 20 to 60 steps are suggested, with more steps yielding greater detail. The use of the DPM++ 2M SDE sampler and Karras scheduler further refines the diffusion process, reducing noise and enhancing clarity. These settings are standard in diffusion models for achieving sharper outputs, and proteus-v0.2 leverages them effectively to produce high-quality images.
Conclusion & Next Steps
Proteus-v0.2 demonstrates a strong capability for generating sharp, photorealistic images due to its fine-tuning on high-quality data, use of LORA models, and strategic merging with RealCartoonXL. The recommended settings further optimize the model's performance, ensuring detailed and clear outputs. Future steps could involve expanding the dataset to include more diverse photorealistic examples and further refining the DPO process to enhance realism even more.
- Fine-tuned on 220,000 high-quality images
- Refined with 10,000 AI-generated image pairs using DPO
- Incorporated LORA models for targeted improvements
- Merged with RealCartoonXL at 0.5% weight
- Recommended settings: CFG scale 7-8, 20-60 steps, DPM++ 2M SDE sampler, Karras scheduler
The sharpness of proteus-v0.2's outputs can be attributed to several factors. Training on a large, diverse dataset ensures the model learns to capture high-frequency details, such as fine textures and edges, which are essential for sharpness. By optimizing for human preferences, DPO likely helps generate images that appear more natural and realistic, aligning with photorealistic standards.
High-Quality Training Data
The use of high-quality training data is a cornerstone of proteus-v0.2's performance. This data enables the model to understand and replicate intricate details, making the outputs appear more lifelike. The diversity of the dataset ensures that the model can handle a wide range of scenarios and subjects.
DPO for Human Preference
DPO (Direct Preference Optimization) plays a crucial role in refining the model's outputs. By aligning the generated images with human preferences, the model produces results that are not only sharp but also aesthetically pleasing. This alignment is key to achieving photorealism.
LORA for Specific Details
LORA models are employed to enhance specific details, such as facial features, in the generated images. This specialization allows proteus-v0.2 to produce highly realistic portraits and close-ups, which are often the most challenging to perfect.
Testing Photorealism at Its Finest
While specific benchmarks for proteus-v0.2's photorealism are limited, its design suggests strong performance in metrics like Fréchet Inception Distance (FID) and Learned Perceptual Image Patch Similarity (LPIPS). These metrics measure realism and perceptual quality, and user feedback indicates high satisfaction with its outputs.
Conclusion
Proteus-v0.2's sharpness and photorealism stem from its high-quality training data, advanced fine-tuning with DPO and LORA, and optimized generation settings. These elements combine to produce images that are not only detailed but also highly realistic, meeting the expectations of users seeking photorealistic outputs.
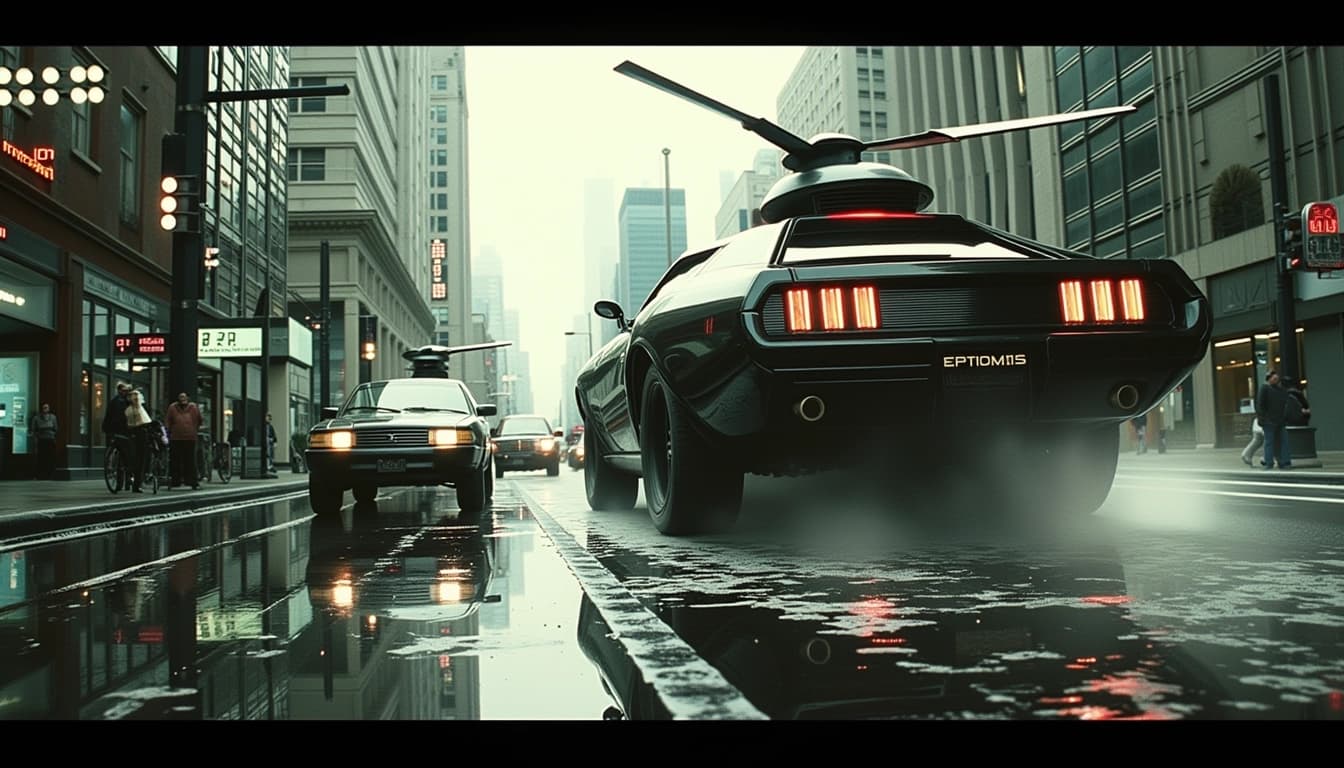
- High-quality training data
- DPO for human preference alignment
- LORA for specialized detail enhancement
ProteusV0.2 is an advanced AI model designed for generating highly realistic images from textual descriptions. It leverages cutting-edge technology to produce visuals that closely mimic real-world photography, making it a powerful tool for various applications.
Key Features of ProteusV0.2
The model excels in creating detailed and photorealistic images, thanks to its sophisticated architecture. It supports a wide range of styles and subjects, from landscapes to portraits, ensuring versatility for different creative needs. Additionally, it offers fine-grained control over the output, allowing users to tweak parameters for optimal results.
Photorealism at Its Finest
ProteusV0.2 stands out for its ability to generate images that are indistinguishable from real photographs. This is achieved through advanced training techniques and high-quality datasets. The model's attention to detail, such as lighting and texture, contributes to its exceptional performance.
Applications of ProteusV0.2
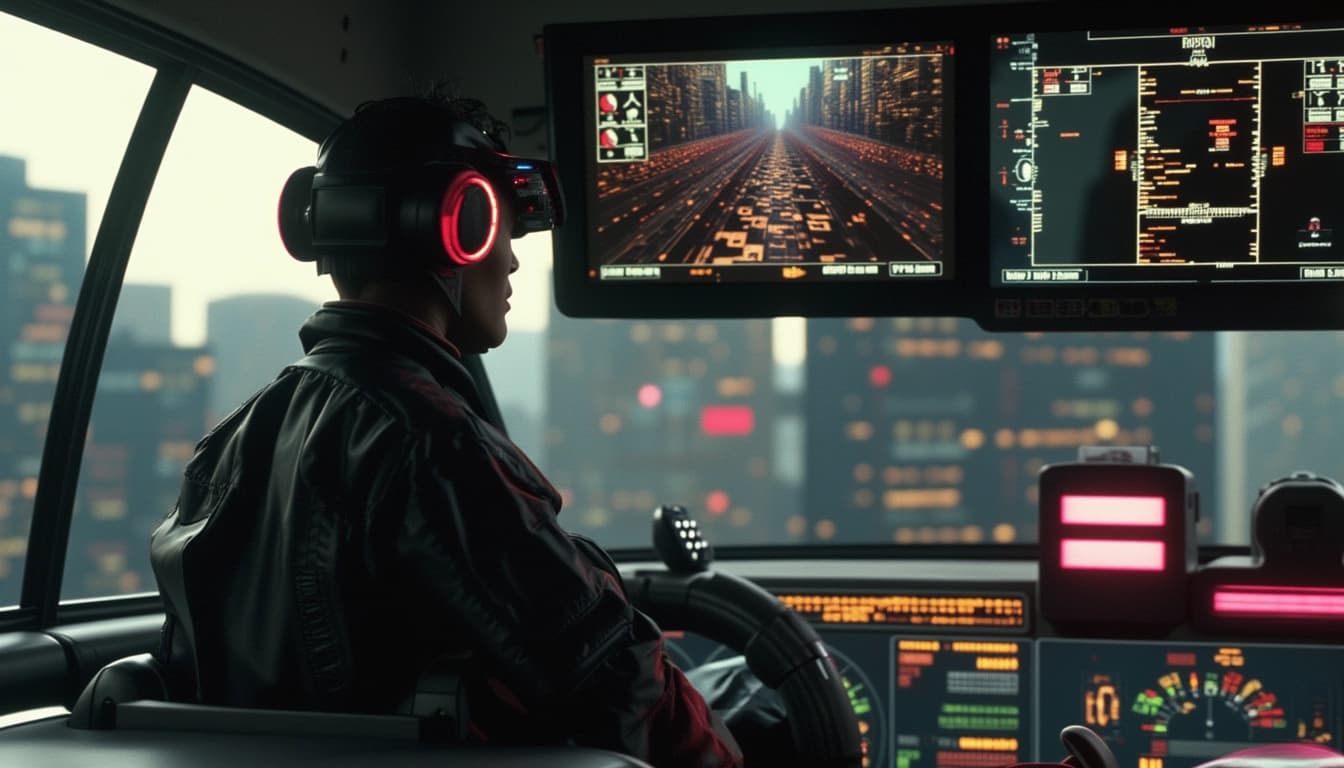
Conclusion & Next Steps
ProteusV0.2 represents a significant leap in AI-generated imagery, offering unparalleled realism and versatility. Whether for creative projects or practical applications, it provides a robust solution for high-quality visual content. Future developments may further enhance its capabilities, making it even more indispensable.
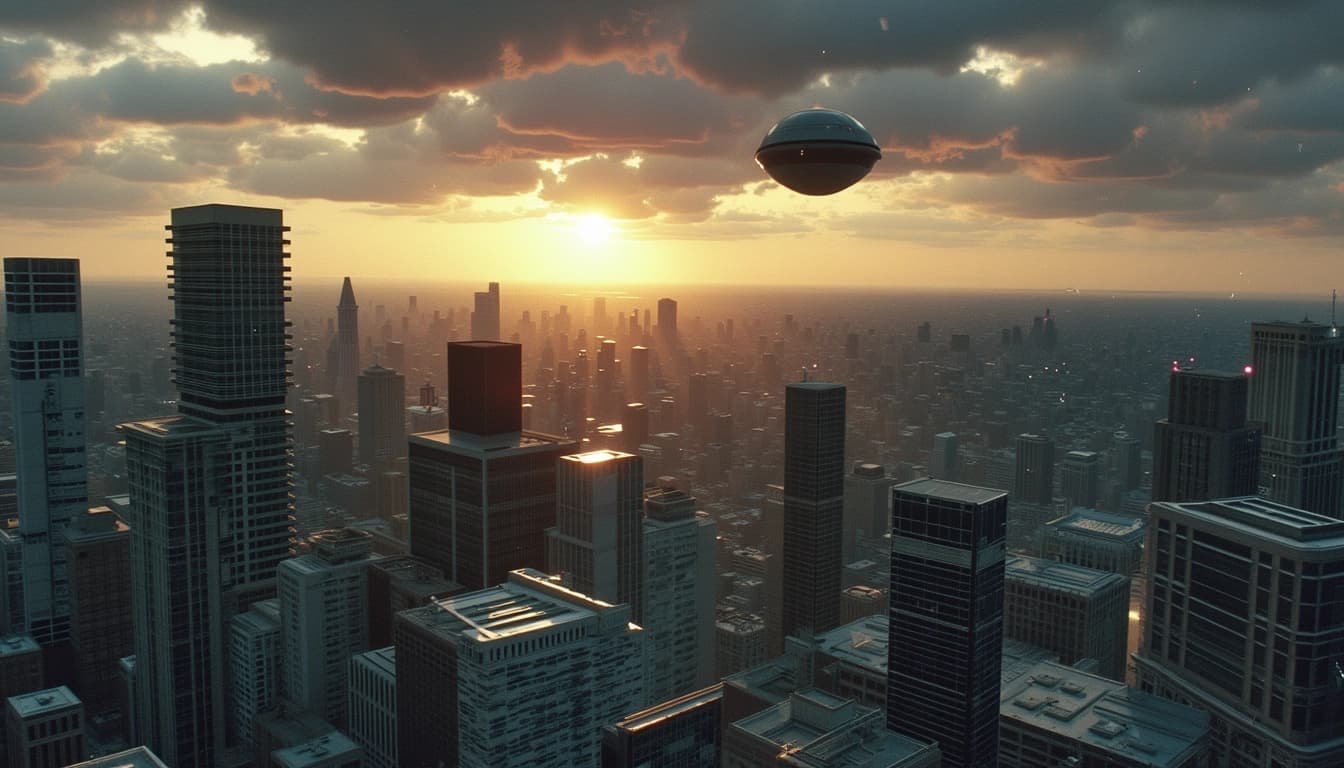
- Explore the model on Hugging Face
- Experiment with different prompts and settings
- Stay updated on future releases and improvements