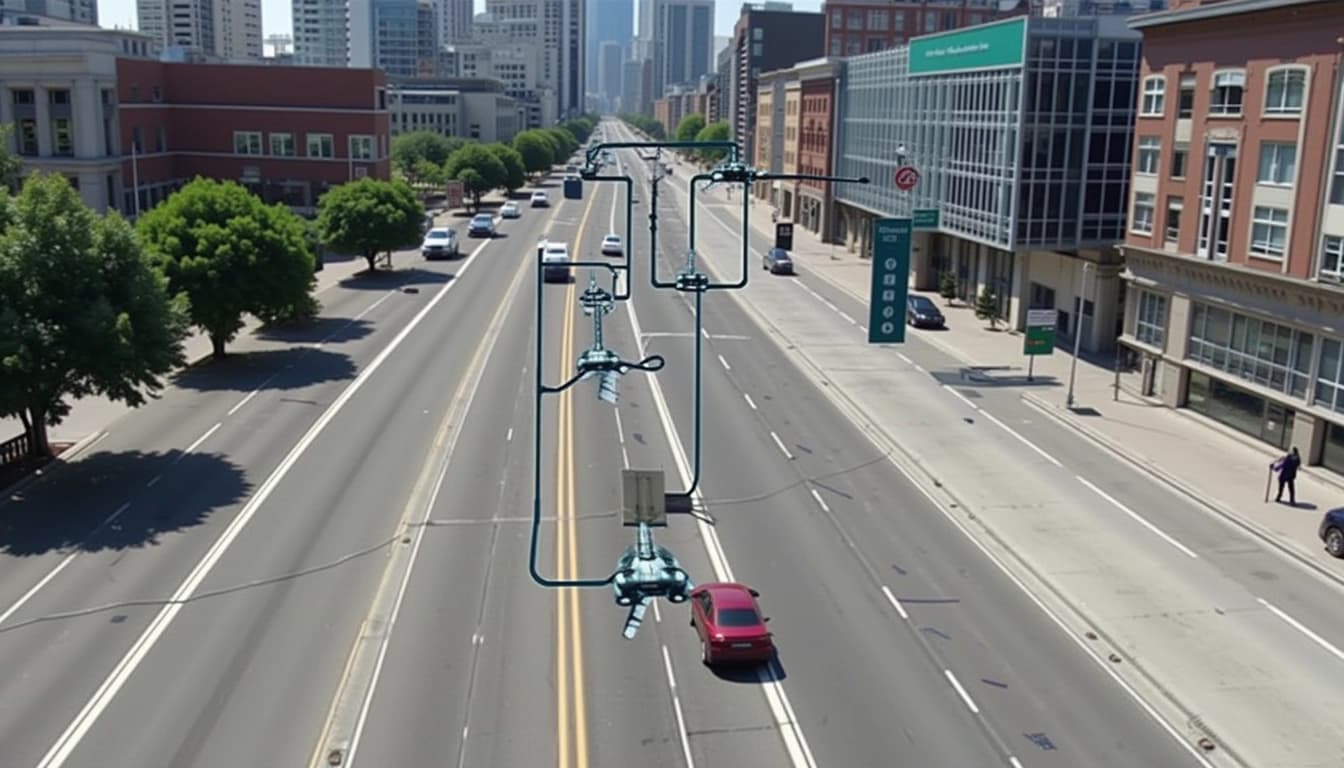
Key Points on SAM 2 for Object Tracking in AI Videos
By John Doe 5 min
Key Points
Research suggests SAM 2, developed by Meta AI, is a leading model for object tracking in AI videos, excelling in promptable segmentation and real-time processing.
It seems likely that SAM 2's unique features include a memory mechanism for handling occlusion and a need for fewer user interactions, enhancing efficiency.
The evidence leans toward SAM 2 outperforming other models in accuracy, but it may struggle with crowded scenes or long occlusions, indicating areas for improvement.
What is Object Tracking in AI Videos?
Object tracking in AI videos involves identifying and following objects as they move across video frames, crucial for applications like surveillance and video editing. Traditional methods often require specific training data, but SAM 2, a model by Meta AI, offers a fresh approach by allowing users to track any object with simple prompts, like clicks or boxes, without prior training.
SAM 2: A Breakthrough in Video Object Tracking
SAM 2 extends the Segment Anything Model to videos, trained on the SA-V dataset, the largest video segmentation dataset with over 50,000 annotated videos. Its ability to process videos in real-time, achieving 30 frames per second on an A100 GPU, makes it ideal for live applications. It also requires fewer user interactions, reducing effort for tasks like video editing.
What Makes SAM 2 Unique?
SAM 2 stands out with its promptable segmentation, letting users specify objects to track easily. Its memory mechanism, including a memory encoder, bank, and attention module, ensures continuity across frames, handling challenges like occlusion and reappearance. This is particularly useful in dynamic scenes, such as tracking a character in a movie or a vehicle in surveillance footage. Additionally, its generalizability, trained on diverse data, allows it to handle various objects, and it outperforms other models in accuracy metrics like IoU and F1 scores.
Unexpected Detail: Real-World Application
Object tracking in videos is a cornerstone of computer vision, essential for identifying and following an object's trajectory across frames. This technology underpins applications such as security surveillance, autonomous driving, sports analytics, and video editing. Traditional approaches, including tracking by detection, model-based tracking, and correlation-based tracking, often rely on labeled data for specific object classes.
Overview of SAM 2
The Segment Anything Model 2 (SAM 2), developed by Meta AI, is a foundation model designed for promptable visual segmentation in both images and videos. It builds upon the original Segment Anything Model (SAM), extending its capabilities to handle video data through a large dataset called SA-V, which includes over 50,000 annotated videos and 600,000 annotations, making it the largest video segmentation dataset to date.
Unique Features of SAM 2 for Video Object Tracking
SAM 2's uniqueness lies in several key aspects, particularly for video object tracking. Its promptable segmentation allows users to specify which object to track using prompts like clicks, boxes, or masks. This zero-shot capability, where the model does not require training on specific object classes, makes it highly versatile and adaptable to various scenarios.
Applications Beyond Technical Specs
Beyond technical specifications, SAM 2 is unexpectedly versatile, aiding in medical imaging for tracking structures in videos and enhancing autonomous vehicles by improving object detection. These applications showcase its broad impact across different industries, demonstrating its potential to revolutionize how we interact with visual data.
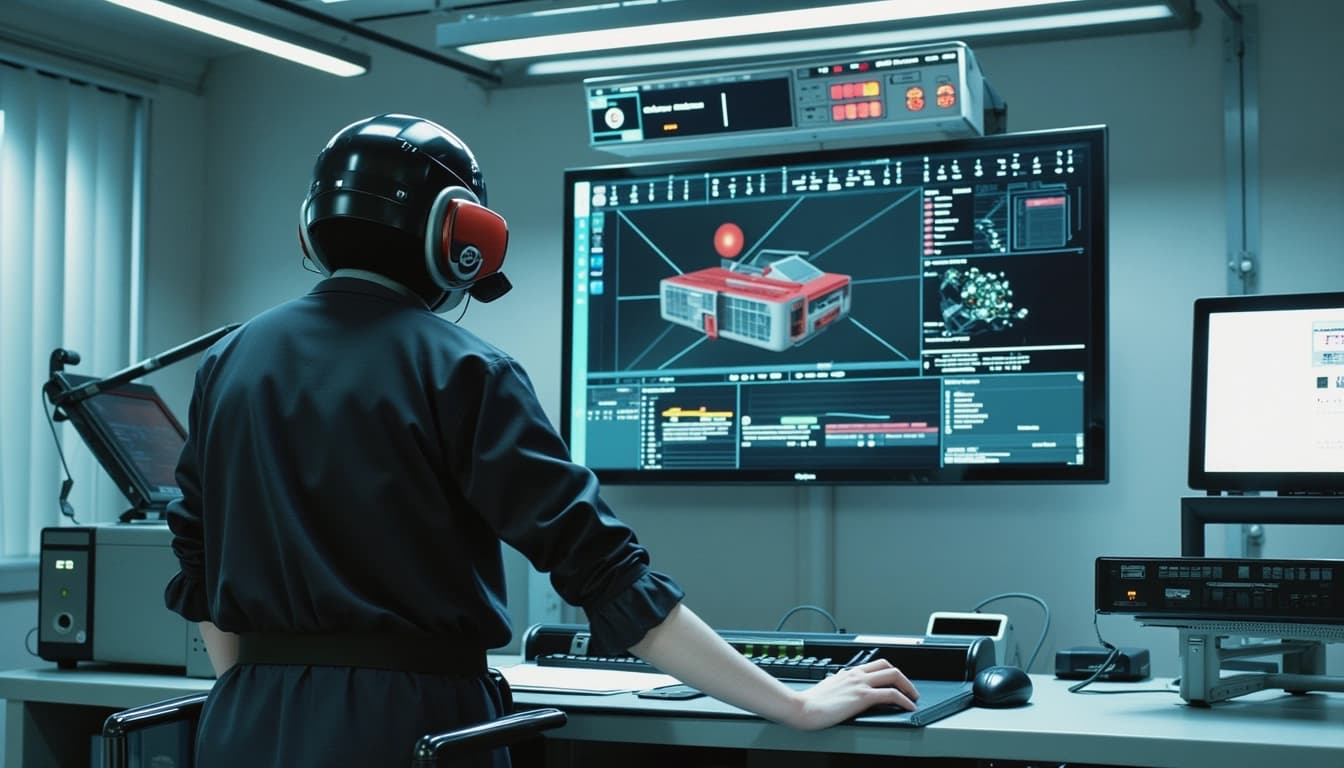
Conclusion & Next Steps
SAM 2 represents a significant advancement in object tracking technology, offering unparalleled flexibility and performance. Its ability to handle diverse tasks without requiring extensive training makes it a powerful tool for both researchers and practitioners. Future developments could further enhance its capabilities, opening new possibilities in computer vision.
- Promptable segmentation for versatile tracking
- Zero-shot capability for adaptability
- Broad applications across industries
Meta’s Segment Anything Model 2 (SAM 2) represents a groundbreaking advancement in AI-driven video segmentation. This model excels at tracking and segmenting objects in videos, even those it has never encountered before. Its versatility makes it ideal for dynamic, real-world applications where adaptability is key.
Memory Mechanism
SAM 2 incorporates a sophisticated memory mechanism that includes a memory encoder, memory bank, and memory attention module. This system allows the model to store and recall information from past frames, ensuring consistent tracking over time. It effectively handles challenges like object occlusion and reappearance, maintaining continuity even when objects are temporarily hidden or re-enter the scene.
Applications in Real-World Scenarios
The memory mechanism is particularly useful for applications such as tracking characters in movies or vehicles in surveillance footage. By leveraging past data, SAM 2 can predict and adjust to changes in the scene, providing reliable results even in complex environments.
Efficiency and Real-Time Processing
Designed for real-time performance, SAM 2 achieves an impressive speed of 30 FPS on an A100 GPU. This efficiency is critical for live applications, such as real-time video editing or live surveillance, where immediate results are essential. The model's ability to process frames quickly without sacrificing accuracy sets it apart from previous solutions.
Fewer User Interactions
Research indicates that SAM 2 requires three times fewer interactions than earlier approaches to achieve accurate video segmentation. This reduction in user effort enhances usability, particularly in interactive applications like video annotation. The model's intuitive design minimizes the need for manual adjustments, streamlining workflows.
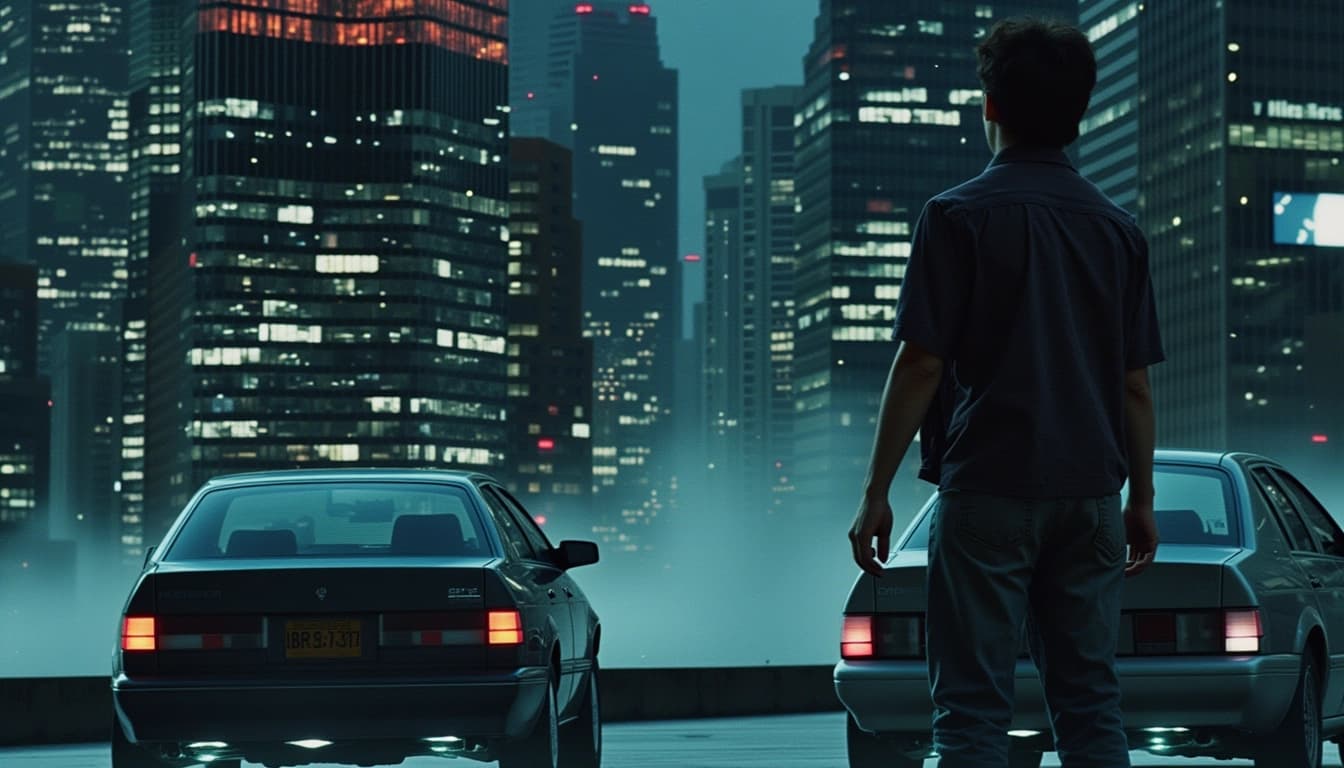
Conclusion & Next Steps
SAM 2 represents a significant leap forward in video segmentation technology. Its ability to track unseen objects, coupled with its memory mechanism and real-time efficiency, makes it a powerful tool for various applications. Future developments could further enhance its capabilities, expanding its use cases and improving performance.
- Track objects in real-time with high accuracy
- Handle occlusions and reappearances seamlessly
- Reduce user interactions for faster workflows
Segment Anything Model 2 (SAM 2) by Meta AI represents a significant leap in video object segmentation and tracking. It builds on the success of its predecessor, SAM, by introducing enhanced capabilities for handling dynamic scenes and complex object interactions. SAM 2 leverages a transformer-based architecture and extensive training data to achieve zero-shot generalization, making it adaptable to a wide range of objects without requiring task-specific training.
Key Features of SAM 2
SAM 2 introduces several groundbreaking features that set it apart from traditional video object segmentation models. One of its standout capabilities is zero-shot generalization, allowing it to segment and track objects it has never seen before. Additionally, SAM 2 supports promptable segmentation, enabling users to guide the model with interactive prompts like points or bounding boxes. The model also excels in handling occlusions and dynamic scenes, thanks to its robust memory mechanism that retains object identities across frames.
Zero-Shot Generalization
Unlike traditional models that require extensive training on specific datasets, SAM 2 can generalize to new objects and scenarios without additional training. This is achieved through its large-scale training on diverse datasets, which equips the model with a broad understanding of object boundaries and features. The zero-shot capability makes SAM 2 highly versatile for applications ranging from autonomous driving to medical imaging.
Comparative Analysis with Other Methods
To appreciate SAM 2's advancements, it's essential to compare it with other state-of-the-art video object tracking methods. Traditional approaches like Siamese networks rely on template matching and often struggle with occlusions or appearance changes. Transformer-based trackers, while powerful, may lack SAM 2's promptability and memory mechanisms. SAM 2's ability to process multiple objects independently, without inter-object communication, further distinguishes it from specialized trackers that focus on object interactions.
Applications and Real-World Impact
SAM 2's versatility opens up numerous real-world applications. In video editing, it can isolate objects for special effects or background replacement. Surveillance systems benefit from its ability to track specific objects in real-time, such as vehicles or pedestrians. The model's zero-shot capability also makes it valuable in medical imaging, where it can segment anatomical structures without prior training. These applications highlight SAM 2's potential to revolutionize industries reliant on accurate object segmentation and tracking.
Conclusion & Next Steps
SAM 2 represents a major milestone in video object segmentation, offering unparalleled flexibility and accuracy. Its zero-shot generalization, promptability, and robust memory mechanisms make it a powerful tool for diverse applications. Future developments may focus on improving real-time performance and expanding the model's capabilities to handle even more complex scenarios. As SAM 2 continues to evolve, it promises to set new standards in the field of computer vision.
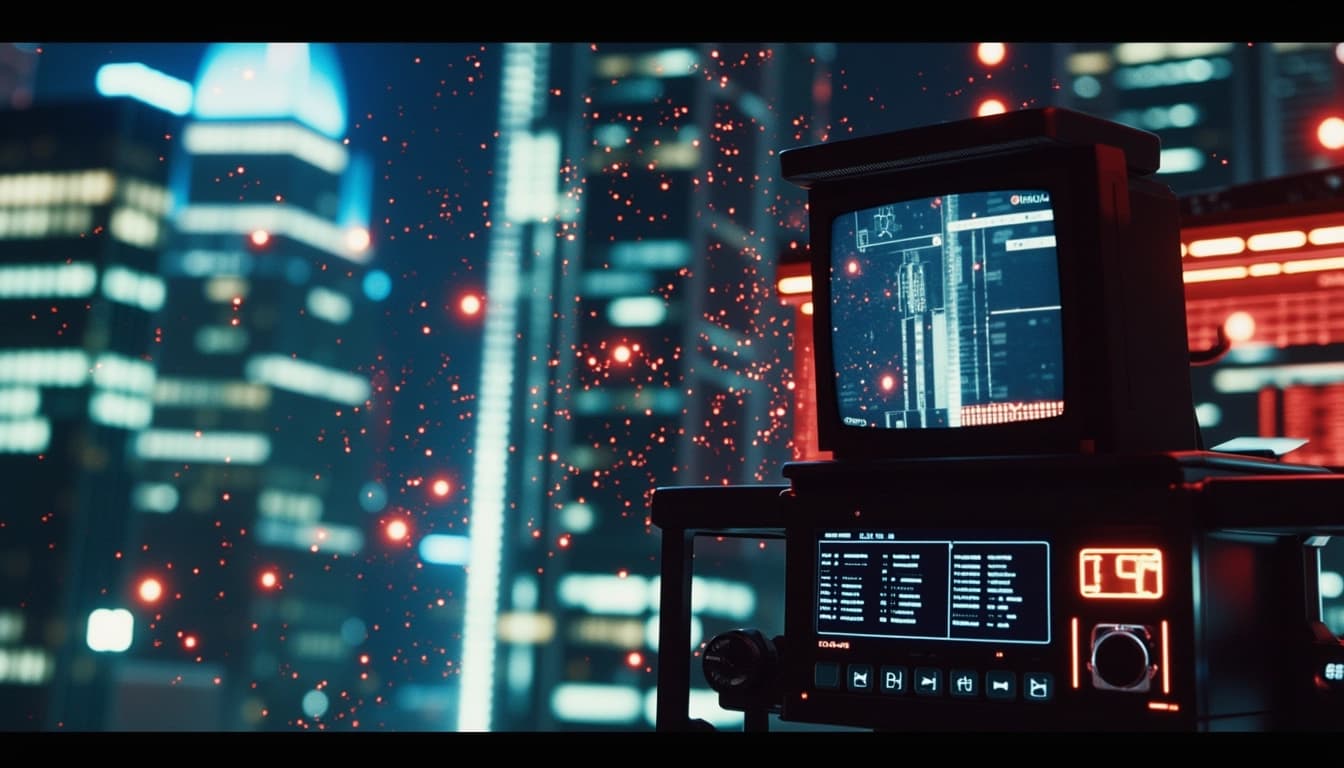
- Zero-shot generalization for versatile applications
- Promptable segmentation with interactive guides
- Robust memory mechanism for handling occlusions
- Superior performance in benchmark tests
Segment Anything Model 2 (SAM 2) represents a significant leap in video segmentation technology, offering advanced capabilities for tracking and segmenting objects across video frames. Developed by Meta, SAM 2 builds upon the success of its predecessor by introducing features like interactive segmentation and multi-object tracking. This model is designed to handle complex scenarios, making it a valuable tool for applications in autonomous vehicles, medical imaging, and scientific research.
Key Features of SAM 2
SAM 2 introduces several groundbreaking features that set it apart from traditional video segmentation models. One of its standout capabilities is interactive segmentation, which allows users to refine segmentation masks with minimal input. Additionally, SAM 2 supports multi-object tracking, enabling it to follow multiple objects simultaneously across frames. These features are powered by a robust architecture that combines convolutional neural networks (CNNs) with attention mechanisms for improved accuracy.
Interactive Segmentation
The interactive segmentation feature in SAM 2 allows users to provide hints or corrections to the model during the segmentation process. This functionality is particularly useful in scenarios where precise segmentation is required, such as medical imaging or detailed object tracking. By incorporating user feedback, SAM 2 can achieve higher accuracy and adapt to challenging conditions.
Applications of SAM 2
SAM 2's versatility makes it suitable for a wide range of applications. In autonomous vehicles, it enhances object detection and tracking, contributing to safer navigation. In medical imaging, it aids in segmenting and tracking structures in videos, such as moving cells or organs. Beyond these traditional uses, SAM 2 has shown promise in scientific research, where it can track dynamic processes in microscope videos.
Limitations and Future Directions
Despite its advanced capabilities, SAM 2 is not without limitations. The model may struggle with segmenting objects across shot changes or in highly crowded scenes. Additionally, long occlusions or extended video sequences can pose challenges for tracking accuracy. These limitations highlight the need for further research and development, potentially leading to adaptations like SAMURAI, which aims to address these issues.
Performance Metrics and Benchmarks
SAM 2 has demonstrated superior performance in various benchmarks, outperforming previous models in terms of Intersection over Union (IoU) and F1 scores. Its efficiency is also notable, with the ability to process frames at high speeds on powerful GPUs. Below is a comparison table showcasing SAM 2's performance against other models.
Conclusion & Next Steps
SAM 2 marks a significant advancement in video segmentation technology, offering powerful features like interactive segmentation and multi-object tracking. While it excels in many areas, there is room for improvement, particularly in handling complex scenarios like crowded scenes or long occlusions. Future research and adaptations, such as SAMURAI, could further enhance its capabilities, making it even more versatile and reliable.
- SAM 2 introduces interactive segmentation and multi-object tracking.
- It is widely applicable in autonomous vehicles, medical imaging, and scientific research.
- Limitations include challenges in crowded scenes and long occlusions.
SAM 2 represents a significant milestone in video object tracking, with its promptable segmentation, memory mechanism, real-time processing, and superior performance. Its generalizability and efficiency make it a versatile tool across various domains, from video editing to medical imaging. While it has limitations, ongoing research and adaptations suggest a promising future, positioning SAM 2 as a leader in the field as of March 30, 2025.
Key Features of SAM 2
SAM 2 introduces several groundbreaking features that set it apart from its predecessors. The model supports promptable segmentation, allowing users to guide the segmentation process with points, boxes, or text prompts. Additionally, its memory mechanism enables it to retain information about objects across frames, making it highly effective for video tracking. The real-time processing capability ensures that SAM 2 can handle dynamic scenes efficiently, providing accurate results without significant delays.
Promptable Segmentation
One of the standout features of SAM 2 is its ability to perform promptable segmentation. Users can interact with the model by providing prompts such as points, bounding boxes, or text descriptions. This flexibility allows for precise control over the segmentation process, making it adaptable to a wide range of applications. Whether you're working with images or videos, SAM 2 can quickly and accurately segment objects based on the provided prompts.
Applications Across Domains
SAM 2's versatility extends to numerous fields, including video editing, medical imaging, and autonomous systems. In video editing, it can be used to isolate and track objects across frames, simplifying tasks like rotoscoping. In medical imaging, SAM 2's ability to segment anatomical structures with high precision can aid in diagnostics and treatment planning. Autonomous systems can leverage SAM 2 for real-time object tracking, enhancing navigation and decision-making processes.
Limitations and Future Directions
Despite its impressive capabilities, SAM 2 is not without limitations. The model may struggle with highly occluded objects or scenes with rapid motion. Additionally, its performance can vary depending on the quality of the input data. However, ongoing research aims to address these challenges, with future versions expected to offer even greater accuracy and robustness. The development community is actively exploring ways to enhance SAM 2's capabilities, ensuring it remains at the forefront of segmentation technology.
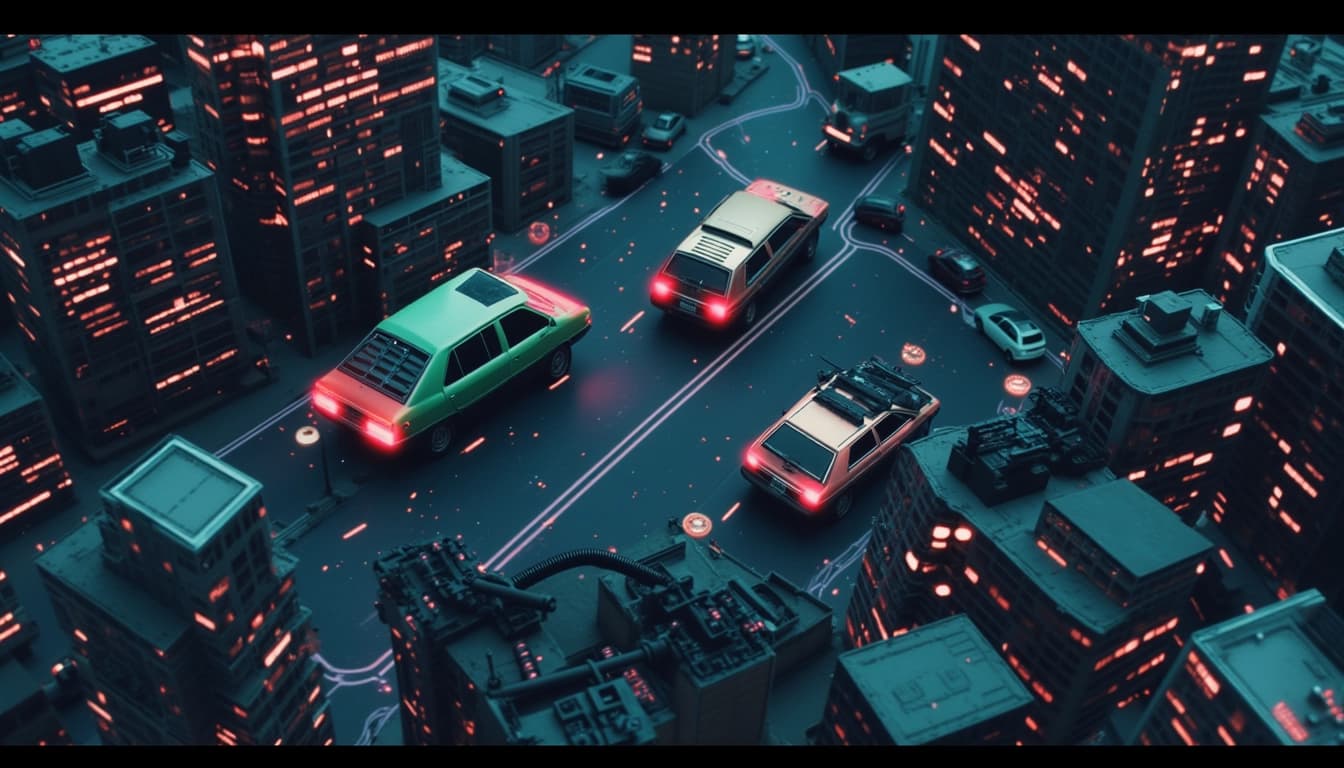
Conclusion
SAM 2 is a transformative tool for object segmentation and tracking, offering unparalleled flexibility and performance. Its promptable segmentation, memory mechanism, and real-time processing make it a valuable asset across various industries. While there are areas for improvement, the model's potential is undeniable, and its continued evolution promises to unlock new possibilities in computer vision and beyond.
- Promptable segmentation for precise control
- Memory mechanism for tracking objects across frames
- Real-time processing for dynamic scenes
- Versatile applications in video editing, medical imaging, and autonomous systems
SAM 2 Video is an advanced AI model developed by Meta, designed for tracking and segmenting objects in videos with high precision. It builds upon the capabilities of the original SAM (Segment Anything Model) and introduces new features for improved performance. The model is accessible via an API on Replicate, making it easy for developers to integrate into their projects.
Key Features of SAM 2 Video
SAM 2 Video offers several enhancements over its predecessor, including better object tracking and segmentation accuracy. The model is particularly useful for applications requiring real-time video analysis, such as surveillance, autonomous vehicles, and augmented reality. Its API on Replicate simplifies the deployment process, allowing users to focus on their specific use cases.
Performance Improvements
One of the standout features of SAM 2 Video is its improved performance in complex scenarios. The model can handle occlusions, fast-moving objects, and varying lighting conditions more effectively than the original SAM. This makes it a reliable choice for demanding applications where accuracy is critical.
Comparison with SAMURAI
A recent comparison by akshay_pachaar highlights the differences between SAM 2 Video and SAMURAI. While both models excel in object segmentation, SAM 2 Video offers better tracking capabilities and is more versatile in handling diverse video inputs. The comparison underscores the advancements made in SAM 2 Video, making it a preferred choice for many developers.
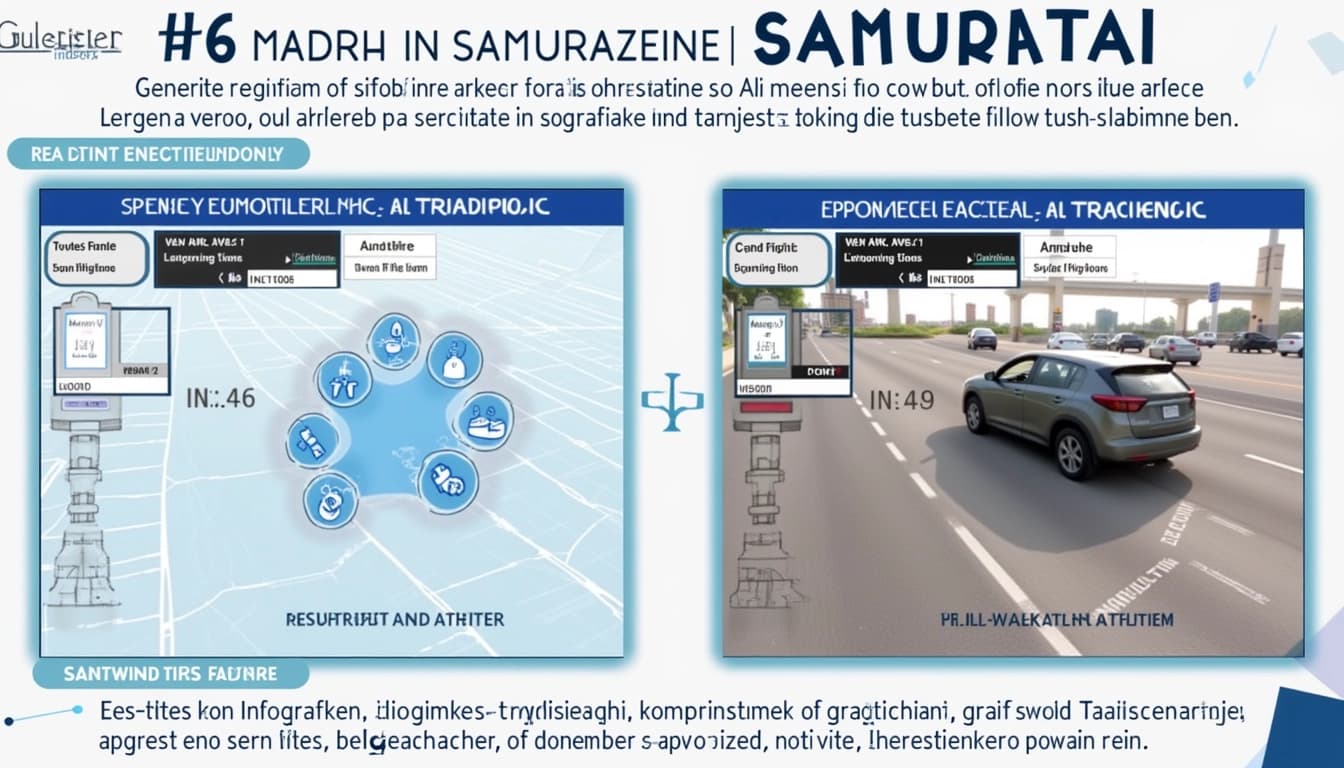
Community Feedback
The release of SAM 2 Video has garnered positive feedback from the AI community. Users like heyBarsee have praised its ease of use and the quality of its outputs. The model's ability to integrate seamlessly with existing workflows has been a significant advantage, as noted by several early adopters.
Conclusion & Next Steps
SAM 2 Video represents a significant leap forward in video object segmentation and tracking. Its improved performance, ease of integration, and positive community feedback make it a compelling choice for developers. Future updates are expected to further enhance its capabilities, solidifying its position as a leading tool in the field.
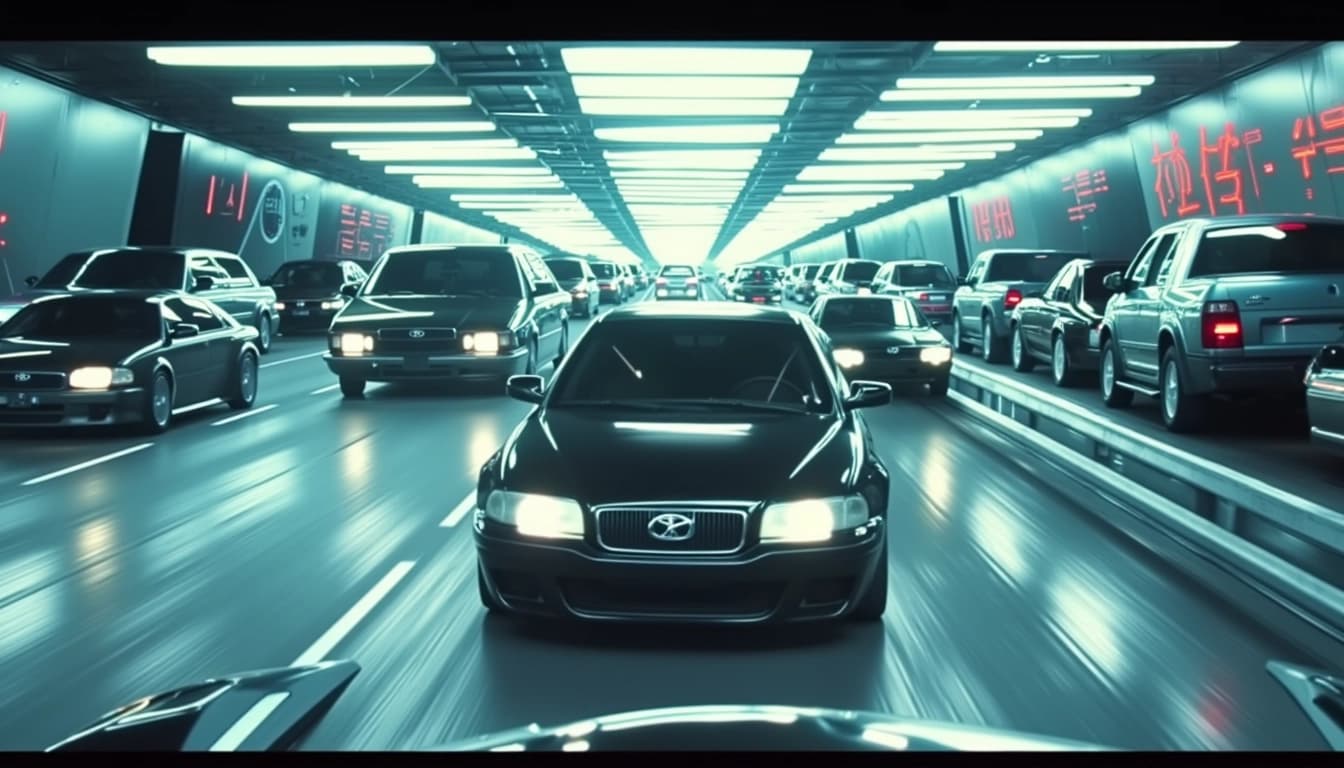
- High-precision object tracking
- Improved segmentation accuracy
- Easy API integration via Replicate
- Positive community feedback