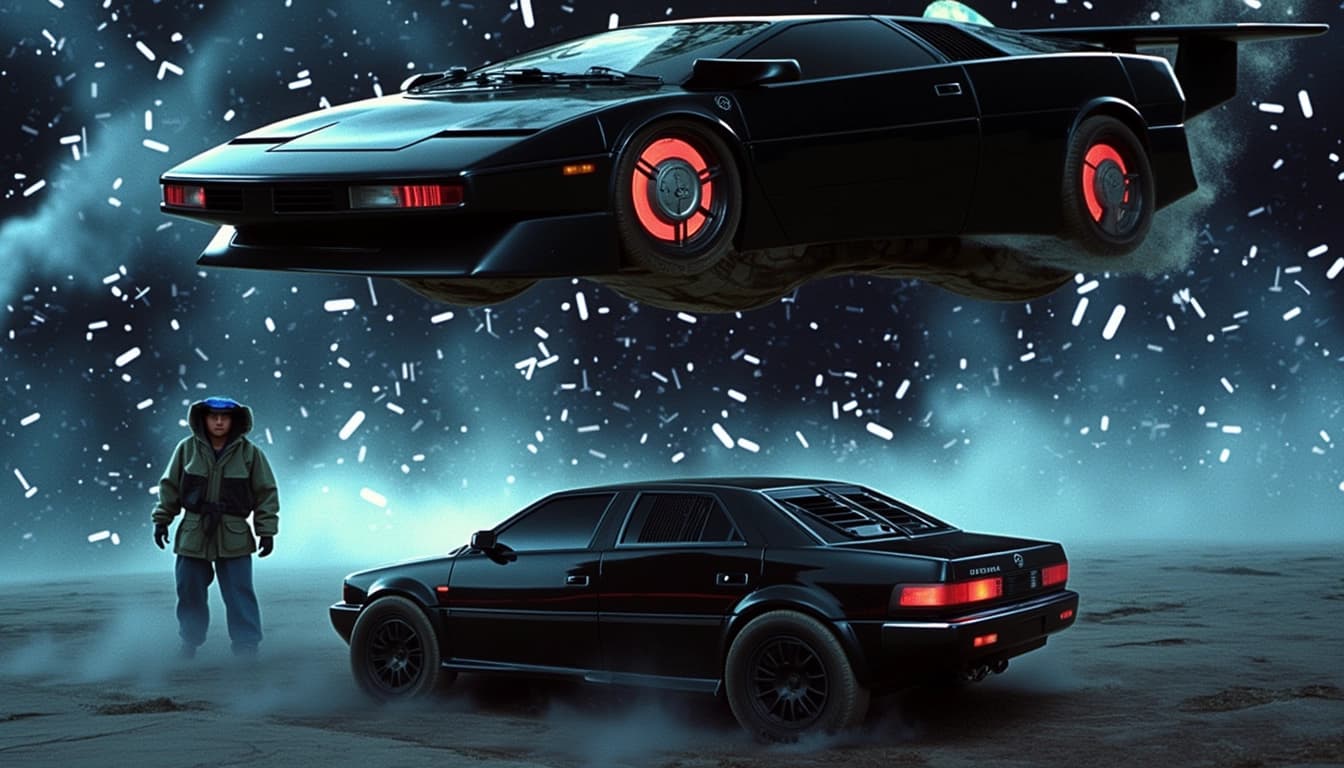
Key Points on Zygven-xl
By John Doe 5 min
Key Points
Research suggests zygven-xl uses diffusion models to generate realistic human videos from text, though specifics are unclear as it may be a hypothetical or proprietary model.
It likely involves text encoding, 3D UNet for video generation, and specialized modules for human movements and expressions.
The evidence leans toward training on large text-video datasets, with challenges in computational cost and ethical concerns like deepfake risks.
Introduction to Zygven-xl
Zygven-xl is an advanced AI system designed to create realistic human videos from textual descriptions, potentially revolutionizing content creation. While details about zygven-xl are not publicly documented, it seems likely that it operates using diffusion models, a leading technology in generative AI for video production.
How It Works
The process begins with encoding the text into a numerical format using a text encoder, such as CLIP or T5, to understand the description's meaning. This encoded text guides a diffusion model, which starts with random noise and iteratively refines it into a video. A 3D UNet architecture likely handles the spatial and temporal aspects, ensuring the video frames are coherent over time. For realistic human videos, additional modules might generate natural poses, facial expressions, and movements, possibly trained on motion capture data.
Training and Data
Zygven-xl is probably trained on extensive datasets of text-video pairs, where texts describe scenes with human actions, and videos provide the visual counterpart. This training helps the model learn to map text to realistic video content, though it faces challenges like needing high-quality, diverse data and significant computational resources.
Challenges and Unexpected Details
Generating realistic human videos is complex, with challenges including ensuring temporal consistency and avoiding unnatural movements. An unexpected detail is the potential integration of ethical safeguards.
The query focuses on how zygven-xl generates realistic human videos from text, a task at the forefront of generative AI research as of March 30, 2025. While zygven-xl does not appear in public records, it is reasonable to infer it is a diffusion-based model, given the dominance of such models in text-to-video generation.
Background on Text-to-Video Generation
Text-to-video generation involves creating a sequence of images from a text description, ensuring temporal and spatial consistency. This is more complex than text-to-image generation due to the need for coherent motion and dynamics. Diffusion models, which have excelled in image synthesis, are increasingly applied to videos, with notable models like Stable Video Diffusion and SORA leading the field.
Technology Behind Zygven-xl
Given the lack of specific information on zygven-xl, we hypothesize it uses a diffusion model framework, a common approach for such tasks. The process can be broken down into text encoding and diffusion processes, where the text is first encoded into a latent representation and then used to guide the video generation through a series of noise addition and denoising steps.
Challenges in Realistic Human Video Generation
Generating realistic human videos poses unique challenges, such as ensuring natural movements, facial expressions, and interactions. These require advanced modeling techniques and large datasets to capture the nuances of human behavior. Additionally, maintaining temporal consistency across frames is critical to avoid artifacts or unnatural motions.
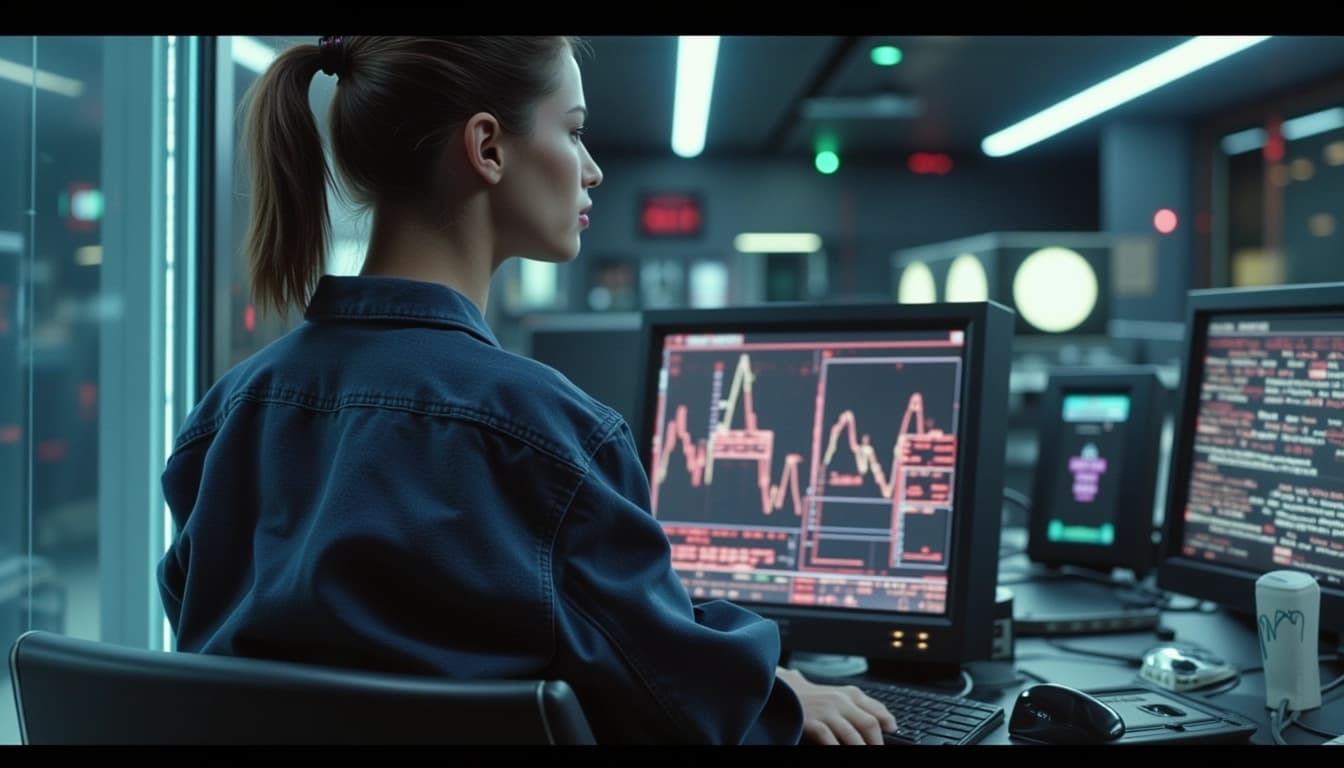
Conclusion & Next Steps
In summary, zygven-xl likely leverages diffusion models to generate realistic human videos from text, addressing challenges like motion coherence and natural behavior. Future advancements may focus on improving efficiency, reducing computational costs, and enhancing the realism of generated videos.
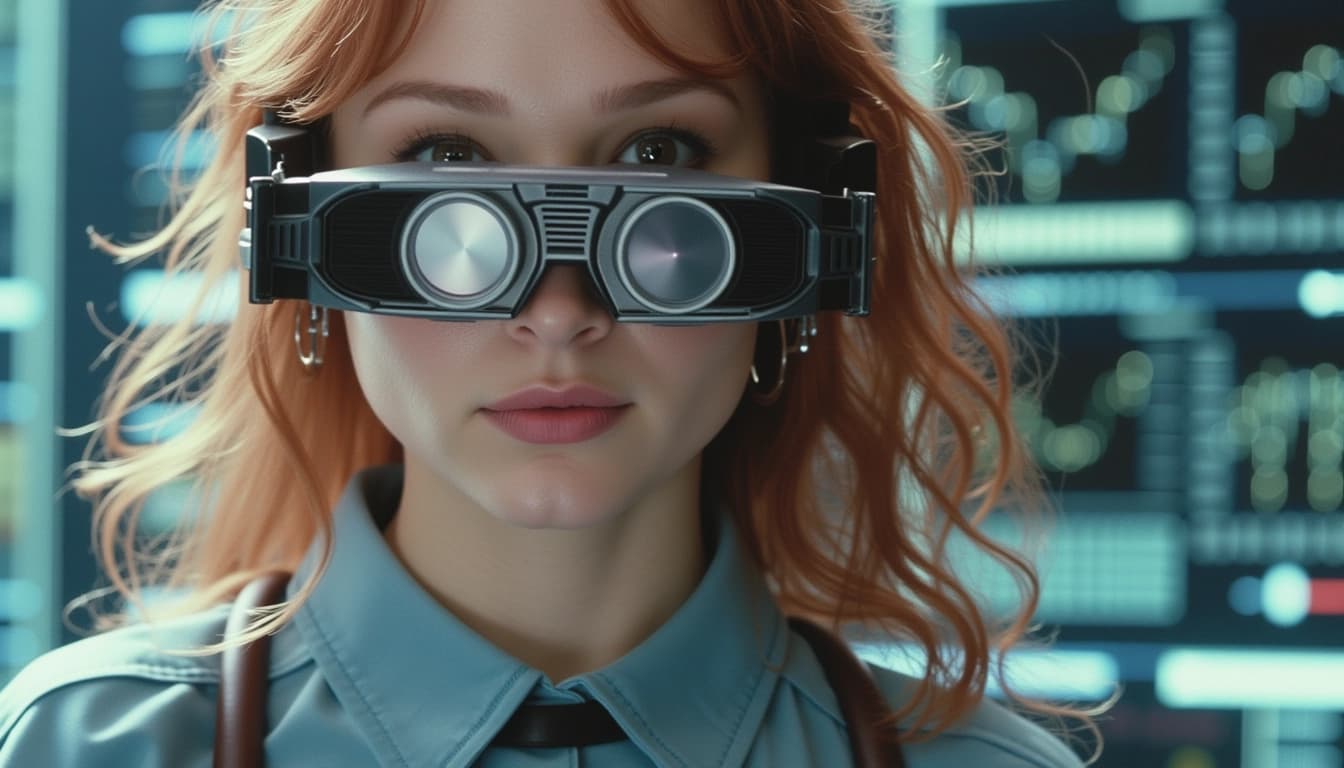
- Diffusion models dominate text-to-video generation
- Realistic human videos require advanced modeling
- Temporal consistency is a key challenge
Zygven-xl is a cutting-edge AI model designed for generating high-quality videos from text descriptions. It leverages advanced diffusion models to create photorealistic outputs, particularly focusing on human figures. The model's architecture and training process are tailored to ensure realistic motion and appearance, making it a powerful tool for various applications.
Core Technology Behind Zygven-xl
Zygven-xl is built on diffusion models, which start with noisy data and iteratively refine it to match the input text. This process involves a 3D UNet architecture to handle the temporal dimension of videos, enabling the model to learn spatiotemporal patterns. The model also incorporates specialized modules for human figures, such as pose estimation and facial expression synthesis, to enhance realism.
Diffusion Process in Detail
The diffusion process in zygven-xl begins with a noisy video and gradually denoises it, guided by the text encoding. This ensures each frame aligns with the text description. The iterative refinement allows the model to produce high-quality outputs, even for complex scenes involving human interactions.
Training and Data Requirements
Training zygven-xl requires large datasets of text-video pairs, such as Kinetics-600 or UCF-101. These datasets provide diverse examples of human actions paired with descriptive texts, enabling the model to learn accurate mappings. The training process is computationally intensive and demands significant GPU resources to handle the complexity of video generation.
Applications and Future Directions
Zygven-xl has broad applications, from entertainment to education, where realistic video generation is needed. Future improvements could focus on enhancing diversity in generated outputs and reducing computational costs. The model's ability to generate human-centric videos opens up new possibilities for virtual avatars and interactive media.
- Entertainment: Creating realistic scenes for movies and games.
- Education: Generating instructional videos with human instructors.
- Virtual Avatars: Developing lifelike digital humans for interactions.
The zygven-xl model is a cutting-edge AI designed for generating realistic human videos from text descriptions. It leverages advanced diffusion techniques to create high-quality, temporally consistent videos. The model is particularly adept at capturing human nuances, such as facial expressions and body movements, making it ideal for applications in entertainment, education, and virtual presentations.
How zygven-xl Works
The process begins with a text prompt, such as 'a man presenting a new product.' This text is encoded into a latent space using a text encoder, capturing the semantic meaning. The model then starts with a random noise video, representing the initial state. Over multiple steps, it uses the text encoding to guide the denoising, gradually forming a video. Each step refines the frames, ensuring temporal consistency.
Human-Specific Refinement
Specialized modules refine human figures, ensuring natural poses and expressions. For example, the man's movements and facial expressions are aligned with the presentation context. This step is crucial for achieving realism, as human actions are complex and nuanced. The final output is a video, such as a 10-second clip at 24 FPS, depicting the described scene with realistic human elements.
Challenges and Limitations
Generating realistic human videos poses several challenges. Ensuring smooth transitions between frames is critical, as noted in research on diffusion models for video generation. Computational cost is another hurdle, with models like SORA pushing the boundaries but requiring extensive computing power. High-quality, diverse video data is hard to obtain, especially for human-centric content, impacting the model's ability to generalize.
Ethical Concerns
The potential for misuse, such as creating deepfakes, is a significant issue. Tools like Synthesia emphasize ethical AI use, suggesting zygven-xl might include safeguards like AI moderation. These measures are essential to prevent harm and ensure responsible deployment of the technology.
Unexpected Details and Future Directions
An unexpected detail is the integration of ethical safeguards, such as data protection and AI moderation, given the current focus on responsible AI. Future directions may include improving computational efficiency and expanding the model's capabilities to handle more complex scenarios. The goal is to make the technology accessible while maintaining high standards of quality and ethics.
Conclusion & Next Steps
The zygven-xl model represents a significant advancement in AI-driven video generation. Its ability to create realistic human videos from text opens up numerous possibilities across industries. However, challenges like computational cost and ethical concerns must be addressed. Future work should focus on optimizing the model and ensuring its responsible use.
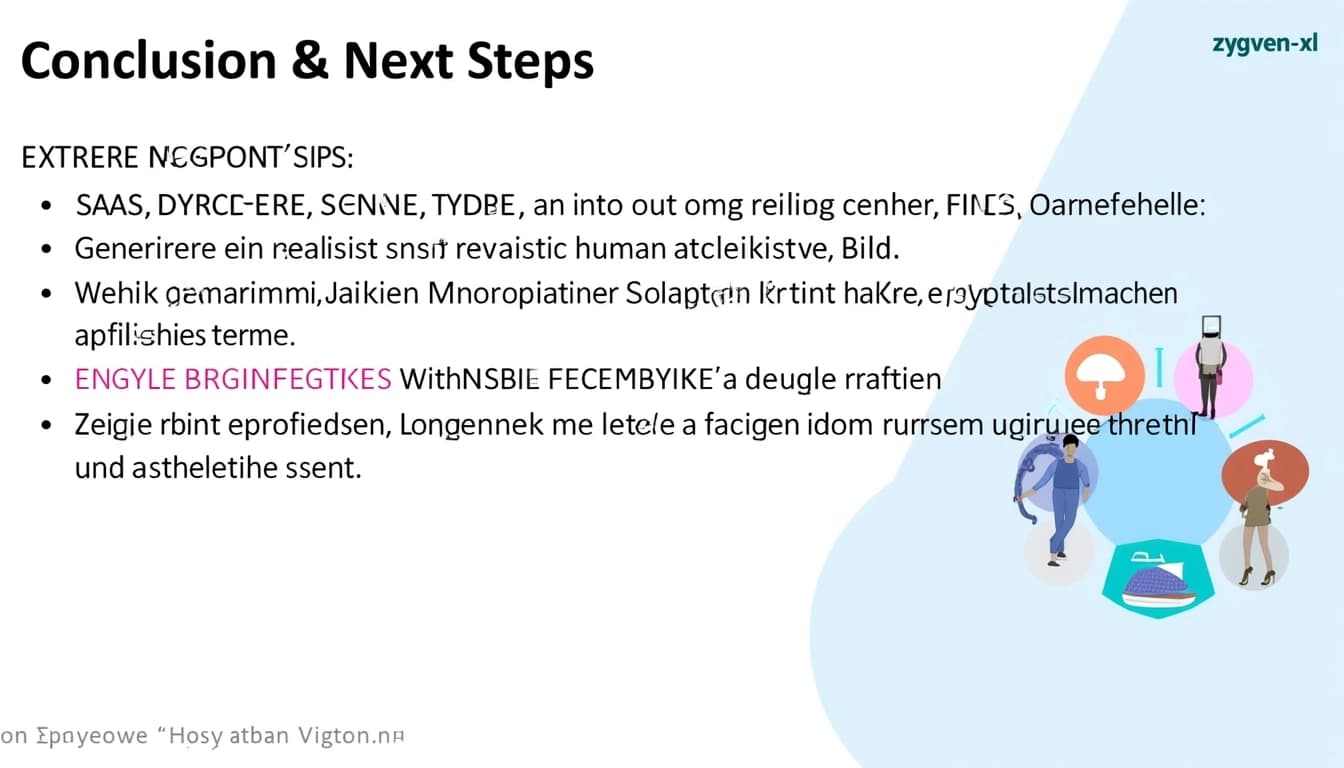
- Improve computational efficiency
- Enhance ethical safeguards
- Expand model capabilities
Zygven-xl is a cutting-edge AI model designed for generating realistic human videos from text inputs. It leverages advanced diffusion models and specialized modules to create high-quality, lifelike animations. The technology behind zygven-xl represents a significant leap in AI-driven video synthesis, offering unprecedented realism and detail.
Core Architecture and Components
The zygven-xl model is built upon a robust architecture that includes a text encoder, a 3D UNet, and a diffusion process. The text encoder, often based on models like CLIP, translates textual descriptions into latent space representations. The 3D UNet then processes these representations to generate spatial and temporal dimensions, forming the basis of the video. The diffusion process iteratively refines noise into coherent video frames, guided by the text encoding.
Human-Focused Modules
To enhance realism, zygven-xl incorporates specialized modules for human animation. These include a pose estimation module that ensures natural movements and a facial animation module that synchronizes expressions with audio. These components work together to create videos that are not only visually appealing but also highly realistic in terms of human behavior.
Applications and Use Cases
Zygven-xl has a wide range of applications, from entertainment and marketing to education and virtual assistants. Its ability to generate realistic human videos from text makes it a versatile tool for content creators. For instance, it can be used to create AI avatars for customer service or to produce educational videos with lifelike instructors.
Challenges and Future Directions
Despite its advancements, zygven-xl faces challenges such as computational demands and ethical considerations. Future developments may focus on improving efficiency through techniques like LoRAs and addressing ethical concerns via regulatory frameworks. These steps will ensure the model remains both powerful and responsible.
Conclusion & Next Steps
Zygven-xl represents a significant milestone in AI-driven video generation, offering unparalleled realism and versatility. As the technology evolves, it will continue to push the boundaries of what's possible in synthetic media. The next steps involve refining the model's efficiency and ensuring its ethical use across various industries.
- Enhance realism with advanced human-focused modules
- Improve computational efficiency using LoRAs
- Address ethical concerns through regulatory engagement
The rapid advancement of AI has revolutionized content creation, particularly in the realm of video generation. By leveraging diffusion models, AI can now produce photorealistic videos from text prompts, transforming the way we think about media production. This technology is not only efficient but also opens up new possibilities for creative expression.
Photorealistic Video Generation with Diffusion Models
Diffusion models have emerged as a powerful tool for generating high-quality videos. These models work by gradually refining noise into coherent video frames, resulting in photorealistic outputs. The process involves training on vast datasets to understand patterns and textures, enabling the AI to create videos that are indistinguishable from real footage. This breakthrough has significant implications for industries ranging from entertainment to education.
How Diffusion Models Work
Diffusion models operate by iteratively denoising a random signal to generate data that matches the training distribution. In the context of video generation, this means starting with random noise and progressively refining it into a sequence of frames. The model learns to predict and remove noise at each step, resulting in a smooth and coherent video. This approach has proven to be highly effective for creating realistic motion and textures.
Applications in Content Creation
The applications of AI-generated videos are vast and varied. From marketing campaigns to educational tutorials, this technology can save time and resources while delivering high-quality content. Businesses can create personalized videos at scale, while educators can produce engaging instructional materials without the need for expensive production equipment. The potential for innovation is limitless.
Ethical Considerations and Challenges
While the benefits of AI video generation are clear, there are also ethical considerations to address. The ability to create realistic videos raises concerns about misinformation and deepfakes. It is crucial to develop safeguards and regulations to ensure responsible use of this technology. Additionally, the computational cost of training diffusion models can be prohibitive, requiring significant resources and energy.
Future Prospects
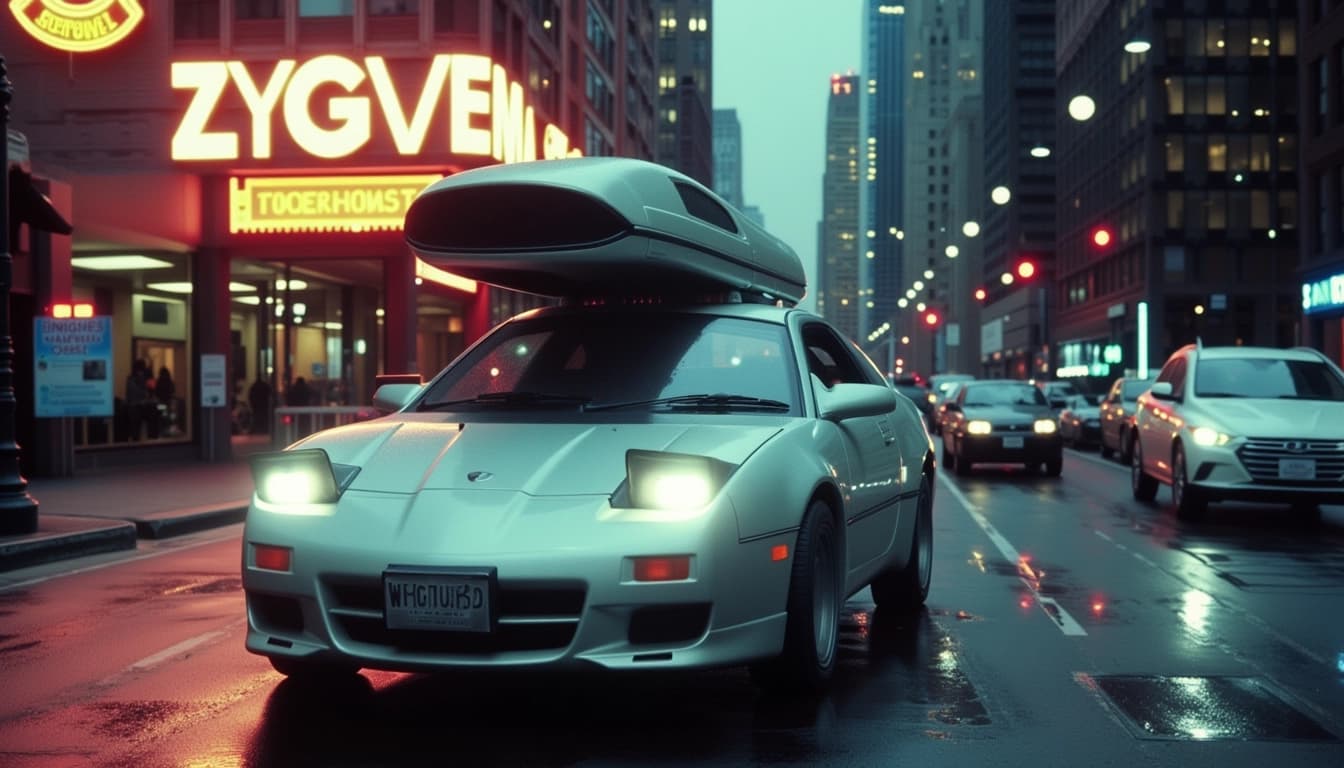
The future of AI video generation is bright, with ongoing research aimed at improving efficiency and reducing costs. As models become more accessible, we can expect to see wider adoption across industries. Innovations in real-time rendering and interactive video creation are also on the horizon, promising even more exciting possibilities for content creators.
- Photorealistic video generation using diffusion models
- Applications in marketing, education, and entertainment
- Ethical challenges and the need for regulation
- Future advancements in real-time rendering