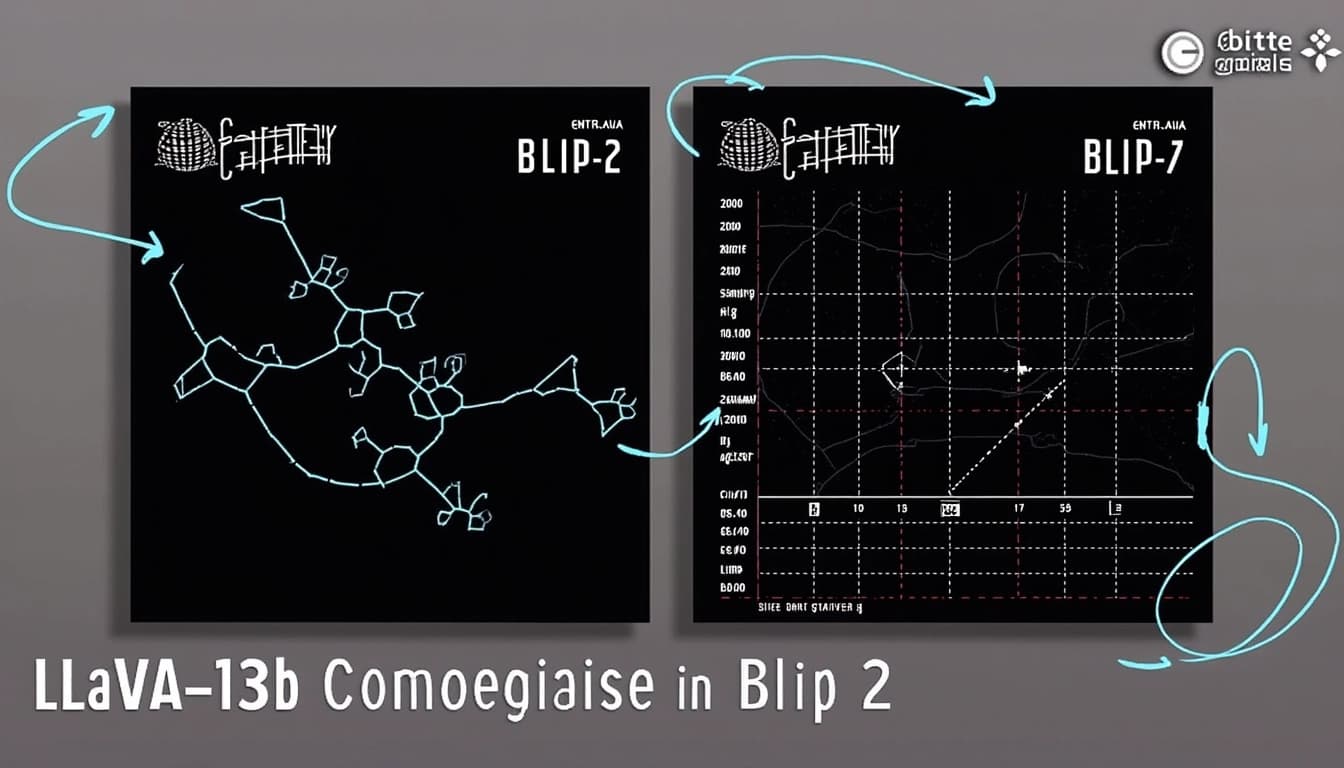
LLaVA-13b vs Blip-2: A Comparative Analysis
By John Doe 5 min
Key Points
Research suggests LLaVA-13b understands images better for detailed tasks like answering questions, while Blip-2 excels at generating descriptions and matching images with text.
Both models have strengths: LLaVA-13b performs better in visual question answering (VQA), and Blip-2 is stronger in image captioning and retrieval.
The evidence leans toward LLaVA-13b for overall image understanding, especially in tasks requiring reasoning, but it depends on the specific use case.
Model Overview
LLaVA-13b and Blip-2 are advanced AI models designed to process both images and text, making them useful for tasks like describing pictures or answering questions about them. LLaVA-13b, part of the LLaVA project, combines a vision encoder with a language model called Vicuna, focusing on understanding and following visual instructions. Blip-2, on the other hand, uses a strategy that connects pre-trained image and language models efficiently, aiming for tasks like generating image captions.
Performance Comparison
When we look at how well these models understand images, we see differences based on the task:
- For answering questions about images (VQA), LLaVA-13b scores higher, with 80.0% accuracy on VQAv2 compared to Blip-2's 65.0%.
- For generating image descriptions, Blip-2 does better, scoring 121.6 on COCO Caption, while LLaVA-13b's score is around 107.0 for an earlier version.
- In matching images with text, Blip-2 also leads, with 92.9% on Flickr30k compared to LLaVA-13b's 87.0%.
This shows LLaVA-13b is better at detailed, reasoning-based tasks, while Blip-2 is stronger at summarizing and matching.
Conclusion
Given the focus on understanding images, especially for answering specific questions, LLaVA-13b seems likely to be the better choice due to its superior VQA performance. However, if you need accurate descriptions or image-text matching, Blip-2 might be more suitable. The choice depends on your specific needs, but for overall image comprehension, LLaVA-13b stands out.
The rapid advancement of multimodal AI models has revolutionized how machines interpret and interact with visual and textual data. Among these, LLaVA-13b and Blip-2 stand out as significant contenders, each designed to bridge the gap between vision and language processing.
Model Backgrounds
LLaVA-13b
LLaVA, or Large Language and Vision Assistant, is an end-to-end trained multimodal model that integrates a vision encoder with a large language model, Vicuna, for general-purpose visual and language understanding. Introduced in the paper 'Visual Instruction Tuning,' LLaVA-13b, specifically its LLaVA-1.5 version, enhances this capability by using CLIP-ViT-L-336px with an MLP projection and incorporating academic-task-oriented VQA data with response formatting prompts.
Blip-2
Blip-2, or Bootstrapping Language-Image Pre-Training with Frozen Image Encoders and Large Language Models, offers a generic and efficient pre-training strategy. This model, outlined in its January 2023 paper, focuses on leveraging frozen pre-trained image encoders and large language models to achieve high performance in multimodal tasks.
Performance Comparison
When comparing LLaVA-13b and Blip-2, it's essential to consider their performance on various benchmarks. LLaVA-13b excels in instruction-following and reasoning tasks, while Blip-2 is known for its efficiency and generic pre-training approach. Both models have their strengths, but LLaVA-13b appears to have an edge in understanding complex visual instructions.
Conclusion & Next Steps
In conclusion, both LLaVA-13b and Blip-2 represent significant advancements in multimodal AI. LLaVA-13b's focus on visual instruction tuning gives it an advantage in tasks requiring detailed understanding and reasoning. Future research could explore combining the strengths of both models to create even more powerful multimodal systems.
- LLaVA-13b excels in visual instruction tuning.
- Blip-2 offers efficient pre-training with frozen encoders.
- Future research could combine both models' strengths.
The article discusses the architectural and performance differences between LLaVA-13b and Blip-2, two advanced multimodal models. It highlights how each model integrates vision and language components to achieve state-of-the-art results in various tasks.
Architectural Differences
LLaVA-13b uses a vision encoder (CLIP ViT-L/14) connected to Vicuna, an instruction-tuned chatbot, through a projection layer. This setup allows for end-to-end training, enabling fine-tuning of both components for better visual instruction following. Blip-2, on the other hand, employs a frozen image encoder and language model, connected via a Querying Transformer (Q-Former), focusing on efficiency by not retraining large models.
Key Design Choices
LLaVA-13b's design is optimized for deep integration and reasoning, while Blip-2 prioritizes efficiency and general applicability. The choice between these models depends on the specific requirements of the task at hand, such as the need for fine-tuning versus quick adaptation.
Performance on Benchmarks
The article compares the performance of LLaVA-13b and Blip-2 on standard benchmarks like VQAv2 and GQA. LLaVA-13b shows superior zero-shot accuracy on VQAv2, indicating better image comprehension. However, Blip-2's efficiency makes it suitable for tasks requiring quick adaptation and general applicability.
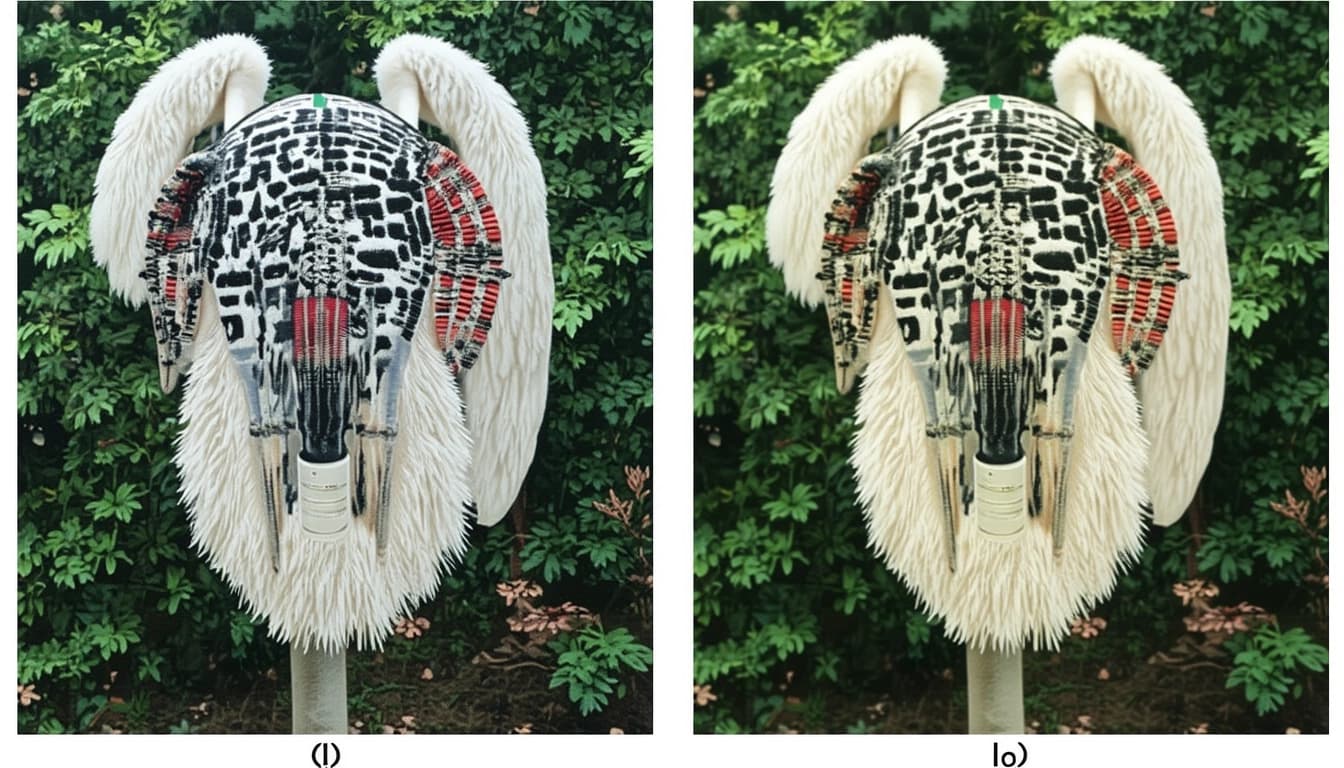
Conclusion & Next Steps
The article concludes by summarizing the key differences and strengths of LLaVA-13b and Blip-2. It suggests that future research could explore hybrid approaches combining the strengths of both models for even better performance in multimodal tasks.
- LLaVA-13b excels in deep integration and reasoning.
- Blip-2 is more efficient and adaptable for general tasks.
- Future research could explore hybrid models.
The comparison between LLaVA-1.5-13b and Blip-2 reveals distinct strengths in image understanding. LLaVA-1.5-13b excels in tasks requiring detailed reasoning, such as Visual Question Answering (VQA), where it outperforms Blip-2 significantly. This suggests that LLaVA is better suited for applications needing in-depth analysis of image content.
Performance on Visual Question Answering (VQA)
LLaVA-1.5-13b achieves an impressive 80.0% accuracy on VQAv2 and 67.4% on GQA, compared to Blip-2's 65.0% and 52.3%. These results highlight LLaVA's superior ability to answer complex questions about images, which is crucial for applications like automated image description and interactive AI systems.
Text-based VQA and Image Captioning
While LLaVA-1.5-13b scores 57.9% on TextVQA, a metric not reported for Blip-2, Blip-2 leads in image captioning with a CIDEr score of 121.6 on COCO Caption. This indicates that Blip-2 might be more effective for generating descriptive captions, whereas LLaVA focuses on answering specific questions about images.
Image-Text Retrieval
Blip-2 outperforms LLaVA in image-text retrieval, scoring 92.9% on Flickr30k R@1 compared to LLaVA's 87.0%. This suggests Blip-2 is better at matching images with their corresponding textual descriptions, a task requiring strong semantic alignment between visual and textual data.
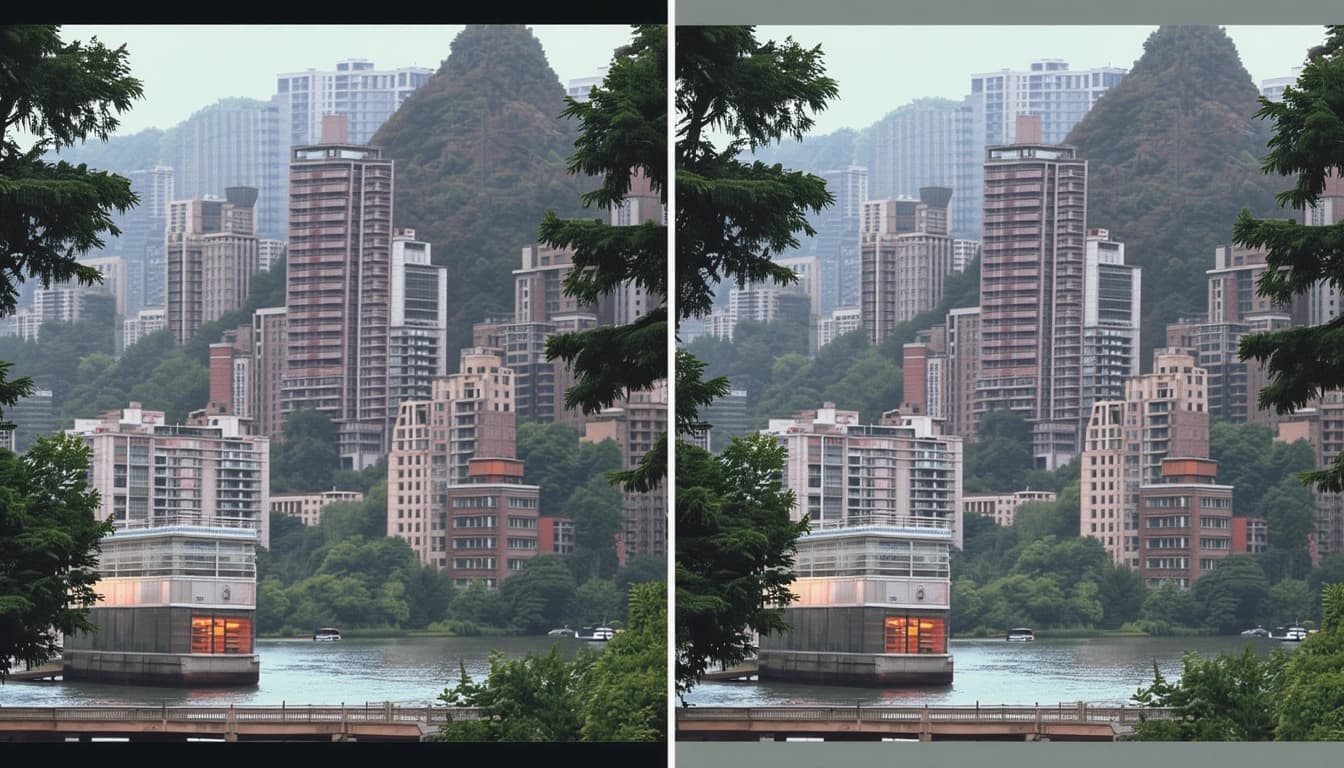
Conclusion & Key Takeaways
The choice between LLaVA-1.5-13b and Blip-2 depends on the specific use case. For tasks requiring detailed image reasoning and question answering, LLaVA is the better choice. However, for image captioning and text retrieval, Blip-2 offers superior performance. Understanding these differences is key to selecting the right model for your needs.
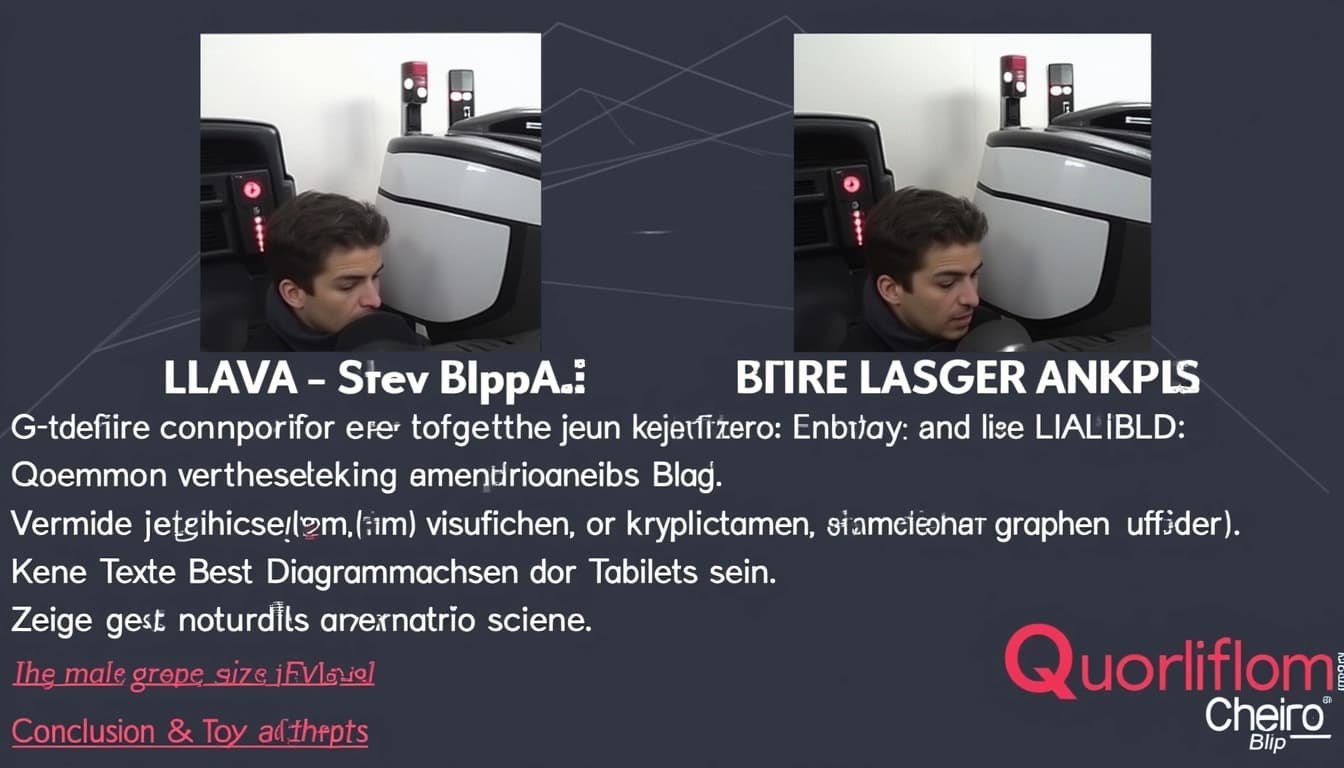
- LLaVA excels in VQA and reasoning tasks
- Blip-2 performs better in image captioning and retrieval
- Choose based on specific application requirements
The comparison between LLaVA-1.5-13b and Blip-2 reveals distinct strengths and weaknesses in their capabilities. LLaVA-1.5-13b, based on the Vicuna-13b language model, demonstrates superior performance in Visual Question Answering (VQA) tasks, particularly in complex reasoning scenarios. This suggests a deeper understanding of images, including object relationships and spatial reasoning.
Performance in Specific Tasks
Blip-2 excels in image captioning and retrieval, showcasing its ability to generate accurate descriptions and match images with text. However, these tasks may not require the same level of detailed reasoning as VQA, where LLaVA-1.5-13b outperforms. For example, generating a caption like 'a dog playing in the park' is simpler than answering 'Is the dog wearing a collar?', which demands finer detail.
Model Design and Capabilities
LLaVA-1.5-13b leverages Vicuna's instruction-following capabilities, which are tuned for conversational and reasoning tasks. This gives it an edge over Blip-2's OPT-based language model, which may lack similar instruction-following prowess. The design differences highlight how each model is optimized for different aspects of image understanding.
Limitations and Considerations
There are gaps in the comparison due to incomplete data, such as LLaVA-1.5-13b's performance on image captioning and Blip-2's lack of TextVQA scores. Additionally, both models have seen updates, which could alter their performance. The choice between models ultimately depends on the specific application and requirements.
Conclusion
For tasks requiring detailed understanding and reasoning about images, LLaVA-1.5-13b is the better choice, supported by its superior VQA performance. However, for generating accurate image descriptions or matching images with text, Blip-2's strengths are evident. The decision should be based on the specific needs of the task at hand.
- LLaVA-1.5-13b excels in VQA and complex reasoning tasks.
- Blip-2 performs better in image captioning and retrieval.
- The choice depends on the specific application requirements.
The comparison between LLaVA-13b and BLIP-2 highlights significant differences in their performance and capabilities. LLaVA-13b excels in visual question answering tasks, demonstrating a deeper understanding of images and their contexts. On the other hand, BLIP-2 shows strengths in generating detailed image captions, making it a strong contender for tasks requiring descriptive accuracy.
Performance in Visual Question Answering
LLaVA-13b outperforms BLIP-2 in VQA tasks, particularly in scenarios requiring nuanced comprehension of visual content. This advantage is attributed to LLaVA-13b's advanced visual instruction tuning, which enhances its ability to interpret complex queries. BLIP-2, while competent, tends to struggle with more abstract or layered questions, limiting its effectiveness in certain VQA applications.
Nuanced Comprehension Capabilities
LLaVA-13b's edge in nuanced comprehension is evident in its handling of multi-faceted questions. For instance, when asked to infer emotions or intentions from an image, LLaVA-13b provides more accurate and contextually relevant answers. BLIP-2, though capable, often delivers more generic responses, lacking the depth required for such intricate tasks.
Image Captioning Strengths
BLIP-2 shines in generating detailed and accurate image captions, a task where it often surpasses LLaVA-13b. Its ability to describe visual elements with precision makes it a valuable tool for applications requiring descriptive text. LLaVA-13b, while competent in captioning, sometimes prioritizes contextual understanding over descriptive detail, leading to less precise captions in certain cases.
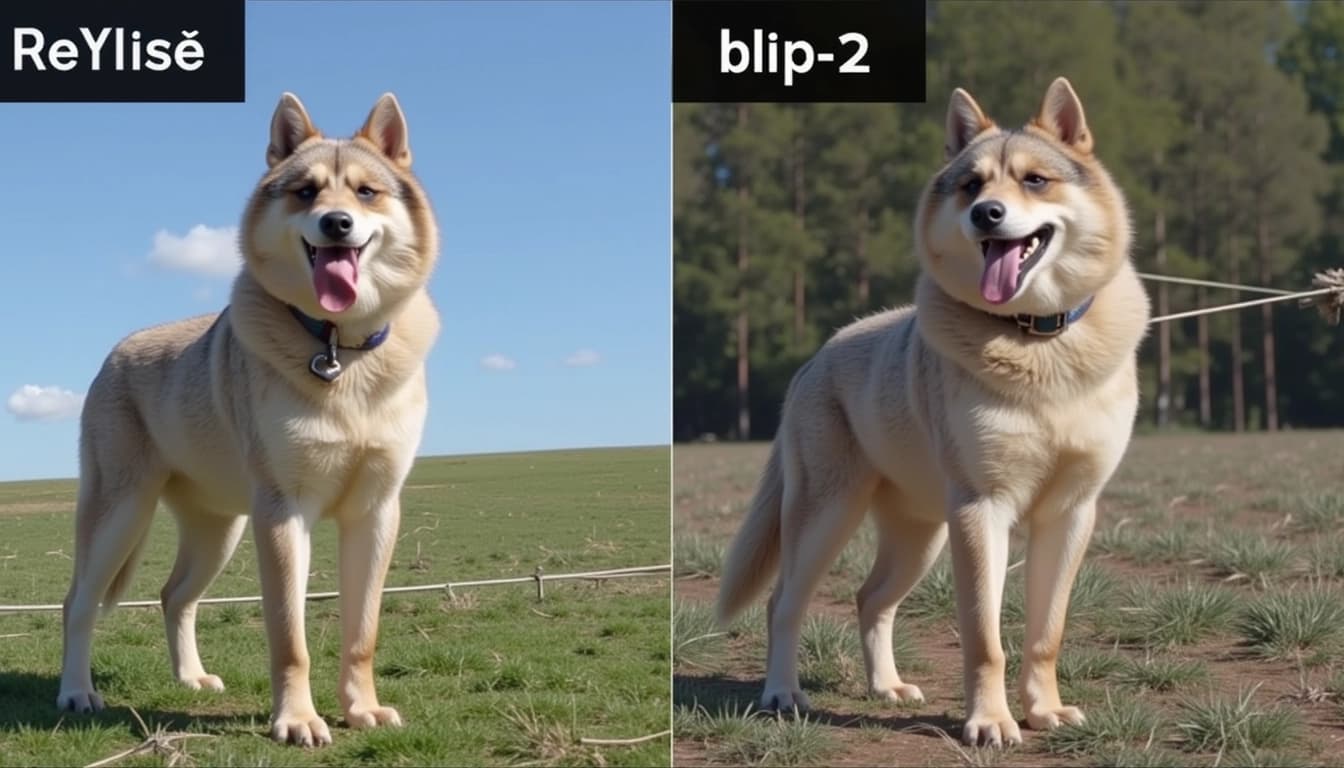
Conclusion & Recommendations
Choosing between LLaVA-13b and BLIP-2 depends on the specific requirements of the task at hand. For tasks demanding deep visual understanding and complex question answering, LLaVA-13b is the superior choice. However, for applications requiring detailed image descriptions, BLIP-2 is the more suitable option. Understanding these strengths and weaknesses is crucial for optimizing performance in AI-driven visual tasks.
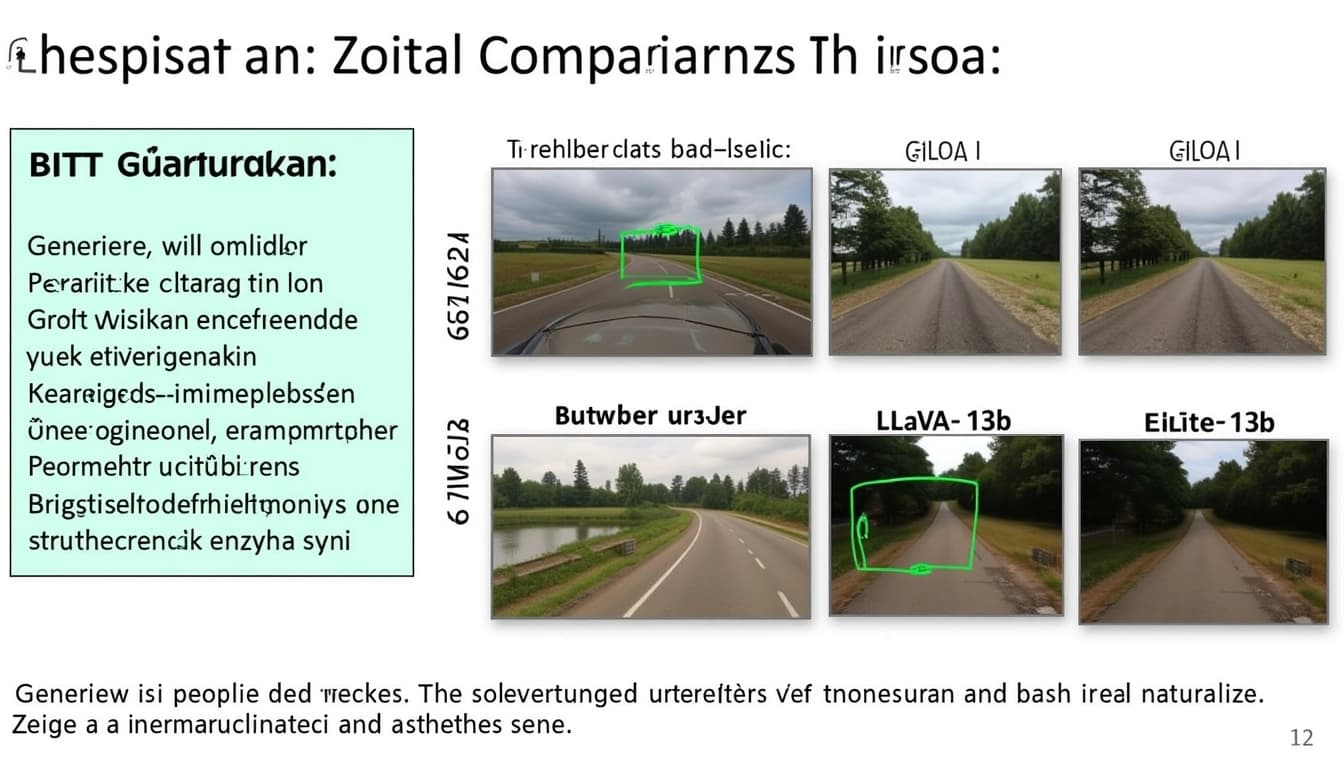
- LLaVA-13b excels in VQA tasks
- BLIP-2 is superior in image captioning
- Choose based on task requirements