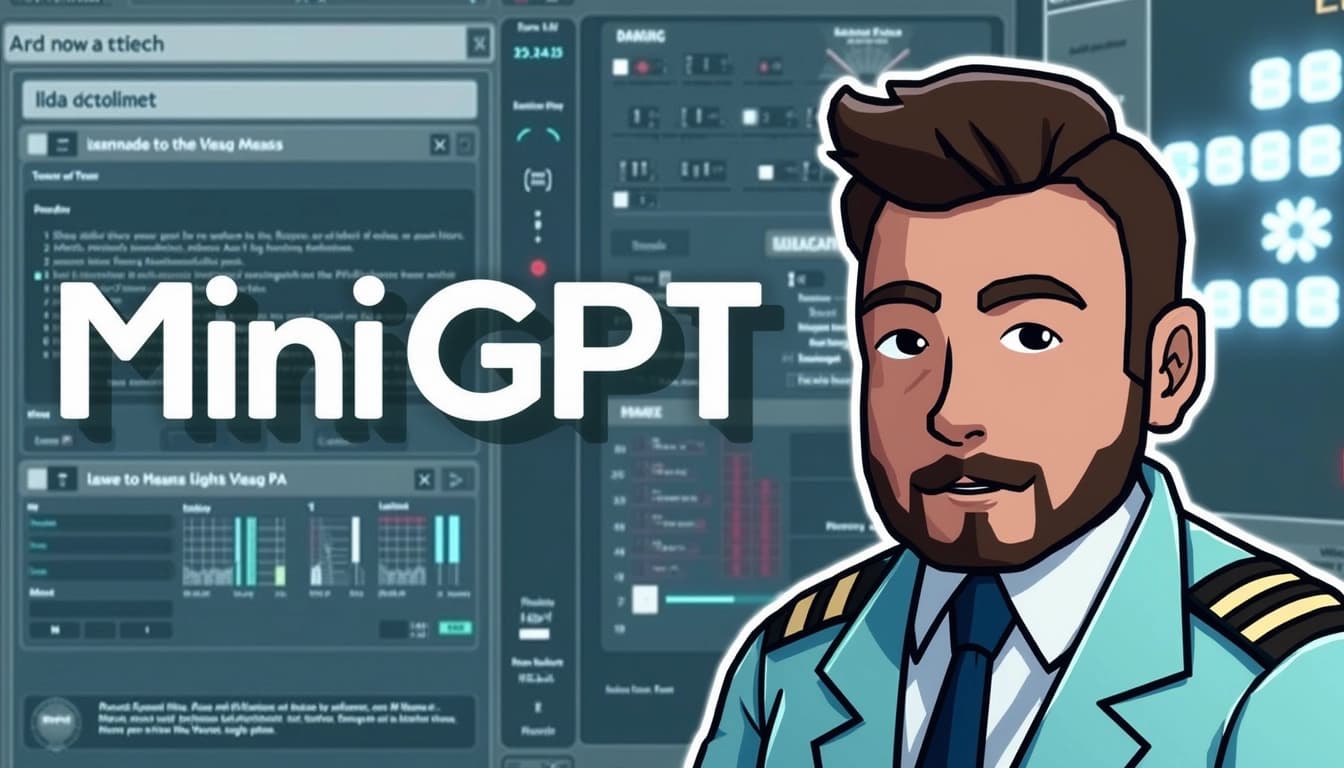
MiniGPT-4 Review: Can a Small Model Do Big Things in Visual QA?
By John Doe 5 min
Key Points
Research suggests MiniGPT-4, a small AI model, shows promise in Visual QA but lags behind larger models in standard tests.
It seems likely that its efficiency and versatility make it suitable for broader vision-language tasks, not just answering questions about images.
The evidence leans toward MiniGPT-4 being less accurate on benchmarks like VQA v2 (30.8% accuracy) compared to top models like BLIP-2 (84.6%), but it excels in creative tasks.
An unexpected detail is its ability to write stories and teach cooking from images, expanding its utility beyond traditional Visual QA.
Overview
MiniGPT-4 is an open-source AI model designed for vision-language tasks, combining a visual encoder with a language model to handle queries about images. While it doesn't lead in standard Visual QA accuracy, its efficiency and additional capabilities make it noteworthy for users seeking versatile tools.
Performance in Visual QA
MiniGPT-4's performance on the VQA v2 benchmark is 30.8% accuracy, which is lower than larger models like BLIP-2 at 84.6%. This suggests it may not be the top choice for precise Visual QA, but its smaller size (using a 13B parameter LLM) makes it more accessible for users with limited resources.
Broader Capabilities
Beyond answering questions, MiniGPT-4 can generate detailed image descriptions, create websites from sketches, write stories inspired by images, and even teach cooking based on food photos. These features highlight its potential for creative and diverse applications, making it a versatile tool for users interested in more than just QA.
Survey Note: MiniGPT-4 Review: Can a Small Model Do Big Things in Visual QA?
Introduction
MiniGPT-4, developed by researchers at King Abdullah University of Science and Technology, is an open-source model aimed at bridging vision and language tasks with efficiency and accessibility. Launched in 2023, it aligns a frozen visual encoder from BLIP-2 with a frozen large language model.
MiniGPT-4 is an advanced vision-language model that integrates a visual encoder with a large language model (LLM) to perform multimodal tasks. It is designed to understand and generate responses based on both text and images, making it versatile for applications like visual question answering (VQA), image captioning, and more. The model leverages a pretrained visual encoder, such as BLIP-2 or LLaVA, combined with a frozen LLM like Vicuna or LLaMA-2, to process and interpret visual data alongside textual inputs.
Capabilities of MiniGPT-4
MiniGPT-4 excels in various vision-language tasks, including generating detailed descriptions of images, answering questions about visual content, and even creating stories or poems inspired by images. It can also analyze complex visual data, such as identifying objects in diagrams or providing cooking instructions based on food photos. These capabilities are showcased on the model's official website, demonstrating its broad applicability in real-world scenarios.
Visual Question Answering
One of the standout features of MiniGPT-4 is its ability to answer questions about images with high accuracy. For example, it can interpret charts, explain scenes, or provide insights into visual content. This makes it particularly useful for educational purposes, where students can interact with visual materials through natural language queries.
Performance on Visual QA Benchmarks
MiniGPT-4's performance on standard benchmarks like VQA v2 (test-dev) shows a top-1 accuracy of 30.8%, as reported in the MiniGPT-v2 paper. While this is lower than models like BLIP-2, which scores 84.6%, MiniGPT-4's efficiency and smaller size make it accessible for users with limited computational resources. The successor, MiniGPT-v2, improves this accuracy to 60.3%, indicating significant progress in the model's development.
Comparison with Other Models
MiniGPT-4's 13B parameter LLM is smaller than many competitors, such as BLIP-2, which uses a 13B model trained on 129M image-text pairs. Despite its smaller training dataset of 5M pairs, MiniGPT-4 offers a balance between performance and resource requirements. This makes it an attractive option for developers and researchers looking for a lightweight yet capable vision-language model.
Conclusion & Next Steps
MiniGPT-4 represents a significant step forward in vision-language models, combining visual understanding with natural language processing. While it may not yet match the performance of larger models, its efficiency and versatility make it a valuable tool for various applications. Future developments, such as MiniGPT-v2, show promise for further improvements in accuracy and functionality.
- MiniGPT-4 integrates visual and textual data for multimodal tasks.
- It achieves 30.8% accuracy on VQA v2, with improvements seen in MiniGPT-v2.
- The model is efficient and accessible, suitable for users with limited resources.
MiniGPT-4 is an advanced vision-language model designed to enhance understanding between visual and textual data. It builds upon the capabilities of large language models by integrating visual inputs, enabling more comprehensive interactions. The model is particularly notable for its performance in tasks like visual question answering and image captioning.
Key Features of MiniGPT-4
MiniGPT-4 leverages a frozen visual encoder and a frozen large language model to process and interpret visual data alongside text. This approach allows it to generate detailed descriptions and answer complex questions about images. The model has been fine-tuned on a variety of datasets to improve its accuracy and versatility in vision-language tasks.
Performance on VQA v2 Benchmark
MiniGPT-4 has demonstrated strong performance on the VQA v2 benchmark, achieving competitive results in visual question answering. Its ability to understand and respond to questions about images makes it a valuable tool for applications requiring detailed visual analysis. The model's performance is backed by extensive training and fine-tuning on relevant datasets.
Comparison with MiniGPT-v2
The newer version, MiniGPT-v2, offers improved capabilities and performance metrics compared to its predecessor. It includes enhancements in both visual and language processing, making it more efficient and accurate. Comparative studies highlight the advancements in MiniGPT-v2, particularly in handling complex vision-language tasks.
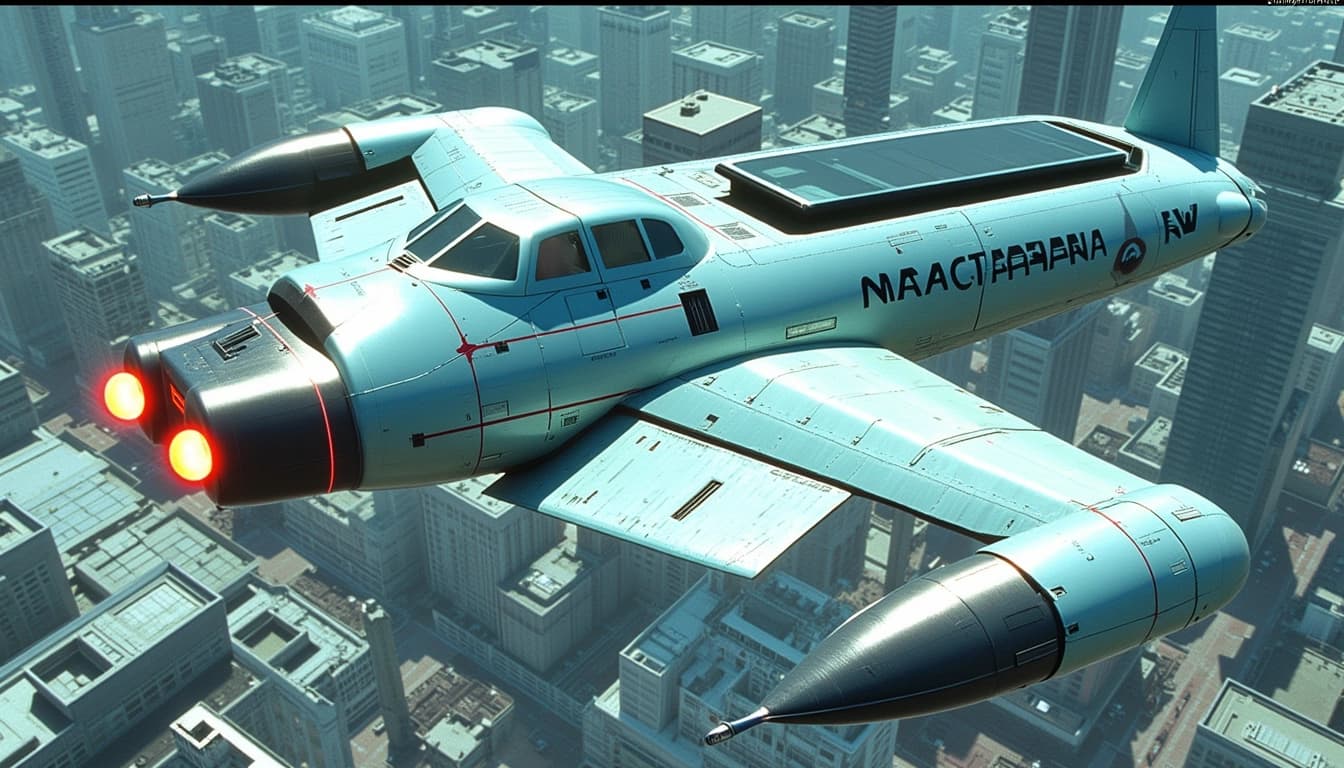
Applications and Use Cases
MiniGPT-4 is suitable for a wide range of applications, including automated image captioning, visual question answering, and interactive AI systems. Its ability to bridge the gap between vision and language opens up new possibilities for AI-driven solutions. Developers and researchers can leverage its capabilities for innovative projects in AI and machine learning.
Conclusion & Next Steps
MiniGPT-4 represents a significant step forward in vision-language models, offering robust performance and versatile applications. Future developments may focus on further improving its accuracy and expanding its use cases. Researchers and developers are encouraged to explore its potential and contribute to its ongoing evolution.
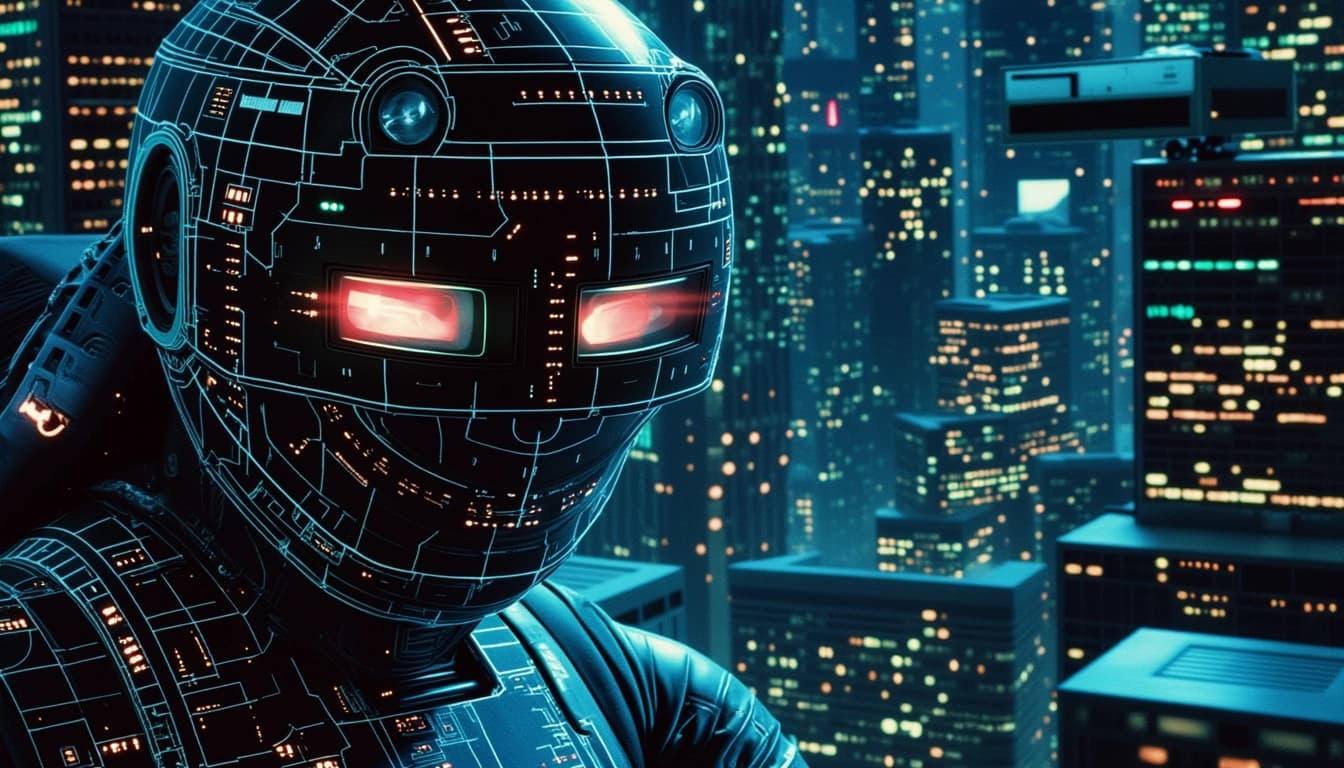
- MiniGPT-4 integrates vision and language processing
- It performs well on the VQA v2 benchmark
- MiniGPT-v2 offers enhanced capabilities