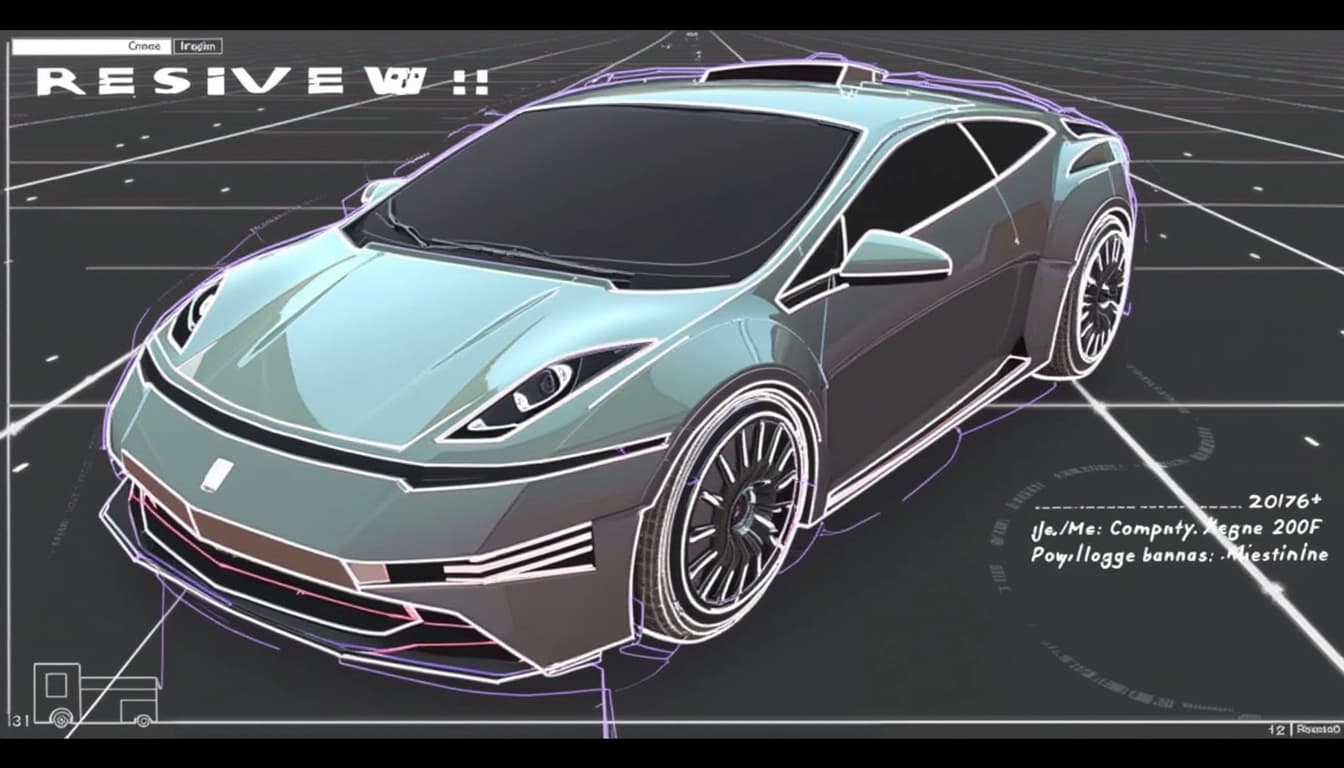
Research on pixart-xl-2 for Fast Visual Prototyping
By John Doe 5 min
Key Points
Research suggests pixart-xl-2 is efficient for fast visual prototyping, generating high-resolution images from text prompts quickly.
It seems likely that its speed, ease of use, and cost-effectiveness make it ideal for rapid design iterations.
The evidence leans toward its competitive performance compared to models like Stable Diffusion and DALL-E 2, with training costs significantly lower.
Introduction to Fast Visual Prototyping
Fast visual prototyping is about quickly creating and refining visual designs to test ideas, gather feedback, and iterate without heavy resource investment. Traditionally, this meant sketching by hand, which could be slow and skill-dependent. Now, AI models like pixart-xl-2 transform text descriptions into images in seconds, revolutionizing the process.
What is pixart-xl-2?
pixart-xl-2, developed by PixArt-alpha, is a text-to-image model using a diffusion-transformer approach. It generates 1024x1024 pixel images from text, trained efficiently in 10.8% of the time needed for Stable Diffusion v1.5, saving nearly $300,000 and reducing CO2 emissions by 90%. It performs comparably to top models like SDXL 0.9 and DALL-E 2, with fast inference times of about 16 seconds on a T4 GPU for the standard version, and just 3.3 seconds for its LCM variant.
Why It’s Ideal for Fast Visual Prototyping
pixart-xl-2 excels in speed, taking seconds to generate images, perfect for iterative design. Its text-prompt system is user-friendly, accessible to non-designers, and cost-effective compared to hiring artists. Users can tweak prompts for variations, and its high-quality output ensures appealing prototypes. An unexpected detail is its environmental benefit, with lower training emissions, aligning with sustainable practices.
Survey Note: Detailed Analysis of pixart-xl-2 for Fast Visual Prototyping
Overview and Context
Fast visual prototyping is a critical phase in design, enabling rapid generation and iteration
This note explores pixart-xl-2 in depth, examining its features, performance, and why it is particularly well-suited for fast visual prototyping. We will cover its technical specifications, compare it with other models, discuss use cases, and provide practical guidance for implementation.
Technical Details of pixart-xl-2
pixart-xl-2 is a diffusion-transformer-based text-to-image generative model, specifically the PixArt-XL-2-1024-MS variant, designed to produce high-resolution 1024x1024 pixel images from text prompts in a single sampling process. It leverages a fixed, pretrained T5 text encoder and a VAE latent feature encoder, similar to other transformer latent diffusion models like stable-diffusion-xl-refiner-1.0.
Model Size and Training Efficiency
With 0.6 billion parameters, it was trained on 0.025 billion images, taking 675 A100 GPU days compared to 6,250 days for Stable Diffusion v1.5. This translates to a training cost of $26,000 versus $320,000, a 90% reduction in CO2 emissions, and just 1% of the training cost of RAPHAEL.
Inference Speed
The standard version uses 14 sampling steps, taking approximately 16 seconds on a T4 GPU (Colab free tier), 5.5 seconds on V100 (32 GB), and 2.2 seconds on A100 (80 GB). The LCM (Latent Consistency Models) variant, PixArt-LCM-XL-2-1024-MS, reduces this to 4 steps, achieving 3.3 seconds on T4, 0.8 seconds on V100, and 0.51 seconds on A100, with batch size 1.
Fast Sampling Methods
The model supports fast sampling methods, making it highly efficient for rapid prototyping. This is particularly useful for designers and developers who need quick iterations and feedback on visual concepts without significant delays.
Use Cases and Practical Applications
pixart-xl-2 is ideal for industries requiring rapid visual prototyping, such as advertising, game design, and product development. Its ability to generate high-quality images quickly allows teams to test ideas and gather feedback without the need for extensive manual work.
Conclusion & Next Steps
pixart-xl-2 represents a significant advancement in text-to-image generation, offering a balance of speed, efficiency, and quality. For those looking to integrate this technology into their workflow, the next steps involve experimenting with the model, exploring its capabilities, and adapting it to specific project needs.
- Experiment with different text prompts to explore the model's range
- Compare output quality and speed with other models like Stable Diffusion
- Integrate the model into existing design workflows for rapid prototyping
art-xl-2 supports SA-Solver, a stochastic Adams method for fast diffusion sampling, integrated into the model for improved efficiency. This training-free sampler enhances performance, especially for few-step sampling, achieving state-of-the-art FID scores on benchmark datasets.
The model is intended for research in generative models, artworks, design, educational tools, and safe deployment, with limitations including challenges in photorealism, rendering legible text, and compositionality (e.g., 'A red cube on top of a blue sphere'), as well as potential social biases.
Suitability for Fast Visual Prototyping
Fast visual prototyping demands speed, ease of use, cost-effectiveness, flexibility, and quality. pixart-xl-2 addresses these needs effectively. Its inference times, particularly with the LCM variant at 3.3 seconds on T4, enable rapid image generation, crucial for iterative design processes.
Speed and Efficiency
The model's speed aligns with the 'prompt-to-pixel in seconds' promise, facilitating quick feedback loops. This is particularly useful for designers and artists who need to iterate rapidly on their ideas without long waiting times.
Ease of Use and Accessibility
By accepting natural language text prompts, it democratizes design, allowing users without advanced skills to generate visuals. This is supported by free inference platforms like Hugging Face and Google Colab notebooks, enhancing accessibility for a wider audience.
Cost-Effectiveness and Sustainability
The model's efficient training and low inference requirements reduce costs compared to traditional methods like hiring artists or using resource-heavy models. Its environmental benefits, with 90% lower CO2 emissions, add to its appeal for sustainable practices.
Flexibility and Quality
Users can modify prompts to explore variations, supporting rapid experimentation. For example, changing a prompt from 'a futuristic cityscape' to 'a futuristic cityscape at night' can generate new visuals instantly. Despite its efficiency, pixart-xl-2 delivers competitive image quality, rated comparably or better than other leading models.
Conclusion & Next Steps
Final thoughts and a summary of the key points of the blog post. The model's combination of speed, ease of use, cost-effectiveness, and quality makes it a strong candidate for fast visual prototyping. Future improvements could focus on enhancing photorealism and text rendering capabilities.
- Speed: 3.3 seconds inference time on T4
- Ease of Use: Natural language prompts
- Cost-Effectiveness: Lower resource requirements
- Quality: Competitive with leading models
PixArt-XL-2 is a cutting-edge text-to-image diffusion model that stands out for its efficiency and high-quality outputs. It leverages advanced techniques to generate images from textual descriptions, making it a powerful tool for various applications. The model is particularly noted for its ability to produce detailed and visually appealing images with minimal computational resources.
Key Features of PixArt-XL-2
PixArt-XL-2 introduces several innovative features that set it apart from other models. One of its standout capabilities is the integration with SA-Solver, which significantly speeds up the generation process without compromising quality. Additionally, the model supports few-step sampling, making it highly efficient for rapid prototyping and iterative design processes.
Efficiency and Speed
The model's efficiency is one of its most notable attributes. With as few as 4 sampling steps, PixArt-XL-2 can produce high-quality images, drastically reducing the time required for generation. This makes it an ideal choice for applications where speed is crucial, such as real-time design and content creation.
Comparative Analysis
When compared to other leading text-to-image models, PixArt-XL-2 demonstrates superior performance in terms of both speed and cost-effectiveness. For instance, it achieves comparable user preference ratings to models like DALL-E 2 and Stable Diffusion, but with significantly lower training costs. This makes it a more accessible option for a wide range of users.
Practical Use Cases
PixArt-XL-2's versatility allows it to be used across various domains. In product design, it enables rapid generation of mockups for stakeholder feedback. Marketing teams can leverage it to create compelling visuals for campaigns, while artists can explore new creative possibilities with its advanced capabilities.
Product Design
Designers can use PixArt-XL-2 to quickly generate and iterate on product concepts. For example, a simple prompt like 'a sleek smartphone with a curved screen' can yield multiple design variations, facilitating faster decision-making and prototyping.
Conclusion & Next Steps
PixArt-XL-2 represents a significant advancement in text-to-image generation, combining efficiency, quality, and affordability. Its ability to produce high-quality images with minimal computational resources makes it a valuable tool for a wide range of applications. Future developments could further enhance its capabilities, making it even more versatile and user-friendly.
- Efficient image generation with few-step sampling
- High-quality outputs comparable to leading models
- Cost-effective training and deployment
PixArt-XL-2 is a cutting-edge text-to-image diffusion model that excels in generating high-quality images from textual prompts. It offers two variants: a standard model for high-quality outputs and a Latent Consistency Model (LCM) variant for rapid inference. The model is designed to be efficient, cost-effective, and environmentally friendly, making it a versatile tool for various creative and professional applications.
Key Features of PixArt-XL-2
PixArt-XL-2 stands out due to its ability to generate 1024px resolution images with minimal computational resources. The standard model focuses on delivering high-quality visuals, while the LCM variant reduces the number of inference steps to just 4-8, significantly speeding up the process. This makes it ideal for rapid prototyping and iterative design workflows. Additionally, the model supports detailed prompts, allowing users to specify intricate details like textures, lighting, and styles.
Standard vs. LCM Variants
The standard variant of PixArt-XL-2 is optimized for quality, producing detailed and visually appealing images. On the other hand, the LCM variant is tailored for speed, enabling quick iterations and real-time feedback. Both variants leverage advanced diffusion techniques to ensure that the generated images meet high aesthetic and technical standards.
Applications of PixArt-XL-2
PixArt-XL-2 is widely used in industries such as marketing, design, education, and game development. Marketers can create compelling visuals for campaigns, while designers can explore different styles and concepts quickly. Educators benefit from visual aids that enhance learning, and game developers can prototype assets efficiently. The model's flexibility and speed make it a valuable tool for any creative or technical project.
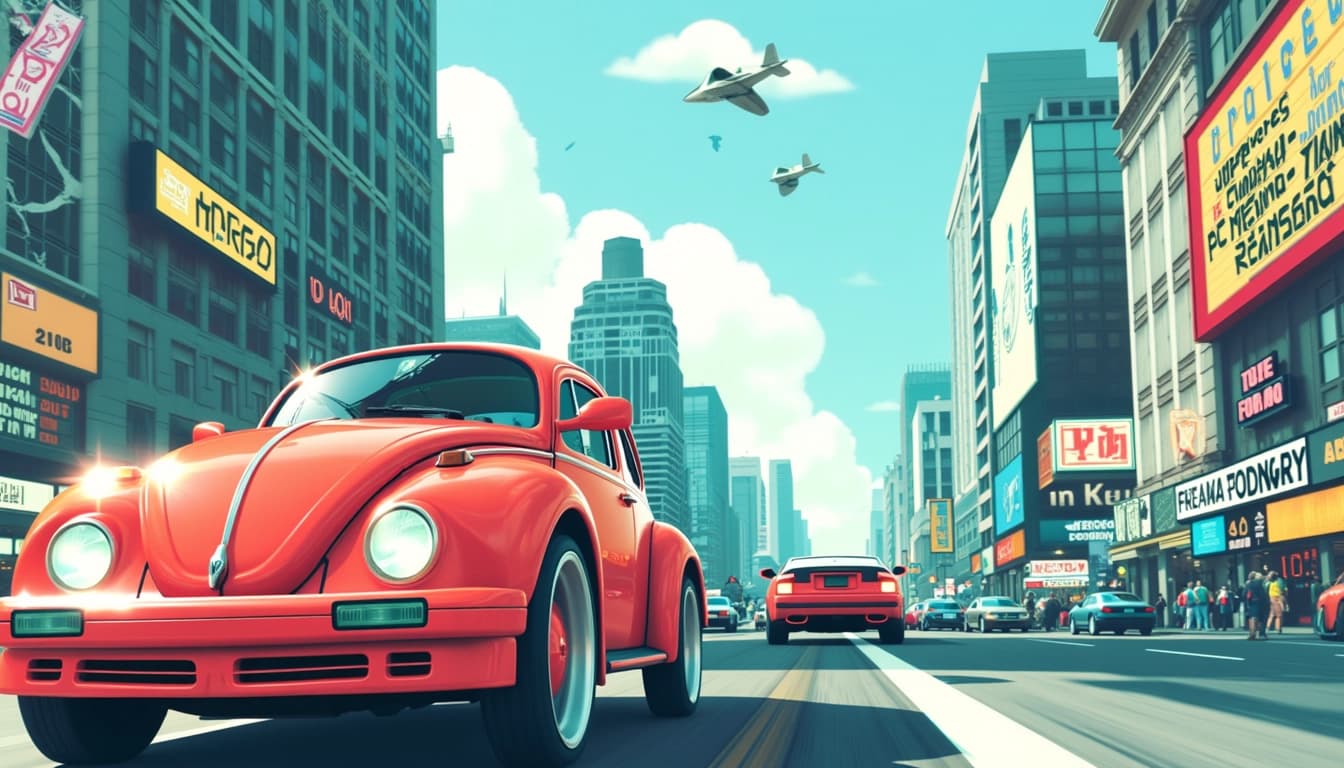
Getting Started with PixArt-XL-2
To start using PixArt-XL-2, users can access the model via Hugging Face or Google Colab. The Hugging Face platform offers free inference, while Colab provides a notebook for easy experimentation. For local use, the GitHub repository includes installation instructions and dependencies. The model requires diffusers, transformers, and other libraries, ensuring compatibility with most development environments.
Conclusion & Next Steps
PixArt-XL-2 is a powerful tool for generating high-quality images quickly and efficiently. Its standard and LCM variants cater to different needs, from detailed visuals to rapid prototyping. By leveraging platforms like Hugging Face and GitHub, users can easily integrate it into their workflows. Whether you're a designer, marketer, educator, or developer, PixArt-XL-2 offers the tools to enhance your creative projects.
- Access PixArt-XL-2 on Hugging Face for free inference.
- Use Google Colab notebooks for quick experimentation.
- Install locally via GitHub for advanced customization.
PixArt-α is a cutting-edge text-to-image diffusion model that offers high-quality image generation with remarkable efficiency. It is designed to transform textual descriptions into visually stunning images, leveraging advanced AI techniques to achieve superior results.
Key Features of PixArt-α
PixArt-α stands out due to its efficiency and high-quality output. The model is optimized to generate images quickly while maintaining exceptional detail and fidelity. It is particularly useful for applications requiring rapid prototyping or creative exploration.
Efficiency and Speed
One of the standout features of PixArt-α is its ability to generate images in significantly less time compared to other models. This makes it ideal for real-time applications or scenarios where speed is a critical factor.
Applications of PixArt-α
PixArt-α can be used in a variety of fields, including digital art, marketing, and entertainment. Its ability to generate high-quality images from text descriptions opens up endless possibilities for creative professionals and businesses alike.
Getting Started with PixArt-α
To start using PixArt-α, you can explore the Hugging Face space or use the provided Google Colab notebook. These resources offer a hands-on introduction to the model, allowing you to experiment with its capabilities and integrate it into your projects.
Hugging Face Space
The Hugging Face space provides an interactive environment where you can test PixArt-α without any setup. Simply input your text prompt and see the model generate images in real-time.
Conclusion & Next Steps
PixArt-α represents a significant advancement in text-to-image generation, combining efficiency with high-quality output. Whether you're an artist, developer, or business professional, this model offers powerful tools to bring your ideas to life.
- Explore the Hugging Face space for interactive demos.
- Use the Google Colab notebook for deeper integration.
- Experiment with different text prompts to see the model's versatility.